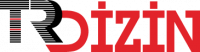
Derin öğrenme yöntemleri ile dokunsal parke yüzeyi tespiti
Yıl: 2020 Cilt: 35 Sayı: 3 Sayfa Aralığı: 1685 - 1700 Metin Dili: Türkçe DOI: 10.17341/gazimmfd.652101 İndeks Tarihi: 13-01-2021
Derin öğrenme yöntemleri ile dokunsal parke yüzeyi tespiti
Öz: Gerçek zamanlı çalışan sistemlerde görüntü işleme uygulamaları yapmak son yıllarda oldukça popüler birkonu haline gelmiştir. Yapay zekâ alanının alt dallarından biri olan derin öğrenme yöntemleri vegörüntülerden nesne tespiti yapma alanında kullanılan görüntü işleme algoritmaları birliktekullanılabilmektedir. Bu sayede otonom otomobiller, otonom insansız hava araçları, yardımcı robotteknolojileri, engelli ve yaşlı bireyler için asistan teknolojileri gibi birçok alanda uygulamalargeliştirilmektedir. Yapılan çalışmada, görme engelli bireyler, otonom araçlar ve robotlar tarafındankullanılabilecek yardımcı bir teknoloji sistemi tasarlamak için dokunsal parke yüzeylerinin derin öğrenmeyöntemleriyle tespit edilmesi gerçekleştirilmiştir. Geleneksel görüntü işleme algoritmalarının aksine buçalışmada derin öğrenme yöntemleri ile görüntü işleme algoritmaları birlikte kullanılmıştır. Nesne tespitetme yöntemleri içinde en iyi yöntemlerden biri olan You Only Look Once-V3(YOLO-V3) modeli DenseNetmodeli ile birleştirilerek YOLOV3-Dense modeli oluşturulmuştur. YOLO-V2, YOLO-V3 veYOLOV3Dense modelleri tarafımızca oluşturulmuş olan ve içerisinde 4580 etiketli görsel bulunan MarmaraDokunsal Parke Yüzeyi(MDPY) veri seti üzerinde ayrı ayrı eğitildikten sonra performansları test veri setiüzerinde birbirleri ile karşılaştırılmıştır. %89 F1-skor, %92 ortalama hassasiyet ve %81 IoU değerleri ileYOLOV3-Dense modelinin dokunsal parke yüzeyi tespit etmede diğer modellerden daha iyi olduğugözlemlenmiştir.
Anahtar Kelime: Tactile paving surface detection with deep learning methods
Öz: Image processing applications in real-time systems have become a popular topic in recent years. Deep learning methods, one of the sub-branches of artificial intelligence, and image processing algorithms used in the field of object detection from images can be used together. In this way, applications are developed in many areas such as autonomous cars, autonomous unmanned aerial vehicles, assist robot technologies, assistant technologies for disabled and elderly individuals. This study aims to detect the tactile paving surfaces with deep learning methods in order to design an assistive technology system that can be used by visually impaired individuals, autonomous vehicles and robots. Contrary to traditional image processing algorithms, deep learning methods and image processing algorithms are used together in this study. The YOLO-V3 model, which is one of the best methods of object detection, is combined with the DenseNet model to create the YOLOV3-Dense model. YOLO-V2, YOLO-V3 and YOLOV3Dense models were trained on the Marmara Tactile Paving Surface (MDPY) dataset, which was created by the researchers and included 4580 images and their performances were compared with each other on the test dataset. It was observed that YOLOV3-Dense model is better than other models in detecting tactile paving surface with 89% F1-score, 92% mean average Precision(mAP) and 81% IoU values.
Anahtar Kelime: Belge Türü: Makale Makale Türü: Araştırma Makalesi Erişim Türü: Erişime Açık
- 1. World Health Organization, Blindness and vision impairment, https://www.who.int/en/news-room/factsheets/detail/blindness-and-visual-impairment, Yayın tarihi Ekim 8, 2019, Erişim tarihi Kasım 11, 2019.
- 2. Lu, J., Siu, K. W. M., & Xu, P., A comparative study of tactile paving design standards in different countries. 9th International Conference on Computer-Aided Industrial Design and Conceptual Design,China,753-758, 22-25 Nov. 2008.
- 3. Asami, T., & Ohnishi, K., Crosswalk location, direction and pedestrian signal state extraction system for assisting the expedition of person with impaired vision, 2014 10th France-Japan/8th Europe-Asia Congress on Mecatronics (MECATRONICS), Tokyo, 285-290, 27- 29 Nov. 2014
- 4. Ghilardi, M. C., Macedo, R. C. O., & Manssour, I. H., A New Approach for Automatic Detection of Tactile Paving Surfaces in Sidewalks, Procedia Computer Science, 80, 662-672, 2016.
- 5. Mancini, A., Frontoni, E., & Zingaretti, P., Mechatronic System to Help Visually Impaired Users During Walking and Running. IEEE Transactions on Intelligent Transportation Systems (T-ITS) , 19(2), 649-660, 2018.
- 6. Shoval, S., Borenstein, J., & Koren, Y., The NavBelt-a computerized travel aid for the blind based on mobile robotics technology, IEEE Transactions on Biomedical Engineering (TBME), 45(11), 1376-1386, 1998.
- 7. Yang, K., Cheng, R., Wang, K., & Zhao, X., A ground and obstacle detection algorithm for the visually impaired, 2015 IET International Conference on Biomedical Image and Signal Processing (ICBISP 2015), Beijing, China. DOI: 10.1049/cp.2015.0777, 2015.
- 8. Garcia-Garcia, A., Orts, S., Oprea, S., Villena Martinez, V., Martinez-Gonzalez, P., & Rodríguez, J., A Survey on Deep Learning Techniques for Image and Video Semantic Segmentation, Applied Soft Computing, 70, 41-65, 2018.
- 9. Krizhevsky, A., Sutskever, I., & E. Hinton, G., ImageNet Classification with Deep Convolutional Neural Networks, Advances in Neural Information Processing Systems, 25(2), Jan. 2012.
- 10. Deng, J., Dong, W., Socher, R., Li, L. J., Li, K., Li, F. F., & Ieee. ImageNet: A Large-Scale Hierarchical Image Database, IEEE-Computer-Society Conference on Computer Vision and Pattern Recognition Workshops, Miami Beach, FL, 248-255, 20-25 Jun. 2009.
- 11. Russakovsky, O., Deng, J., Su, H., Krause, J., Satheesh, S., Ma, S., Li, F. F., ImageNet Large Scale Visual Recognition Challenge, International Journal of Computer Vision (IJCV), 115, 211-252, 2015.
- 12. He, K., Zhang, X., Ren, S., & Sun, J., Delving deep into rectifiers: Surpassing human-level performance on imagenet classification, Proceedings of the IEEE international conference on computer vision, Massachusetts-USA, 1026-1034, 2015.
- 13. Tan, M., & Le, Q. V. EfficientNet: Rethinking Model Scaling for Convolutional Neural Networks, 2019 International Conference on Machine Learning(ICML), California-USA, 6105-6114, 2019.
- 14. Girshick, R., Donahue, J., Darrell, T., & Malik, J., Rich feature hierarchies for accurate object detection and semantic segmentation. Proceedings of the IEEE conference on computer vision and pattern recognition, Columbus-OH-USA, 580-587, 25 Sept. 2014.
- 15. Liu, W., Anguelov, D., Erhan, D., Szegedy, C., Reed, S., Fu, C.-Y., & Berg, A. C., Ssd: Single shot multibox detector, European conference on computer vision(ECCV2016), Amsterdam-Netherlands, 21-37, 11-14 Oct. 2016.
- 16. Redmon, J., Divvala, S., Girshick, R., & Farhadi, A., You only look once: Unified, real-time object detection, IEEE conference on computer vision and pattern recognition, Las Vegas, NV, USA, 779-788, 27-30 June 2016.
- 17. Sermanet, P., Eigen, D., Zhang, X., Mathieu, M., Fergus, R., & LeCun, Y., Overfeat: Integrated recognition, localization and detection using convolutional networks, International Conference on Learning Representations(ICLR2013), arXiv:1312.6229 2013.
- 18. Einloft, D. C., Ghilardi, M. C., & Manssour, I. H., Automatic Detection of Tactile Paving Surfaces in Indoor Environments, Workshop of Undergraduate Works (WUW) in the 29th Conference on Graphics, Patterns and Images (SIBGRAPI'16), 2016.
- 19. Jie, X., Xiaochi, W., & Zhigang, F., Research and implementation of blind sidewalk detection in portable eta system, International Forum on Information Technology and Applications (IFITA2010), KunmingChina, 431-434, 16-18 July 2010.
- 20. Kassim, A. M., Yasuno, T., Mohd Aras, M. S., hj shukor, A., Jaafar, H. I., Baharom, F., & Jafar, F., Vision based of tactile paving detection method in navigation system for blind person, Jurnal Teknologi, 77, No:20, 11 August 2015.
- 21. Shen, J., Liu, N., Sun, H., Tao, X., & Li, Q., Vehicle Detection in Aerial Images Based on Hyper Feature Map in Deep Convolutional Network, KSII Transactions on Internet & Information Systems, 13(4).No:4, 29 April 2019.
- 22. Ren, S., He, K., Girshick, R., & Sun, J., Faster r-cnn: Towards real-time object detection with region proposal networks, IEEE Transactions on Pattern Analysis and Machine Intelligence (IEEE T PATTERN ANAL), 39,No:6, 4 June 2015.
- 23. Du, Y., Pan, N., Xu, Z., Deng, F., Shen, Y., & Kang, H., Pavement distress detection and classification based on YOLO network, International Journal of Pavement Engineering, doi=10.1080/10298436.2020.1714047, 24 Jan. 2020.
- 24. Wang, J., Wang, N., Li, L., & Ren, Z., Real-time behavior detection and judgment of egg breeders based on YOLO v3, Neural Computing and Applications (NEURAL COMPUT APPL), doi: 10.1007/s00521- 019-04645-4, 2019.
- 25. Kaya, A., Keçeli, A. S., & Can, A. B., Examination of various classification strategies in classification of lung nodule characteristics, Journal of the Faculty of Engineering and Architecture of Gazi University, 34(2), 709-725, 2019.
- 26. Yildiz, O., Melanoma detection from dermoscopy images with deep learning methods: A comprehensive study, Journal of the Faculty of Engineering and Architecture of Gazi University, 34(4), 2241-2260, 2019.
- 27. Dandil, E., & Polattimur, R., Dog Behavior Recognition and Tracking based on Faster R-CNN, Journal of the Faculty of Engineering and Architecture of Gazi University, 35(2), 819-834, 2019.
- 28. Lv, J.-J., Shao, X.-H., Huang, J.-S., Zhou, X.-D., & Zhou, X., Data augmentation for face recognition. Neurocomputing, 230, 184-196, 2017.
- 29. Shorten, C., & Khoshgoftaar, T. M., A survey on Image Data Augmentation for Deep Learning. Journal of Big Data, 6(1), No: 60, (2019).
- 30. Redmon, J., & Farhadi, A., Yolov3: An incremental improvement, arXiv preprint arXiv:1804.02767, 2018.
- 31. Redmon, J., & Farhadi, A., YOLO9000: Better, Faster, Stronger, 2017 IEEE Conference on Computer Vision and Pattern Recognition (CVPR), Hawaii-USA, 6517- 6525, 21-26 July 2017.
- 32. Huang, G., Liu, Z., Maaten, L. v. d., & Weinberger, K. Q., Densely Connected Convolutional Networks. 2017 IEEE Conference on Computer Vision and Pattern Recognition (CVPR), Hawaii-USA, 2261-2269, 21-26 July 2017.
- 33. Goutte, C., & Gaussier, E., A Probabilistic Interpretation of Precision, Recall and F-Score, with Implication for Evaluation, Proceedings of the 27th European conference on Advances in Information Retrieval Research, Spain, 345-359, 2005.
- 34. Everingham, M., Van Gool, L., Williams, C. K., Winn, J., & Zisserman, A., The pascal visual object classes (voc) challenge, International journal of computer vision, 88(2), 303-338, 2010.
APA | aktaş a, Doğan B, Demir O (2020). Derin öğrenme yöntemleri ile dokunsal parke yüzeyi tespiti. , 1685 - 1700. 10.17341/gazimmfd.652101 |
Chicago | aktaş abdulsamet,Doğan Buket,Demir Onder Derin öğrenme yöntemleri ile dokunsal parke yüzeyi tespiti. (2020): 1685 - 1700. 10.17341/gazimmfd.652101 |
MLA | aktaş abdulsamet,Doğan Buket,Demir Onder Derin öğrenme yöntemleri ile dokunsal parke yüzeyi tespiti. , 2020, ss.1685 - 1700. 10.17341/gazimmfd.652101 |
AMA | aktaş a,Doğan B,Demir O Derin öğrenme yöntemleri ile dokunsal parke yüzeyi tespiti. . 2020; 1685 - 1700. 10.17341/gazimmfd.652101 |
Vancouver | aktaş a,Doğan B,Demir O Derin öğrenme yöntemleri ile dokunsal parke yüzeyi tespiti. . 2020; 1685 - 1700. 10.17341/gazimmfd.652101 |
IEEE | aktaş a,Doğan B,Demir O "Derin öğrenme yöntemleri ile dokunsal parke yüzeyi tespiti." , ss.1685 - 1700, 2020. 10.17341/gazimmfd.652101 |
ISNAD | aktaş, abdulsamet vd. "Derin öğrenme yöntemleri ile dokunsal parke yüzeyi tespiti". (2020), 1685-1700. https://doi.org/10.17341/gazimmfd.652101 |
APA | aktaş a, Doğan B, Demir O (2020). Derin öğrenme yöntemleri ile dokunsal parke yüzeyi tespiti. Gazi Üniversitesi Mühendislik Mimarlık Fakültesi Dergisi, 35(3), 1685 - 1700. 10.17341/gazimmfd.652101 |
Chicago | aktaş abdulsamet,Doğan Buket,Demir Onder Derin öğrenme yöntemleri ile dokunsal parke yüzeyi tespiti. Gazi Üniversitesi Mühendislik Mimarlık Fakültesi Dergisi 35, no.3 (2020): 1685 - 1700. 10.17341/gazimmfd.652101 |
MLA | aktaş abdulsamet,Doğan Buket,Demir Onder Derin öğrenme yöntemleri ile dokunsal parke yüzeyi tespiti. Gazi Üniversitesi Mühendislik Mimarlık Fakültesi Dergisi, vol.35, no.3, 2020, ss.1685 - 1700. 10.17341/gazimmfd.652101 |
AMA | aktaş a,Doğan B,Demir O Derin öğrenme yöntemleri ile dokunsal parke yüzeyi tespiti. Gazi Üniversitesi Mühendislik Mimarlık Fakültesi Dergisi. 2020; 35(3): 1685 - 1700. 10.17341/gazimmfd.652101 |
Vancouver | aktaş a,Doğan B,Demir O Derin öğrenme yöntemleri ile dokunsal parke yüzeyi tespiti. Gazi Üniversitesi Mühendislik Mimarlık Fakültesi Dergisi. 2020; 35(3): 1685 - 1700. 10.17341/gazimmfd.652101 |
IEEE | aktaş a,Doğan B,Demir O "Derin öğrenme yöntemleri ile dokunsal parke yüzeyi tespiti." Gazi Üniversitesi Mühendislik Mimarlık Fakültesi Dergisi, 35, ss.1685 - 1700, 2020. 10.17341/gazimmfd.652101 |
ISNAD | aktaş, abdulsamet vd. "Derin öğrenme yöntemleri ile dokunsal parke yüzeyi tespiti". Gazi Üniversitesi Mühendislik Mimarlık Fakültesi Dergisi 35/3 (2020), 1685-1700. https://doi.org/10.17341/gazimmfd.652101 |