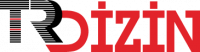
Lösemi hastalığının temel türlerinden ALL ve KML malignitelerinin grafik sinir ağları ve bulanık mantık algoritması ile sınıflandırılması
Yıl: 2023 Cilt: 38 Sayı: 2 Sayfa Aralığı: 707 - 720 Metin Dili: Türkçe DOI: 10.17341/gazimmfd.1022624 İndeks Tarihi: 13-03-2023
Lösemi hastalığının temel türlerinden ALL ve KML malignitelerinin grafik sinir ağları ve bulanık mantık algoritması ile sınıflandırılması
Öz: Beyaz kan hücresi kanseri olan lösemi, yaşam kalitesini düşüren ve ilerleyen aşamalarda ölüme sebep olabilen maliyeti yüksek bir malignitedir. Farklı yaş gruplarında görülebilen bu hastalığın erken ve doğru teşhisinin sağlanması tedavi sürecini etkilemekte ve hastalığın ilerlemesini engellemektedir. Bu çalışmada löseminin temel türlerinden olan ALL ve KML malignitelerinin sınıflandırılması amaçlanmıştır. Genetik temelli maligniteler olan bu türlerin ayırt edilmesinde DNA’da bir mutasyon sonucunda beliren BCR-ABL geni analiz edilmiştir. Tıp dünyasında BCR-ABL geni üzerinden mevcut türlerin ayırt edilmesi hususunda PCR tekniği kullanılarak değerlendirmeler yapılabilmektedir. Teşhis ve tedavi sürecindeki maliyetin ve zamanın indirgenmesi amacıyla disiplinlerarası çalışmalar da mevcuttur . İki aşamadan oluşan bu çalışmanın ilk aşamasında farklı nükleotit uzunluklarına sahip ALL ve KML DNA dizilimlerinin spektral yoğunluk bilgisi sinyal işleme teknikleri kullanılarak spektogramlara yansıtılmıştır. Ardından CNN ve GNN teknolojilerinin hibrit yaklaşımı olan DGCNN teknolojisi ile ALL ve KML malignitelerine ait spektogramlar sınıflandırılmıştır. Ancak nükleotitlerin farklı uzunluklarda olmasından dolayı spektogramlar üzerinde net ifadeler elde edilememiştir. Çalışmanın ikinci aşamasında farklı uzunluklara sahip DNA dizilimleri, kodon dağılımlarının esas alındığı entropi temelli haritalama tekniği ile sayısallaştırılmıştır. Sayısallaştırılan bu dizilimler üzerinden çıkarılan istatiktiksel ve yapısal özellikler uyarlanabilir bulanık mantık algoritması ile sınıflandırılarak nükleotit uzunluğundan bağımsız bir çalışma gerçekleştirilmiştir. Böylece KML ve ALL malignitelerinin sınıflandırılmasında %80’lik bir başarı düzeyi yakalanmıştır.
Anahtar Kelime: Classification of ALL and CML malignancies being among the main types of leukaemia with graph neural networks and fuzzy logic algorithm
Öz: Leukaemia, a white blood cell cancer, is a costly malignancy that reduces the quality of life and can lead to death in the later stages. Early and accurate diagnosis of this disease, which can be seen in different age groups, affects the treatment process and prevents the progression of the disease. This study aims to classify ALL and CML malignancies, which are the main types of leukaemia. The BCR-ABL gene, which appears due to a mutation in the DNA, is analyzed to distinguish these types, which are genetically based malignancies. In the medical world, evaluations can be made using the PCR technique to distinguish the existing species over the BCR-ABL gene. There are also interdisciplinary studies to reduce the cost and time in the diagnosis and treatment process. In the first stage of this two-stage study, the spectral density information of ALL and CML DNA sequences with different nucleotide lengths is reflected on the spectrograms using signal processing techniques. Then, the spectrograms of ALL and CML malignancies are classified with DGCNN technology, which is a hybrid approach of CNN and GNN technologies. However, due to the different lengths of the nucleotides, clear expressions on the spectrograms could not be obtained. In the second stage of the study, DNA sequences with different lengths are digitized with the entropy-based mapping technique based on codon distributions. Statistical and structural features extracted from these digitized sequences are classified by the adaptive fuzzy logic algorithm, and a study independent of nucleotide length is performed. Thus, a maximum 80% success level is achieved in the classification of CML and ALL malignancies.
Anahtar Kelime: Belge Türü: Makale Makale Türü: Araştırma Makalesi Erişim Türü: Erişime Açık
- Aydın G., Quercetinin KML kök hücreleri üzerine sitotoksik etkilerinin moleküler düzeyde incelenmesi, Yüksek Lisans Tezi, Erciyes Üniversitesi, Sağlık Bilimleri Enstitüsü, Kayseri, 2017.
- Healthline. A guide to leukemia. https://www.healthline.com/health/leukemia#treatment. 2021.
- Arslan S., KML ve ALL tanılı hastalarda BCR / ABL füzyon geni mutasyonlarının taranması, Yüksek Lisans Tezi, Eskişehir Osmangazi Üniversitesi, Sağlık Bilimleri Enstitüsü, Eskişehir, 2014.
- Kitamura H. et al., A new highly sensitive real-time quantitative-PCR method for detection of BCR-ABL1 to monitor minimal residual disease in chronic myeloid leukemia after discontinuation of imatinib, PLoS One, 14 (3), 1–13, 2019.
- MedlinePlus. BCR-ABL genetic test. https://medlineplus.gov/lab- tests/bcr-abl-genetic-test/. 2021.
- Paiva A.S. et al., Detection of the BCR-ABL Gene By the Real-Time PCR Method in Patients with Chronic Myeloid Leukemia in Rio Grande Do Norte, Brazil, Blood, 132 (1), 2018.
- Uzoma I., Nna E., Detection and quantitation of bcr-abl1 fusion gene in saliva of chronic myeloid leukaemic patients in nigeria, Proceedings - 2017 IEEE International Conference on Bioinformatics and Biomedicine, BIBM 2017, 2017.
- Smitalova D., Dvorakova D., Racil Z., Romzova M., Digital PCR can provide improved BCR-ABL1 detection in chronic myeloid leukemia patients in deep molecular response and sensitivity of standard quantitative methods using EAC assays, Practical Laboratory Medicine, 25, 2021.
- Yang R., Paparini A., Monis P., Ryan U., Comparison of next- generation droplet digital PCR (ddPCR) with quantitative PCR (qPCR) for enumeration of Cryptosporidium oocysts in faecal samples, International Journal for Parasitology., 44 (14), 1105–1113, 2014.
- Maier J., Lange T., Cross M., Wildenberger K., Niederwieser D., and Franke G.N., Optimized Digital Droplet PCR for BCR-ABL, Journal of Molecular Diagnostics,21 (1), 27–37, 2019.
- Jennings L.J., George D., Czech J., Yu M., and Joseph L., Detection and quantification of BCR-ABL1 fusion transcripts by droplet digital PCR, Journal of Molecular Diagnostics, 16 (2), 174–179, 2014.
- Khodaei A., Feizi-Derakhshi M.R., and Mozaffari-Tazehkand B., A pattern recognition model to distinguish cancerous DNA sequences via signal processing methods, Soft Computing, 24 (21), 16315–16334, 2020.
- Chakraborty S. and Gupta V., DWT based cancer identification using EIIP, Proceedings - 2016 2nd International Conference on Computational Intelligence and Communication Technology, CICT 2016, 718–723, 2016.
- Liu D.W. et al., Automated detection of cancerous genomic sequences using genomic signal processing and machine learning, Future Generation Computer Systems, 98, 233–237, 2019.
- Das J. and Barman S., Bayesian Fusion in Cancer Gene Prediction, International Journal of Computer Application (0975 – 8887) International Conference on Computing, Communication and Sensor Network (CCSN 2014), 5–10, 2014.
- Wang B., Mohl J., Leung M. Y., Computational Prediction of Functional Effects for Cancer Related Genetic Sequence Variants, Proceedings - 2020 IEEE International Conference on Bioinformatics and Biomedicine, BIBM 2020, 2999–3001, 2020.
- Sawyer E., Banuelos M., Marcia R. F., and Sindi S., A Neural Network Approach for Anomaly Detection in Genomic Signals, 2020 Asia- Pacific Signal and Information Processing Association Annual Summit and Conference, APSIPA ASC 2020 - Proceedings, 968–971, 2020.
- Saini S. and Dewan L., Application of discrete wavelet transform for analysis of genomic sequences of Mycobacterium tuberculosis, SpringerPlus, 5 (1), 1–15, 2016.
- Gayathri T. T., Analysis of Genomic sequences for prediction of Cancerous cells using Wavelet technique, International Research Journal of Engineering and Technology(IRJET), 4 (4), 1071–1077, 2017.
- Zhao Y. et al., Uncovering the prognostic gene signatures for the improvement of risk stratification in cancers by using deep learning algorithm coupled with wavelet transform, BMC Bioinformatics, 21 (1),1–24, 2020.
- Ghosh A. and Barman S., Prediction of Prostate Cancer Cells based on Principal Component Analysis Technique, Procedia Technology, 10, 37–44, 2013.
- Muflikhah L., Widodo N., Mahmudy W.F, Solimun, Prediction of Liver Cancer Based on DNA Sequence Using Ensemble Method, 2020 3rd International Seminar on Research of Information Technology and Intelligent Systems, ISRITI 2020, 37–41, 2020.
- Das J., Barman S., DSP based entropy estimation for identification and classification of Homo sapiens cancer genes, Microsystem Technologies, 23 (9), 4145–4154, 2017.
- Yu N., Li Z., Yu Z., Survey on encoding schemes for genomic data representation and feature learning-from signal processing to machine learning, Big Data Mining and Analytics, 1 (3), 191–210, 2018.
- Das B., DNA dizilimlerinden hastalık tanılanması için işaret işleme temelli yeni yaklaşımların geliştirilmesi, Doktora Tezi, Fırat Üniversitesi, Fen Bilimleri Üniversitesi,Elazığ, 2018.
- Abo-Zahhad M., Ahmed S.M., Abd-Elrahman S.A., Genomic Analysis and Classification of Exon and Intron Sequences Using DNA Numerical Mapping Techniques, International Journal of Information Technology and Computer Science, 4 (8), 22–36, 2012.
- Das B., Turkoglu I. A novel numerical mapping method based on entropy for digitizing DNA sequences, Neural Comput. Appl., 29 (8), 207–215, 2018.
- Ahmadi H.R., Mahdavi N., Bayat M., A novel damage identification method based on short time Fourier transform and a new efficient index, Structures, 33, 3605–3614, 2021.
- Fidan H., Dalgacık dönüşümü tekniği ile motor arıza tespiti, Yüksek Lisans Tezi, Süleyman Demirel Üniversitesi, Fen Bilimleri Üniversitesi, Isparta 2006.
- Avci K., Coskun O., Spectral performance analysis of cosh window based new two parameter hybrid windows, 26th IEEE Signal Processing and Communications Applications Conference, SIU 2018, 1–4, 2018.
- Xia Y., Johnson B.K., Jiang Y., Fischer N., Xia H., A new method based on artificial neural network, Wavelet Transform and Short Time Fourier Transform for Subsynchronous Resonance detection, International Journal of Electrical Power and Energy Systems, 103, 377–383, 2018.
- Valizadeh M., Sohrabi M., Ameri Braki Z., Rashidi R., and Pezeshkpur M., Investigation of spectrophotometric simultaneous absorption of Salmeterol and Fluticasone in Seroflo spray by continuous wavelet transform and radial basis function neural network methods, Spectrochimica Acta - Part A: Molecular and Biomolecular Spectroscopy, 263, 2021.
- Volkan Öner İ., Yeşilyurt K., Yilmaz E.Ç., Wavelet Analı̇z Teknı̇ğı̇ Ve Uygulama Alanları, Ordu Üniv. Bil. Tek. Derg, 7 (1), 42–56, 2017.
- National Center for Biotechnology Information. Nucleotide database. https://www.ncbi.nlm.nih.gov. 2021.
- Zhang M., Cui Z., Neumann M., Chen Y., An end-to-end deep learning architecture for graph classification, 32nd AAAI Conference on Artificial Intelligence, AAAI 2018, 4438–4445, 2018.
- Wu Z., Pan S., Chen F., Long G., Zhang C., Yu P.S., A Comprehensive Survey on Graph Neural Networks, IEEE Transactions on Neural Networks and Learning Systems., 32 (1), 4–24, 2019.
- Wang S. H., Govindaraj V.V., Górriz J. M., Zhang X., Zhang Y. D., Covid-19 classification by FGCNet with deep feature fusion from graph convolutional network and convolutional neural network, Information Fusion, 67, 208–229, 2021.
- Boyaci A.Ç., Solmaz M.B., Kabak M., A model proposal for occupational health and safety risk assessment based on multi-criteria hesitant fuzzy linguistic term sets: An application in plastics industry, Journal of the Faculty of Engineering and Architecture of Gazi University, 36 (2), 1041–1053, 2021.
- [39] Öztürk M., Paksoy T., An new interval type-2 hybrid fuzzy rule- based AHP system for supplier selection, Journal of the Faculty of Engineering and Architecture of Gazi University, 35 (3), 1519–1535, 2020.
- Raza K., Fuzzy logic based approaches for gene regulatory network inference, Artificial Intelligence in Medicine, 97, 189–203, 2019.
- Thakur N., Juneja M., Classification of glaucoma using hybrid features with machine learning approaches, Biomedical Signal Processing and Control, 62, 2020.
- Matlab. İstatistik komutları. https://www.mathworks.com/help/. 2021.
- Wikipedia. Group method of data handling. https://en.wikipedia.org/wiki/Group_method_of_data_handling. 2021.
- Ivakhnenko A.G., Polynomial theory of complex systems. IEEE transactıons on systems, man, and cybernetıcs, SMC-1 (4), 364–378, 1971.
- Mahdevari S.,Khodabakhshi M.B, A hybrid PSO-ANFIS model for predicting unstable zones in underground roadways, Tunnelling and Underground Space Technology, 117, 2021.
- Das B., Türkoglu I., Classification of DNA sequences using numerical mapping techniques and Fourier transformation, Journal of the Faculty of Engineering and Architecture of Gazi University, 31 (4), 921–932, 2016.
- Wang X., Gotoh O., Cancer classification using single genes., International Conference on Genome Informatics, 23 (1), 179–188, 2009.
- Ghorai S., Mukherjee A., Dutta P. K., Gene Expression Data Classification by VVRKFA, Procedia Technology, 4, 330–335, 2012.
- Maulik U., Chakraborty D., Fuzzy preference based feature selection and semisupervised SVM for cancer classification, IEEE Transactions on Nanobioscience, 13 (2), 152–160, 2014.
- Begum S., Sarkar R., Chakraborty D., Sen S., Maulik U., Application of active learning in DNA microarray data for cancerous gene identification, Expert Systems with Applications, 177, 2021.
- Ocampo-Vega R., Sanchez-Ante G., De Luna M.A., Vega R., Falcón- Morales L.E., Sossa H., Improving pattern classification of DNA microarray data by using PCA and Logistic Regression, Intelligent Data Analysis., 20, 2016.
- Chen Y., Zhao Y., A novel ensemble of classifiers for microarray data classification, Applied Soft Computing Journal, 8 (4), 1664–1669, 2008.
- Wang X., Simon R., Microarray-based cancer prediction using single genes, BMC Bioinformatics, 12, 2011.
- Khorshed T., Moustafa M.N., Rafea A., Learning Visualizing Genomic Signatures of Cancer Tumors using Deep Neural Networks, Proceedings of the International Joint Conference on Neural Networks, 2020.
APA | Akalın F, Yumusak N (2023). Lösemi hastalığının temel türlerinden ALL ve KML malignitelerinin grafik sinir ağları ve bulanık mantık algoritması ile sınıflandırılması. , 707 - 720. 10.17341/gazimmfd.1022624 |
Chicago | Akalın Fatma,Yumusak Nejat Lösemi hastalığının temel türlerinden ALL ve KML malignitelerinin grafik sinir ağları ve bulanık mantık algoritması ile sınıflandırılması. (2023): 707 - 720. 10.17341/gazimmfd.1022624 |
MLA | Akalın Fatma,Yumusak Nejat Lösemi hastalığının temel türlerinden ALL ve KML malignitelerinin grafik sinir ağları ve bulanık mantık algoritması ile sınıflandırılması. , 2023, ss.707 - 720. 10.17341/gazimmfd.1022624 |
AMA | Akalın F,Yumusak N Lösemi hastalığının temel türlerinden ALL ve KML malignitelerinin grafik sinir ağları ve bulanık mantık algoritması ile sınıflandırılması. . 2023; 707 - 720. 10.17341/gazimmfd.1022624 |
Vancouver | Akalın F,Yumusak N Lösemi hastalığının temel türlerinden ALL ve KML malignitelerinin grafik sinir ağları ve bulanık mantık algoritması ile sınıflandırılması. . 2023; 707 - 720. 10.17341/gazimmfd.1022624 |
IEEE | Akalın F,Yumusak N "Lösemi hastalığının temel türlerinden ALL ve KML malignitelerinin grafik sinir ağları ve bulanık mantık algoritması ile sınıflandırılması." , ss.707 - 720, 2023. 10.17341/gazimmfd.1022624 |
ISNAD | Akalın, Fatma - Yumusak, Nejat. "Lösemi hastalığının temel türlerinden ALL ve KML malignitelerinin grafik sinir ağları ve bulanık mantık algoritması ile sınıflandırılması". (2023), 707-720. https://doi.org/10.17341/gazimmfd.1022624 |
APA | Akalın F, Yumusak N (2023). Lösemi hastalığının temel türlerinden ALL ve KML malignitelerinin grafik sinir ağları ve bulanık mantık algoritması ile sınıflandırılması. Gazi Üniversitesi Mühendislik Mimarlık Fakültesi Dergisi, 38(2), 707 - 720. 10.17341/gazimmfd.1022624 |
Chicago | Akalın Fatma,Yumusak Nejat Lösemi hastalığının temel türlerinden ALL ve KML malignitelerinin grafik sinir ağları ve bulanık mantık algoritması ile sınıflandırılması. Gazi Üniversitesi Mühendislik Mimarlık Fakültesi Dergisi 38, no.2 (2023): 707 - 720. 10.17341/gazimmfd.1022624 |
MLA | Akalın Fatma,Yumusak Nejat Lösemi hastalığının temel türlerinden ALL ve KML malignitelerinin grafik sinir ağları ve bulanık mantık algoritması ile sınıflandırılması. Gazi Üniversitesi Mühendislik Mimarlık Fakültesi Dergisi, vol.38, no.2, 2023, ss.707 - 720. 10.17341/gazimmfd.1022624 |
AMA | Akalın F,Yumusak N Lösemi hastalığının temel türlerinden ALL ve KML malignitelerinin grafik sinir ağları ve bulanık mantık algoritması ile sınıflandırılması. Gazi Üniversitesi Mühendislik Mimarlık Fakültesi Dergisi. 2023; 38(2): 707 - 720. 10.17341/gazimmfd.1022624 |
Vancouver | Akalın F,Yumusak N Lösemi hastalığının temel türlerinden ALL ve KML malignitelerinin grafik sinir ağları ve bulanık mantık algoritması ile sınıflandırılması. Gazi Üniversitesi Mühendislik Mimarlık Fakültesi Dergisi. 2023; 38(2): 707 - 720. 10.17341/gazimmfd.1022624 |
IEEE | Akalın F,Yumusak N "Lösemi hastalığının temel türlerinden ALL ve KML malignitelerinin grafik sinir ağları ve bulanık mantık algoritması ile sınıflandırılması." Gazi Üniversitesi Mühendislik Mimarlık Fakültesi Dergisi, 38, ss.707 - 720, 2023. 10.17341/gazimmfd.1022624 |
ISNAD | Akalın, Fatma - Yumusak, Nejat. "Lösemi hastalığının temel türlerinden ALL ve KML malignitelerinin grafik sinir ağları ve bulanık mantık algoritması ile sınıflandırılması". Gazi Üniversitesi Mühendislik Mimarlık Fakültesi Dergisi 38/2 (2023), 707-720. https://doi.org/10.17341/gazimmfd.1022624 |