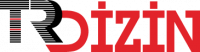
Derin öğrenme temelli hibrid altın endeksi (XAU/USD) yön tahmin modeli
Yıl: 2023 Cilt: 38 Sayı: 2 Sayfa Aralığı: 1117 - 1128 Metin Dili: Türkçe DOI: 10.17341/gazimmfd.888456 İndeks Tarihi: 13-03-2023
Derin öğrenme temelli hibrid altın endeksi (XAU/USD) yön tahmin modeli
Öz: Borsa, döviz kuru, kripto para yön tahminlerinin yanı sıra 1 ons altının dolar cinsinden değerini belirleyen altın endeksinin (XAU/USD) yönünün tahminlenmesi de yatırımcılar, araştırmacılar ve analistler için cezbedici bir araştırma konusu olmuştur. Bu çalışma kapsamında, derin öğrenme metodolojisi temelli altın endeksinin yönünü tahmin eden bir modelin oluşturulması amaçlanmıştır. Önerilen model, altın endeksine ait gerçek zamanlı verilerle metin içerikli verilerin harmanlanması sonucu elde edilmiş olup hem bu yönüyle hem de yön tahmininde kullanılan hibrid derin öğrenme yöntemleri açısından hibrid bir tahmin modeli özelliği de taşımaktadır. Bildiğimiz kadarıyla bu çalışma, sosyal medya platformunu finansal duygu analizi amacıyla kaynak olarak kullanan ve bunu sayısal verilerle harmanlayarak altın endeksi için derin öğrenme temelli yön tahmin modeli oluşturan literatürdeki ilk çalışma niteliğindedir. Çalışmanın literatüre katkısı dört aşamada özetlenebilir: İlk aşamada, finansal duygu analizini gerçekleştirebilmek için Twitter ortamından toplanan verileri ayrıştırma, kelimelerin sözlükteki doğru hallerini bulma, kelimelerin köklerini bulma, kelimeleri normalize etme, kullanılmayan karakterleri ve kelimeleri temizleme gibi yöntemlerle veriler temizlenip modellenmeye hazır hale getirilmiştir. Modellemeye hazır olan veri CNN, RNN, LSTM, Word2Vec, Glove, fastText, BERT, M-BERT, DistilBERT, ELMo, ULMFiT, RoBERTa, ELECTRA, GPT-2 yöntemleri kullanılarak sınıflandırılmıştır. İkinci olarak, XAU/USD gerçek zamanlı verileriyle finansal duygu analizinden elde edilen verilerin sonuçları harmanlanmıştır. Üçüncü olarak, MLP, CNN, LSTM, CNN-LSTM, ConvLSTM derin öğrenme modelleri ile XAU/USD yön tahmin modeli inşa edilmiştir. Dördüncü olarak, birbirinden farklı yapıda olan beş tahmin modelinden alınan sonuçların XAU/USD yönünün tahminindeki performansı sunulmuştur. Sonuç olarak, önerilen modelin performansı literatür çalışmalarıyla kıyaslandığında yüksek doğrulukla kayda değer ölçüde üstünlük göstermektedir.
Anahtar Kelime: Deep learning based hybrid gold index (XAU/USD) direction forecast model
Öz: Forecasting the direction of the gold index, which determines the dollar value of 1 ounce of gold, is an attractive research topic for investors, researchers and analysts. In this study, it is aimed to construct a model that predicts the direction of the gold index based on deep learning. The proposed model is obtained as a result of blending the numerical dataset of the gold index with the textual data. This is a hybrid prediction model both in this aspect and in terms of hybrid deep learning methods used in direction prediction. As far as we know, this is the first attempt in the literature that uses the social media platform as a source for financial sentiment analysis and constructs a deep learning-based direction prediction model for the gold index by blending it with numerical data. The contribution of the study to the literature is summarized in four stages: In the first stage, in order to carry out the sentiment analysis, the dataset is cleaned and ready for modeling by methods such as parsing the dataset collected from the Twitter environment, finding the correct forms of the words in the dictionary, finding the roots of the words, normalizing the words, and cleaning the unused characters. Afterwards, the dataset is classified using 14 different deep learning-based methods. Secondly, the results of the sentiment analysis are blended with the numerical data of XAU/USD. Third, the XAU/USD direction prediction model is constructed with deep learning models. Fourth, the performance of the results from five different forecasting models in predicting the direction of XAU/USD is presented. As a result, the performance of the proposed model is significantly superior with high accuracy when compared to the state-of-the-art studies.
Anahtar Kelime: Belge Türü: Makale Makale Türü: Araştırma Makalesi Erişim Türü: Erişime Açık
- Sohangir S., Wang D., Pomeranets A., Khoshgoftaar T.M., Big Data: Deep learning for financial sentiment analysis, J Big Data 5 (1), 1-25, 2018.
- Day M., Lee C., Deep learning for financial sentiment analysis on finance news providers, IEEE/ACM International Conference on Advances in Social Networks Analysis and Mining, San Francisco, California, 1127-1134, 18-21 Ağustos, 2016.
- Bollen J., Mao H., Zeng X., Twitter mood predicts the stock market, Journal of Computational Science, 2 (1), 1-8, 2011.
- Smailović J., Grčar M., Lavrač N., Žnidaršič M., Stream-based active learning for sentiment analysis in the financial domain, Information Sciences, 285, 181-203, 2014.
- Bing L., Chan K.C.C., Ou C., Public sentiment analysis in twitter data for prediction of a company's stock price movements, IEEE 11th International Conference on E-Business Engineering, Guangzhou, China, 232-239, 5-7 Kasım, 2014.
- Kilimci Z.H., Duvar R., An efficient word embedding and deep learning based model to forecast the direction of stock exchange market using twitter and financial news sites: A case of Istanbul stock exchange (BIST 100), IEEE Access, 8, 188186-188198, 2020.
- Kilimci Z.H., Financial sentiment analysis with deep ensemble models (DEMs) for stock market prediction, Journal of the Faculty of Engineering and Architecture of Gazi University, 35 (2), 635-650, 2019.
- Yasar H., Kilimci Z.H., US Dollar/Turkish Lira exchange rate forecasting model based on deep learning methodologies and time series analysis, Symmetry, 12 (9), 1553-1571, 2020.
- Kilimci Z.H., Sentiment analysis based direction prediction in bitcoin using deep learning algorithms and word embedding models, International Journal of Intelligent Systems and Applications in Engineering, 8 (2), 60-65, 2020.
- Yakut E., Elmas B., Yavuz S., Yapay sinir ağları ve destek vektör makineleri yöntemleriyle borsa endeksi tahmini, Süleyman Demirel Üniversitesi İktisadi İdari Bilimler Fakültesi Dergisi, 19 (1), 139-157, 2014.
- Kutlu B., Badur B., Yapay sinir ağları ile borsa endeksi tahmini, Yönetim Dergisi, 20 (63), 25-40, 2009.
- Wang J., Sun T., Liu B., Cao Y., Wang D., Financial markets prediction with deep learning, 17th IEEE International Conference on Machine Learning and Applications, Orlando, Florida, 97-104, 17-20 Aralık, 2018.
- Shen S., Jiang H., Zhang T., Stock market forecasting using machine learning algorithms, Proje, Stanford University, Department of Electrical Engineering, Standford, 2012.
- Tsai C. F., Wang S. P., Stock price forecasting by hybrid machine learning techniques, Proceedings of the International Multiconference of Engineers and Computer Scientists, Hong Kong, China, 755-760, 18- 20 Mart, 2009.
- Livieris I. E., Pintelas E., Pintelas P., A CNN–LSTM model for gold price time-series forecasting, Neural Computing and Applications, 32 (23), 17351–17360, 2020.
- Pennington J., Socher R., Manning C.D., Glove: Global vectors for word representation, International Conference on Empirical Methods in Natural Language Processing, Doha-Qatar, 1532-1543, 25-29 Ekim, 2014.
- Mikolov T., Sutskever I., Chen K., Corrado G.S., Dean J., Distributed representations of words and phrases and their compositionality, International Conference on Neural Information Processing Systems, Harrahs and Harveys-Lake, Tahoe, 3111-3119, 5-10 Aralık, 2013.
- Bojanowski P., Grave E., Joulin A., Mikolov T., Enriching word vectors with subword information, Transactions of the Association for Computational Linguistics, 5, 135-146, 2017.
- Lipton Z.C., Berkowitz J., Elkan C.A. Critical review of recurrent neural networks for sequence learning. https://arxiv.org/abs/1506.00019. Ekim 17, 2015. Kasım 11, 2009. Temmuz 25, 2019.
- O'Shea K. ve Nash R. An introduction to convolutional neural networks. https://arxiv.org/abs/1511.08458. Aralık 2, 2015. Temmuz 30, 2019.
- Sak H., Senior A., Beaufays F., Long short-term memory recurrent neural network architectures for large scale acoustic modeling, Annual Conference of the International Speech Communication Association, Singapore, 338-342, 14-18 Eylül, 2014.
- Kelotra A., Pandey P., Stock market prediction using optimized Deep- ConvLSTM model, Big Data, 8 (1), 5-24, 2020.
- Gao Z., Feng A., Song X., Wu X., Target-dependent sentiment classification with BERT, IEEE Access, 7,154290-154299, 2019.
- Rönnqvist S., Kanerva J., Salakoski T., Ginter F., Is multilingual BERT fluent in language generation?, Proceedings of the First NLPL Workshop on Deep Learning for Natural Language Processing, Turku- Finland, 29-36, 30 Eylül, 2019.
- Acheampong F., Nunoo-Mensah H., Wenyu C., Comparative analyses of BERT, RoBERTa, DistilBERT, and XLNet for text-based emotion recognition, 17th International Computer Conference on Wavelet Active Media Technology and Information Processing, Chengdu, China, 117-121, 18 Eylül, 2020.
- Ethayarajh K., How contextual are contextualized word representations? comparing the geometry of BERT, ELMo, and GPT-2 embeddings, Proceedings of the 2019 Conference on Empirical Methods in Natural Language Processing and the 9th International Joint Conference on Natural Language Processing, Hong Kong-China, 55-65, 3-7 Kasım, 2019.
- Howard J., Ruder S., Universal language model fine-tuning for text classification, Proceedings of the 56th Annual Meeting of the Association for Computational Linguistics (Volume 1: Long Papers), Melbourne-Australia, 328–339, 15-20 Temmuz, 2018.
- Clark K., Luong M., Le Q.V., Manning C.D., ELECTRA: Pre-training text encoders as discriminators rather than generators, International Conference on Learning Representations, Addis Ababa, Ethiopia, 1-18, 26 Nisan-1 Mayıs, 2020.
- Gökdemir A., Çalhan A., Deep learning and machine learning based anomaly detection in internet of things environments, Journal of the Faculty of Engineering and Architecture of Gazi University, 37 (4), 1945-1956, 2022.
- Noyan T., Kuncan F., Tekin R., Kaya Y., A new content-free approach to identification of document language: Angle patterns, Journal of the Faculty of Engineering and Architecture of Gazi University, 37 (3), 1277-1292, 2022.
APA | Kantar O, Kilimci Z (2023). Derin öğrenme temelli hibrid altın endeksi (XAU/USD) yön tahmin modeli. , 1117 - 1128. 10.17341/gazimmfd.888456 |
Chicago | Kantar Onur,Kilimci Zeynep Hilal Derin öğrenme temelli hibrid altın endeksi (XAU/USD) yön tahmin modeli. (2023): 1117 - 1128. 10.17341/gazimmfd.888456 |
MLA | Kantar Onur,Kilimci Zeynep Hilal Derin öğrenme temelli hibrid altın endeksi (XAU/USD) yön tahmin modeli. , 2023, ss.1117 - 1128. 10.17341/gazimmfd.888456 |
AMA | Kantar O,Kilimci Z Derin öğrenme temelli hibrid altın endeksi (XAU/USD) yön tahmin modeli. . 2023; 1117 - 1128. 10.17341/gazimmfd.888456 |
Vancouver | Kantar O,Kilimci Z Derin öğrenme temelli hibrid altın endeksi (XAU/USD) yön tahmin modeli. . 2023; 1117 - 1128. 10.17341/gazimmfd.888456 |
IEEE | Kantar O,Kilimci Z "Derin öğrenme temelli hibrid altın endeksi (XAU/USD) yön tahmin modeli." , ss.1117 - 1128, 2023. 10.17341/gazimmfd.888456 |
ISNAD | Kantar, Onur - Kilimci, Zeynep Hilal. "Derin öğrenme temelli hibrid altın endeksi (XAU/USD) yön tahmin modeli". (2023), 1117-1128. https://doi.org/10.17341/gazimmfd.888456 |
APA | Kantar O, Kilimci Z (2023). Derin öğrenme temelli hibrid altın endeksi (XAU/USD) yön tahmin modeli. Gazi Üniversitesi Mühendislik Mimarlık Fakültesi Dergisi, 38(2), 1117 - 1128. 10.17341/gazimmfd.888456 |
Chicago | Kantar Onur,Kilimci Zeynep Hilal Derin öğrenme temelli hibrid altın endeksi (XAU/USD) yön tahmin modeli. Gazi Üniversitesi Mühendislik Mimarlık Fakültesi Dergisi 38, no.2 (2023): 1117 - 1128. 10.17341/gazimmfd.888456 |
MLA | Kantar Onur,Kilimci Zeynep Hilal Derin öğrenme temelli hibrid altın endeksi (XAU/USD) yön tahmin modeli. Gazi Üniversitesi Mühendislik Mimarlık Fakültesi Dergisi, vol.38, no.2, 2023, ss.1117 - 1128. 10.17341/gazimmfd.888456 |
AMA | Kantar O,Kilimci Z Derin öğrenme temelli hibrid altın endeksi (XAU/USD) yön tahmin modeli. Gazi Üniversitesi Mühendislik Mimarlık Fakültesi Dergisi. 2023; 38(2): 1117 - 1128. 10.17341/gazimmfd.888456 |
Vancouver | Kantar O,Kilimci Z Derin öğrenme temelli hibrid altın endeksi (XAU/USD) yön tahmin modeli. Gazi Üniversitesi Mühendislik Mimarlık Fakültesi Dergisi. 2023; 38(2): 1117 - 1128. 10.17341/gazimmfd.888456 |
IEEE | Kantar O,Kilimci Z "Derin öğrenme temelli hibrid altın endeksi (XAU/USD) yön tahmin modeli." Gazi Üniversitesi Mühendislik Mimarlık Fakültesi Dergisi, 38, ss.1117 - 1128, 2023. 10.17341/gazimmfd.888456 |
ISNAD | Kantar, Onur - Kilimci, Zeynep Hilal. "Derin öğrenme temelli hibrid altın endeksi (XAU/USD) yön tahmin modeli". Gazi Üniversitesi Mühendislik Mimarlık Fakültesi Dergisi 38/2 (2023), 1117-1128. https://doi.org/10.17341/gazimmfd.888456 |