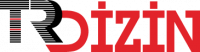
Evrişimli sinir ağları ile ağaç kabuğu görüntülerinden ağaç türlerinin transfer öğrenme yöntemiyle tanımlanması
Yıl: 2021 Cilt: 36 Sayı: 3 Sayfa Aralığı: 1253 - 1269 Metin Dili: Türkçe DOI: 10.17341/gazimmfd.689038 İndeks Tarihi: 10-11-2022
Evrişimli sinir ağları ile ağaç kabuğu görüntülerinden ağaç türlerinin transfer öğrenme yöntemiyle tanımlanması
Öz: Derin Öğrenme yöntemiyle ağaç kabuğu görüntülerinden ağaçların tanımlanması, ağaçların geleceğe taşınmasında, yaşamsal açısından önemli ağaçların koruma altına alınmasında, orman kaynaklarının sürdürülebilir yönetim planlarının yapılmasında, botanik konusunda deneyimi olmayan kişilerin doğayı araştırmasında, meyvecilik sektöründe farklı ya da aynı sınıfın alt türlerinin belirlenmesinde, kereste/mobilya/kâğıt vb. sektörlerdeki ağaç kesim operasyonları ile ağaçların işlenme süreçlerinde, ağaçların doğru ve verimli kullanılmasında yararlı olabilecek bir problem çözümüdür. Fakat Derin Öğrenmedeki son gelişmeler etkileyici sonuçlar ortaya koymasına rağmen, veri kümesi eksikliği veya yetersizliği ağaç kabuğu türlerinin tanımlanmasında derin öğrenme kullanımını kısıtlamıştır. Bu alandaki çalışmalara katkı sağlamak ve ağaç kabuğu görüntülerinden ağaçların tanımlanmasının mümkün olduğunu göstermek amacıyla 59 ağaç türünün 24686 ağaç kabuğu görüntüsü Türkiye’deki çeşitli bölgelerden bir yıllık süreçte toplanarak bir veri seti oluşturulmuştur. Bu veri setiyle yedi adet önceden eğitilmiş evrişimli sinir ağı, AlexNet, DenseNet201, ResNet18, ResNet50, ResNet101, VGG16, VGG19’da transfer öğrenme yöntemi kullanılarak ağaç kabuğu görüntülerinden ağaç türü tanımlamasının mümkün olduğu gösterilmiştir. Bunu yanı sıra transfer öğrenme yönteminin bu tür problemlere hızlı ve başarılı çözümler getireceği gösterilmiş ve ağların derinlik, katman, parametre sayısının ve parti büyüklüğünün (Batch Size) çözüme etkisi incelenmiştir. Çalışmada kullanılan tüm ağların, görüntü sayısı ve eğitim verisi oranına bağlı olarak başarı ortalamaları %93,21 ile %95,89 arasındayken, en başarılı iki ağın başarı ortalaması ise %99,46’dır.
Anahtar Kelime: Identifying species of trees through bark images by convolutional neural networks with transfer learning method
Öz: Identifying trees by images of barks via Deep Learning method has a potentially useful contribution to many areas, such as revision of forests, preparation of sustainable management plans for forest resources, operations and processing of trees for paper and furniture industries, preservation of trees having vital importance to environments, definition of species and sub-species of fruits for orcharding, for amateur purposes, and entirely for handling tree sources efficiently. Even though the current progress in Deep Learning has proven to be impressive, the lack or insufficiency of datasets has limited the use of Deep Learning on identification of tree species from barks images. In order make contribution to the researches on this field, and to prove that tree identification via images of barks with high accuracy is possible, 24686 bark images of 59 tree species from different parts of Turkey has been collected within a span of a year, and the data set is used for this work. With the use of seven pre-trained convolutional neural networks, AlexNet, DenseNet201, ResNet18, ResNet50, ResNet101, VGG16, VGG19. It has been demonstrated that identification of tree species by images of barks is possible through transfer learning method. Additionally, it has been inferred that transfer learning method provides fast and accurate solutions to classification problems. Furthermore, the impact of the depth, layer, number of parameters and batch size of the networks has been analyzed. While the average accuracy of all the networks, regarding the ratio of number of images and training data, is between 93.21% and 95.89%, the average of accuracy of the two most successful networks is 99.46%.
Anahtar Kelime: Belge Türü: Makale Makale Türü: Araştırma Makalesi Erişim Türü: Erişime Açık
- 1. Fiel S. ve Sablatnig R., Automated identification of tree species from Images of the bark, leaves and needles, in Proceedings of 16th Computer Vision Winter Workshop, Mitterberg, Austria, 1-6, 2-4 Şubat, 2011.
- 2. Hellstrom T., Larkeryd P., Nordfjell T., Ringdahl O., Autonomous forest vehicles: Historic, envisioned, and state-of-the-art, International Journal of Forest Engineering, 20 (1), 31-38, 2009.
- 3. He K., Zhang X., Ren S., Sun J., Delving deep into rectifiers: Surpassing human-level performance on ImageNet classification, in Proceedings of the IEEE International Conference on Computer Vision (ICCV), 1026–1034, 2016.
- 4. Beech E., Rivers M., Oldfield S., Smith P. P., GlobalTreeSearch: The first complete global database of tree species and country distributions, Journal of Sustainable Forestry, 36 (5), 454–489, 2017.
- 5. Carpentier M., Giguère P., Gaudreault J., Tree species identification from bark images using convolutional neural networks, 2018 IEEE/RSJ International Conference on Intelligent Robots and Systems (IROS), 1075-1081, 2018.
- 6. Goëau H., Bonnet P., Joly A., Bakic V., Barthélémy D., Boujemaa N., Molino J.F., The imageCLEF 2013 plant identification task. CLEF 2013 Working Notes, Valencia-Spain, hal-00960929, 2013.
- 7. Goëau H., Joly A., Bonnet P., Selmi S., Molino J.F., Barthélémy D., Boujemaa N., LifeCLEF plant identification task 2014. In CLEF2014 Working Notes, Sheffield-UK, 598–615, September 15-18, 2014.
- 8. Lowe D. G., Object recognition from local scale- invariant features, Proceedings of the seventh IEEE international conference on computer vision, 1150– 1157, 1999.
- 9. Bay H., Tuytelaars T., Van Gool L., Surf: Speeded up robust features, In Computer vision–ECCV 2006, 404– 417, Springer, 2006.
- 10. Ojala T., Pietikainen M., Harwood D., A comparative study of texture measures with classification based on featured distributions, Pattern Recognition, 29 (1), 51– 59, 1996.
- 11. Goëau H., Bonnet P., Joly A., LifeCLEF plant identification task 2015, In CLEF2015 Working Notes, Toulouse-France, 2015.
- 12. Šulc M., Mishkin D., Matas J., Very deep residual networks with maxout for plant identification in the wild, In CLEF2016 Working Notes, Portugal, 2016.
- 13. Sunderhauf N., McCool C., Upcroft B., Tristan P., Fine- grained plant classification using convolutional neural networks for feature extraction, In CLEF2014 Working Notes, 756–762, 2014.
- 14. Champ J., Lorieul T., Servajean M., Joly A., A comparative study of fine-grained classification methods in the context of the LifeCLEF plant identification challenge 2015, In CLEF2015 Working Notes, Toulouse-France, 2015.
- 15. Goëau H., Bonnet P., Joly A., Plant identification based on noisy web data: the amazing performance of deep learning, In CLEF2015 Working Notes, Dublin-Ireland, 2017.
- 16. Huang Z.K., Quan Z.H., Du J.X., Bark classification based on contourlet filter features using RBPNN, In Intelligent Computing, 1121–1126, 2006.
- 17. Fiel S. ve Sablatnig R., Automated identification of tree species from images of the bark, leaves and needles, 16th Computer Vision Winter Workshop, Mitterberg- Austria, 67–74, 2011.
- 18. Sulc M. ve Matas J., Kernel-mapped histograms of multi-scale LBPs for tree bark recognition, 28th International Conference of Image and Vision Computing New Zealand (IVCNZ), Wellington-New Zealand, 82–87, 2013.
- 19. Boudra S., Yahiaoui I., Behloul A., A comparison of multi-scale local binary pattern variants for bark image retrieval, 16th International Conference on Advanced Concepts for Intelligent Vision Systems (ACIVS), Catania-Italy, 764–775, 2015.
- 20. Bressane A., Roveda J. A. F., Martins A. C. G., Statistical analysis of texture in trunk images for biometric identification of tree species, Environmental Monitoring and Assessment, 187 (212), 2015.
- 21. Othmani A. A., Jiang C., Lomenie N., Favreau J. M., Piboule A., Voon L. F. C. L. Y., A novel computer-aided tree species identification method based on burst wind segmentation of 3D bark textures, Machine Vision and Applications, 27 (5), 751–766, 2016.
- 22. Mizoguchi T., Ishii A., Nakamura H., Inoue T., Takamatsu H., Lidar-based individual tree species classification using convolutional neural network, SPIE Optocal Metrology 2017 Videometrics Range Imaging and Applications, 10332, 2017.
- 23. Carpentier M., Giguère P., Gaudreault J., Tree species identification from bark images using convolutional neural networks, IEEE/RSJ International Conference on Intelligent Robots and Systems (IROS), 2018.
- 24. Arı A., Hanbay D., Tumor detection in MR images of regional convolutional neural networks, Journal of the Faculty of Engineering and Architecture of Gazi University, 34 (3), 1395-1408, 2019.
- 25. Dandıl E., Polattimur R., Dog Behavior Recognition and Tracking based on Faster R-CNN, Journal of the Faculty of Engineering and Architecture of Gazi University, 35 (2), 819-834, 2020.
- 26. Yıldız O., Melanoma detection from dermoscopy images with deep learning methods: A comprehensive study, Journal of the Faculty of Engineering and Architecture of Gazi University, 34 (4), 2241-2260, 2019.
- 27. Bingöl K., Akan A. E., Örmecioğlu H. T., Er A., Artificial intelligence applications in earthquake resistant architectural design: Determination of irregular structural systems with deep learning and ImageAI method, Journal of the Faculty of Engineering and Architecture of Gazi University, 35 (4), 2197-2209, 2020.
- 28. Aktaş A., Doğan B., Demir Ö., Tactile paving surface detection with deep learning methods, Journal of the Faculty of Engineering and Architecture of Gazi University, 35 (3), 1685-1700, 2020.
- 29. Lawrence S., Giles C. L., Tsoi A. C., Back A. D., Face recognition: A convolutional neural-network approach, IEEE Transactions on Neural Networks, 8 (1), 98-113, 1997.
- 30. https://www.mathworks.com/help/deeplearning/ug/intr oduction-to-convolutional-neural-networks.html, Yayın tarihi, 2019. Erişim tarihi Kasım 26, 2019.
- 31. Baykal E., Dogan H., Ercin M. E., Ersoz S. , Ekinci M., Transfer learning with pre-trained deep convolutional neural networks for serous cell classification, Multimedia Tools and Applications, 1-19, 2019.
- 32. Zhou Y., Nejati H., Do T. T., Cheung N. M., Cheah L., Image-based vehicle analysis using deep neural network: A systematic study, IEEE international conference on digital signal processing, 276-280, 2016.
- 33. Krizhevsky A., Sutskever I., Hinton G. E., ImageNet classification with deep convolutional neural networks, NIPS'12: Proceedings of the 25th International Conference on Neural Information Processing Systems, Nevada, 1097–1105, 2012.
- 34. Huang G., Liu Z., Maaten L. V. D., Weinberger K. Q., Densely connected convolutional networks, IEEE Conference on Computer Vision and Pattern Recognition, Honolulu, 4700-4708, 2018.
- 35. He K., Zhang X., Ren S., Sun J., Deep Residual Learning for Image Recognition, IEEE Conference on Computer Vision and Pattern Recognition, Las Vegas, 770-778, 2016.
- 36. Theckedath D., Sedamkar R. R., Detecting Affect States Using VGG16, ResNet50 and SE ResNet50 Networks, Springer Nature Computer Science, 79, 1-7, 2020.
- 37. Ye H., Han H., Zhu L., Duan Q., Vegetable pest image recognition method based on improved VGG convolution neural network, Journal of Physics: Conference Series, 1237 (3), 2019.
- 38. Pan S. J., Yang Q., A survey on transfer learning, IEEE Transactions on Knowledge and Data Engineering, 22 (10), 1345-1359, 2010.
- 39. https://machinelearningmastery.com/transfer-learning- for-deep-learning/, Erişim tarihi Ekim 12, 2019
- 40. Chi Z., Houqiang L., Chao W., Plant species recognition based on bark patterns using novel Gabor filter banks, International Conference on Neural Networks and Signal Processing, Nanjing, 1035–1038, 2003.
- 41. Blaanco L. J., Travieso C. M., Quinteiro J. M., Hernandez P. V., Dutta M. K., Singh A., A bark recognition algorithm for plant classification using a least square support vector machine, in 2016 Ninth International Conference on Contemporary Computing (IC3), Noida, 1–5, 2016.
APA | Elmas B (2021). Evrişimli sinir ağları ile ağaç kabuğu görüntülerinden ağaç türlerinin transfer öğrenme yöntemiyle tanımlanması. , 1253 - 1269. 10.17341/gazimmfd.689038 |
Chicago | Elmas Bahadır Evrişimli sinir ağları ile ağaç kabuğu görüntülerinden ağaç türlerinin transfer öğrenme yöntemiyle tanımlanması. (2021): 1253 - 1269. 10.17341/gazimmfd.689038 |
MLA | Elmas Bahadır Evrişimli sinir ağları ile ağaç kabuğu görüntülerinden ağaç türlerinin transfer öğrenme yöntemiyle tanımlanması. , 2021, ss.1253 - 1269. 10.17341/gazimmfd.689038 |
AMA | Elmas B Evrişimli sinir ağları ile ağaç kabuğu görüntülerinden ağaç türlerinin transfer öğrenme yöntemiyle tanımlanması. . 2021; 1253 - 1269. 10.17341/gazimmfd.689038 |
Vancouver | Elmas B Evrişimli sinir ağları ile ağaç kabuğu görüntülerinden ağaç türlerinin transfer öğrenme yöntemiyle tanımlanması. . 2021; 1253 - 1269. 10.17341/gazimmfd.689038 |
IEEE | Elmas B "Evrişimli sinir ağları ile ağaç kabuğu görüntülerinden ağaç türlerinin transfer öğrenme yöntemiyle tanımlanması." , ss.1253 - 1269, 2021. 10.17341/gazimmfd.689038 |
ISNAD | Elmas, Bahadır. "Evrişimli sinir ağları ile ağaç kabuğu görüntülerinden ağaç türlerinin transfer öğrenme yöntemiyle tanımlanması". (2021), 1253-1269. https://doi.org/10.17341/gazimmfd.689038 |
APA | Elmas B (2021). Evrişimli sinir ağları ile ağaç kabuğu görüntülerinden ağaç türlerinin transfer öğrenme yöntemiyle tanımlanması. Gazi Üniversitesi Mühendislik Mimarlık Fakültesi Dergisi, 36(3), 1253 - 1269. 10.17341/gazimmfd.689038 |
Chicago | Elmas Bahadır Evrişimli sinir ağları ile ağaç kabuğu görüntülerinden ağaç türlerinin transfer öğrenme yöntemiyle tanımlanması. Gazi Üniversitesi Mühendislik Mimarlık Fakültesi Dergisi 36, no.3 (2021): 1253 - 1269. 10.17341/gazimmfd.689038 |
MLA | Elmas Bahadır Evrişimli sinir ağları ile ağaç kabuğu görüntülerinden ağaç türlerinin transfer öğrenme yöntemiyle tanımlanması. Gazi Üniversitesi Mühendislik Mimarlık Fakültesi Dergisi, vol.36, no.3, 2021, ss.1253 - 1269. 10.17341/gazimmfd.689038 |
AMA | Elmas B Evrişimli sinir ağları ile ağaç kabuğu görüntülerinden ağaç türlerinin transfer öğrenme yöntemiyle tanımlanması. Gazi Üniversitesi Mühendislik Mimarlık Fakültesi Dergisi. 2021; 36(3): 1253 - 1269. 10.17341/gazimmfd.689038 |
Vancouver | Elmas B Evrişimli sinir ağları ile ağaç kabuğu görüntülerinden ağaç türlerinin transfer öğrenme yöntemiyle tanımlanması. Gazi Üniversitesi Mühendislik Mimarlık Fakültesi Dergisi. 2021; 36(3): 1253 - 1269. 10.17341/gazimmfd.689038 |
IEEE | Elmas B "Evrişimli sinir ağları ile ağaç kabuğu görüntülerinden ağaç türlerinin transfer öğrenme yöntemiyle tanımlanması." Gazi Üniversitesi Mühendislik Mimarlık Fakültesi Dergisi, 36, ss.1253 - 1269, 2021. 10.17341/gazimmfd.689038 |
ISNAD | Elmas, Bahadır. "Evrişimli sinir ağları ile ağaç kabuğu görüntülerinden ağaç türlerinin transfer öğrenme yöntemiyle tanımlanması". Gazi Üniversitesi Mühendislik Mimarlık Fakültesi Dergisi 36/3 (2021), 1253-1269. https://doi.org/10.17341/gazimmfd.689038 |