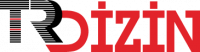
Evrişimli sinir ağı kullanarak çoklu-pozlamalı görüntü birleştirme
Yıl: 2023 Cilt: 38 Sayı: 3 Sayfa Aralığı: 1439 - 1452 Metin Dili: Türkçe DOI: 10.17341/gazimmfd.1067400 İndeks Tarihi: 23-03-2023
Evrişimli sinir ağı kullanarak çoklu-pozlamalı görüntü birleştirme
Öz: Aynı sahneye ait iki ya da daha fazla düşük dinamik alana (LDR) sahip görüntülerden yüksek dinamik alana (HDR) sahip tek bir görüntü elde etme yöntemlerine çoklu-pozlamalı görüntü birleştirme (MEF) denir. Bu çalışmada MEF için derin öğrenme (DL) modellerinden evrişimli sinir ağı (CNN) kullanan yeni bir yöntem önerilmiştir. Önerilen yöntemde ilk adımda CNN modeli kullanılarak kaynak görüntülerden birleştirme haritası (fmap) elde edilmiştir. Birleştirilmiş görüntülerde testere-dişi etkisini ortadan kaldırmak için fmap üzerinde ağırlıklandırma işlemi gerçekleştirilmiştir. Daha sonra ağırlıklandırılmış fmap kullanılarak her tarafı iyi pozlanmış birleştirilmiş görüntüler oluşturulmuştur. Önerilen yöntem literatürde yaygın olarak kullanılan MEF veri setlerine uygulanmış ve elde edilen birleştirilmiş görüntüler kalite metrikleri kullanılarak değerlendirilmiştir. Önerilen yöntem ve diğer iyi bilinen görüntü birleştirme yöntemleri, görsel ve niceliksel değerlendirme açısından karşılaştırılmıştır. Elde edilen sonuçlar, geliştirilen tekniğin uygulanabilirliğini göstermektedir.
Anahtar Kelime: Multi-exposure image fusion using convolutional neural network
Öz: The method of obtaining a single high dynamic range (HDR) image from two or more low dynamic range (LDR) images of the same scene is called multi-exposure image fusion (MEF). In this study, a new MEF method using convolutional neural network (CNN) from deep learning (DL) models is proposed. In the proposed method, firstly, the fusion map (fmap) was computed from source LDR images using the CNN model. In order to eliminate the saw-tooth effect in the fused images, weighting was performed on the fmap. Then, a well-exposed fused image was constructed using the weighted fmap. After that, the proposed method was applied to the MEF datasets, which are widely used in the literature, and the fused images obtained were evaluated using well-known quality metrics. The developed technique and other well-known MEF techniques are compared in terms of quantitative and visual evaluation. The results obtained show the feasibility of the proposed technique.
Anahtar Kelime: Belge Türü: Makale Makale Türü: Araştırma Makalesi Erişim Türü: Erişime Açık
- 1. Kaur H., Koundal D., Kadyan V., Image fusion techniques: A Survey, Arch Computat Methods Eng, 28, 4425–4447, 2021.
- 2. Karishma C.B., Bhumika S., A review of image fusion techniques, 2018 Second International Conference on Computing Methodologies and Communication (ICCMC), 2018.
- 3. Ma J., Ma Y., Li C., Infrared and visible image fusion methods and applications: A survey, Information Fusion, 45, 153-178, 2019.
- 4. Aslantas V., Bendes E., Kurban R., Toprak, A.N., New optimised region- based multi-scale image fusion method for thermal and visible images, IET Image Processing, 289-299, 2014.
- 5. Maruthi R., Lakshmi I., Multi-focus image fusion methods – A Survey, IOSR Journal of Computer Engineering (IOSR-JCE), 19 (4), 9-25, 2017.
- 6. Aslantas V., Kurban R., Fusion of multi-focus images using differential evolution algorithm, Expert Systems with Applications, 8861–8870, 2010.
- 7. Aslantaş V., Kurban R., A comparison of criterion functions for fusion of multi-focus noisy images, Optics Communications, 16 (282), 3231– 3242, 2009.
- 8. Aslantas V., Bendes E., A new image quality metric for image fusion: The sum of the correlations of differences, Int. J. Electron. Commun., 69, 1890-1896, 2015.
- 9. Wei H., Zhongliang J., Evaluation of focus measures in multi-focus image fusion, Pattern Recognition Letters, 28 (4), 493-500, 2007.
- 10. Ke P., Jung C., Fang Y., Perceptual multi-exposure image fusion with overall image quality index and local saturation, Multimedia Systems, 23 (2), 239–250, 2017.
- 11. Singh S., Mittal N., Singh H., Review of various image fusion algorithms and image fusion performance metric, Archives of Computational Methods in Engineering, 28 (5), 3645–3659, 2021.
- 12. Zhao S., Wang Y., A Novel patch-based multi-exposure image fusion using super-pixel segmentation, IEEE Access, 8, 39034-39045, 2020.
- 13. Yadong X., Beibei S., Color-compensated multi-scale exposure fusion based on physical features, Optik, 223, 2020.
- 14. Bavirisetti D.P., Dhuli R., Multi-focus image fusion using multi-scale image decomposition and saliency detection, Ain Shams Engineering Journal, 9, 1103–1117, 2018.
- 15. Zhang X., Benchmarking and comparing multi-exposure image fusion algorithms, Information Fusion, 74, 111-131, 2021.
- 16. Mertens T., Kautz J., Reeth F.V., Exposure Fusion, 15th Pacific Conference on Computer Graphics and Applications, USA, 2007.
- 17. Malik M.H., Gilani S.A.M., Anwaar-ul-Haq, Wavelet based exposure fusion, Proceedings of the World Congress on Engineering, London, 2008.
- 18. Wang J., Xu D., Lang C., Li B., Exposure fusion based on shift-invariant discrete wavelet transform, Journal Of Information Science And Engineering, 27, 197-211, 2011.
- 19. Martorell O., Sbert C., Buades A., Ghosting-free DCT based multi- exposure image fusion, Signal Processing: Image Communication, 78, 409-425, 2019.
- 20. Kou F., Zhengguo L., Changyun W., Weihai C., Edge-preserving smoothing pyramid based multi-scale exposure fusion, J. Vis. Commun. Image Represent, 53, 235–244, 2018.
- 21. Hayat N., Imran M., Ghost-free multi exposure image fusion technique using dense SIFT descriptor and guided filter, Journal of Visual Communication and Image Representation, 62, 295-308, 2019.
- 22. Qiegen L., Leung H., Variable augmented neural network for decolorization and multi-exposure fusion, Information Fusion, 46, 114- 127, 2019.
- 23. Song M., Tao D., Chen C., Bu J., Luo J., Zhang C., Probabilistic exposure fusion, IEEE Trans. Image Process, 21 (1), 341-357, 2012.
- 24. Gu B., Li W., Wong J., Zhu M., Wang M., Gradient field multi-exposure images fusion for high dynamic range image visualization, J. Vis. Commun. Image Represent, 23 (4), 604-610, 2012.
- 25. Li S., Kang X., Fast multi-exposure image fusion with median filter and recursive filter, IEEE Trans. Consum. Electron, 58 (2), 626-632, 2012.
- 26. Bo G., Wujing L., Jiangtao W., Minyun Z., Minghui W., Gradient field multi-exposure images fusion for high dynamic range image visualization, Journal of Visual Communication and Image Representation, 23 (4), 604-610, 2012.
- 27. Zhang W., Cham W., Gradient-directed multiexposure composition, IEEE Transactions on Image Processing, 21 (4), 2318-2323, 2012.
- 28. Sujoy P., Ioana S.S., Panajotis A., Multi-exposure and multi-focus image fusion in gradient domain, Journal of Circuits, Systems and Computers, 25 (10), 1650123, 2016.
- 29. Goshtasby A.A., Fusion of multi-exposure images, Image and Vision Computing, 23 (6), 611–618, 2005.
- 30. Kong J., Wang R., Lu Y., Feng X., Zhang J., A Novel fusion approach of multi-exposure image, EUROCON 2007 The International Conference on “Computer as a Tool”, Warsaw-Poland, 2007.
- 31. Kede M., Hui L., Hongwei Y., Zhou W., Deyu M., Lei Z., Robust multi- exposure image fusion: A structural patch decomposition approach, IEEE Trans. Image Processing, 26 (5), 2519–2532, 2017.
- 32. Zhang W., Hu S., Liu K., Patch-based correlation for deghosting in exposure fusion, Information Sciences, 415–416, 19-27, 2017.
- 33. Zhang W., Hu S., Liu K., Yao J., Motion-free exposure fusion based on inter-consistency and intra-consistency, Information Sciences, 376, 190- 201, 2017.
- 34. Ma K., Duanmu Z., Zhu H., Fang Y., Wang Z., Deep guided learning for fast multi-exposure image fusion, IEEE Transactions on Image Processing, 29, 2808-2819, 2020.
- 35. Xu H., Ma J., Zhang X., MEF-GAN: Multi-exposure image fusion via generative adversarial networks, IEEE Transactions on Image Processing, 29, 7203-7216, 2020.
- 36. Prabhakar K.R., Srikar V.S., Babu R.V., A Deep unsupervised approach for exposure fusion with extreme exposure image pairs, International Conference on Computer Vision (ICCV), 2017.
- 37. Qi Y., Zhou S., Zhang Z., Luo S., Lin X., Wang L., Qiang B., Deep unsupervised learning based on color un-referenced loss functions for multi-exposure image fusion, Information Fusion, 66, 18-39, 2021.
- 38. Somuncu E., Aydın Atasoy N., Realization of character recognition application on text images by convolutional neural network, Journal of the Faculty of Engineering and Architecture of Gazi University, 37 (1), 17-28, 2021.
- 39. Elmas B., Identifying species of trees through bark images by convolutional neural networks with transfer learning method, Journal of the Faculty of Engineering and Architecture of Gazi University, 36 (3), 1253-1270, 2021.
- 40. Elmas B., Classification varieties of marble and granite by convolutional neural networks with transfer learning method, Journal of the Faculty of Engineering and Architecture of Gazi University, 37 (2), 985-1002, 2002.
- 41. Narin A., İşler Y., Detection of new coronavirus disease from chest x-ray images using pre-trained convolutional neural networks. Journal of the Faculty of Engineering and Architecture of Gazi University, 36 (4), 2095-2108, 2021.
- 42. Romanuke V.V., An infinitely scalable dataset of single-polygon grayscale images as a fast test platform for semantic image segmentation, KPI Science News, 1, 24-34, 2019.
- 43. Alzubaidi L., Zhang J., Humaidi A.J., Al-Dujaili A., Duan Y., Review of deep learning: concepts, CNN architectures, challenges, applications, future directions, Journal of Big Data, 8 (53), 2021.
- 44. Krizhevsky A., Sutskever I., Hinton G.E., Imagenet classification with deep convolutional neural networks, Advances in Neural Information Processing Systems, 1097–1105, 2012.
- 45. Simonyan K., Zisserman A., Very deep convolutional networks for large- scale image recognition, 3rd International Conference on Learning Representations (ICLR), San Diego-CA-USA, 2015.
- 46. Szegedy C., Liu W., Jia Y., Sermanet P., Reed S., Anguelov D., Erhan D., Vanhoucke V., Rabinovich A., Going deeper with convolutions, 2015 IEEE Conference on Computer Vision and Pattern Recognition (CVPR), 2015.
- 47. He K., Zhang X., Ren S., Sun J., Deep residual learning for image recognition, IEEE Conference on Computer Vision and Pattern Recognition, 2016.
- 48. Badrinarayanan V., Kendall A., Cipolla R., SegNet: A deep convolutional encoder-decoder architecture for image segmentation, IEEE Transactions on Pattern Analysis and Machine Intelligence, 39 (12), 2481-2495, 2017.
- 49. Minaee S., Boykov Y.Y., Porikli F., Plaza, A.J., Kehtarnavaz N., Terzopoulos D., Image segmentation using deep learning: A Survey, IEEE Transactions on Pattern Analysis and Machine Intelligence, 2021.
- 50. Paoletti M.E., Haut J.M., Plaza J., Plaza A., Deep learning classifiers for hyperspectral imaging: A review, ISPRS Journal of Photogrammetry and Remote Sensing, 158, 279-317, 2019.
- 51. Ma K., Zeng K., Wang Z., Perceptual quality assessment for multi- exposure image fusion, IEEE Transactions on Image Processing, 24 (11), 3345-3356, 2015.
- 52. Jagalingam P., Hegde A.V., A review of quality metrics for fused image, Aquatic Procedia, 133-142, 2015.
- 53. Nayar S.K., Nakagawa Y., Shape from focus, IEEE Transactions on Pattern Analysis and Machine Intelligence, 16 (8), 824–831, 1994.
- 54. Chen Y., Blum, R.S., A new automated quality assessment algorithm for image fusion, Image and Vision Computing, 1421-1432, 2009.
- 55. Wang Z., Bovik A.C., Sheikh H.R., Simoncelli E.P., Image quality assessment: from error visibility to structural similarity, IEEE Transactions on Image Processing, 13 (4), 600-612, 2004.
- 56. Hasan M., Sohel F., Diepeveen D., Laga H., Jones M.G.K., A survey of deep learning techniques for weed detection from images, Computers and Electronics in Agriculture, 184, 2021.
- 57. Li H., Zhang L., Multi-exposure fusion with CNN features, 25th IEEE International Conference on Image Processing (ICIP), Athens-Greece, 2018.
- 58. Li H., Ma K., Yong H., Zhang L., Fast multi-scale structural patch decomposition for multi-exposure image fusion, IEEE Trans. Image Process, 29, 5805–5816, 2020.
APA | AKBULUT H, aslantaş v (2023). Evrişimli sinir ağı kullanarak çoklu-pozlamalı görüntü birleştirme. , 1439 - 1452. 10.17341/gazimmfd.1067400 |
Chicago | AKBULUT HARUN,aslantaş veysel Evrişimli sinir ağı kullanarak çoklu-pozlamalı görüntü birleştirme. (2023): 1439 - 1452. 10.17341/gazimmfd.1067400 |
MLA | AKBULUT HARUN,aslantaş veysel Evrişimli sinir ağı kullanarak çoklu-pozlamalı görüntü birleştirme. , 2023, ss.1439 - 1452. 10.17341/gazimmfd.1067400 |
AMA | AKBULUT H,aslantaş v Evrişimli sinir ağı kullanarak çoklu-pozlamalı görüntü birleştirme. . 2023; 1439 - 1452. 10.17341/gazimmfd.1067400 |
Vancouver | AKBULUT H,aslantaş v Evrişimli sinir ağı kullanarak çoklu-pozlamalı görüntü birleştirme. . 2023; 1439 - 1452. 10.17341/gazimmfd.1067400 |
IEEE | AKBULUT H,aslantaş v "Evrişimli sinir ağı kullanarak çoklu-pozlamalı görüntü birleştirme." , ss.1439 - 1452, 2023. 10.17341/gazimmfd.1067400 |
ISNAD | AKBULUT, HARUN - aslantaş, veysel. "Evrişimli sinir ağı kullanarak çoklu-pozlamalı görüntü birleştirme". (2023), 1439-1452. https://doi.org/10.17341/gazimmfd.1067400 |
APA | AKBULUT H, aslantaş v (2023). Evrişimli sinir ağı kullanarak çoklu-pozlamalı görüntü birleştirme. Gazi Üniversitesi Mühendislik Mimarlık Fakültesi Dergisi, 38(3), 1439 - 1452. 10.17341/gazimmfd.1067400 |
Chicago | AKBULUT HARUN,aslantaş veysel Evrişimli sinir ağı kullanarak çoklu-pozlamalı görüntü birleştirme. Gazi Üniversitesi Mühendislik Mimarlık Fakültesi Dergisi 38, no.3 (2023): 1439 - 1452. 10.17341/gazimmfd.1067400 |
MLA | AKBULUT HARUN,aslantaş veysel Evrişimli sinir ağı kullanarak çoklu-pozlamalı görüntü birleştirme. Gazi Üniversitesi Mühendislik Mimarlık Fakültesi Dergisi, vol.38, no.3, 2023, ss.1439 - 1452. 10.17341/gazimmfd.1067400 |
AMA | AKBULUT H,aslantaş v Evrişimli sinir ağı kullanarak çoklu-pozlamalı görüntü birleştirme. Gazi Üniversitesi Mühendislik Mimarlık Fakültesi Dergisi. 2023; 38(3): 1439 - 1452. 10.17341/gazimmfd.1067400 |
Vancouver | AKBULUT H,aslantaş v Evrişimli sinir ağı kullanarak çoklu-pozlamalı görüntü birleştirme. Gazi Üniversitesi Mühendislik Mimarlık Fakültesi Dergisi. 2023; 38(3): 1439 - 1452. 10.17341/gazimmfd.1067400 |
IEEE | AKBULUT H,aslantaş v "Evrişimli sinir ağı kullanarak çoklu-pozlamalı görüntü birleştirme." Gazi Üniversitesi Mühendislik Mimarlık Fakültesi Dergisi, 38, ss.1439 - 1452, 2023. 10.17341/gazimmfd.1067400 |
ISNAD | AKBULUT, HARUN - aslantaş, veysel. "Evrişimli sinir ağı kullanarak çoklu-pozlamalı görüntü birleştirme". Gazi Üniversitesi Mühendislik Mimarlık Fakültesi Dergisi 38/3 (2023), 1439-1452. https://doi.org/10.17341/gazimmfd.1067400 |