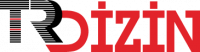
Hiperspektral görüntüler için değiştirilmiş SLIC tabanlı süperpiksel bölütleme
Yıl: 2023 Cilt: 38 Sayı: 1 Sayfa Aralığı: 399 - 408 Metin Dili: Türkçe DOI: 10.17341/gazimmfd.835846 İndeks Tarihi: 23-03-2023
Hiperspektral görüntüler için değiştirilmiş SLIC tabanlı süperpiksel bölütleme
Öz: SLIC (basit doğrusal iteratif kümeleme) süperpiksel algoritması, bölütleme için verimli ve hızlı bir algoritmadır. Bu algoritma doğası gereği üç bantlı renkli görüntüler için tasarlanmıştır. Uzaktan algılamada yeni bir teknoloji olan hiperspektral görüntüleme, zengin spektral ve uzamsal bilgi taşıyan yüzlerce bant içermektedir. Bu çalışmada, SLIC algoritması hiperspektral görüntülerin yapısına göre modifiye edilmiştir. Buna ek olarak, benzer süperpikseller DBSCAN (gürültülü uygulamaların yoğunluk tabanlı uzamsal kümelenmesi) algoritması ile birleştirilmiştir. Esinlenen yeni bir yaklaşımla süperpikseller arasındaki spektral benzerlik indeksi, evrensel görüntü kalitesi indeksine göre hesaplanmıştır. Elde edilen bölütleme haritalarının sınıflandırma performansına katkısı karşılaştırılmalı olarak sunulmuştur. Çalışmada sunulan yaklaşımlarla, Pavia Universitesi veri kümesinin doğruluğu boyut indirgeme yapılarak kullanılan SLIC algoritması ile %86,85’ten %96,66’ya yükseltilmiştir. Indian Pines veri kümesinde, geleneksel SLIC yaklaşımı sınıflandırmaya %89,06 katkı sağlarken, önerilen yaklaşım %97,26 katkı sağlamıştır.
Anahtar Kelime: A modified SLIC-based superpixel segmentation for hyperspectral images
Öz: The SLIC (simple linear iterative clustering) superpixel algorithm is an efficient and fast algorithm for segmentation. The algorithm is inherently designed on three band color images. Hyperspectral imaging, which is a relatively new remote sensing technology, contains hundreds of bands which carry rich spectral and spatial information. In this study, the SLIC algorithm is modified according to the structure of hyperspectral images. In addition to that, the similar superpixels are merged with the DBSCAN (density-based spatial clustering of applications with noise) algorithm. As a novel inspired approach, the spectral similarity index between the superpixels are computed based on the universal image quality index. The contribution of the obtained segmentation maps to the classification performance is presented comparatively. With the approaches presented in the study, the accuracy of the Pavia University dataset was increased from 86.85% to 96.66% using the SLIC algorithm by performing dimension reduction. In the Indian Pines dataset, the traditional SLIC approach contributed 89.06% to the classification, while the proposed approach contributed 97.26%.
Anahtar Kelime: Belge Türü: Makale Makale Türü: Araştırma Makalesi Erişim Türü: Erişime Açık
- 1. J. Ham, Y. Chen, M. M. Crawford, and J. Ghosh, Investigation of the random forest framework for classification of hyperspectral data, IEEE Transaction on Geoscience and Remote Sensing, 43 (3), 492–501, 2005.
- 2. E. Arzuaga-Cruz, L. O. Jimenez-Rodriguez, and M. Velez-Reyes, Unsupervised feature extraction and band subset selection techniques based on relative entropy criteria for hyperspectral data analysis, in Proceeding of SPIE, 5093, 462–473, 2003.
- 3. S. Kawaguchi and R. Nishii, Hyperspectral image classification by bootstrap adaboost with random decision stumps, IEEE Transaction on Geoscience and Remote Sensing, 45 (11), 3845–3851, 2007.
- 4. F. Melgani and L. Bruzzone, Classification of hyperspectral remote sensing images with support vector machines, IEEE Transaction on Geoscience and Remote Sensing, 42 (8), 1778–1790, 2004.
- 5. J. Li, J. M. Bioucas-Dias, and A. Plaza, Spectral–spatial classification of hyperspectral data using loopy belief propagation and active learning, IEEE Transaction on Geoscience and Remote Sensing, 51(2), 844–856, 2013.
- 6. G. Camps-Valls and L. Bruzzone, Kernel-based methods for hyperspectral image classification, IEEE Transaction on Geoscience and Remote Sensing, 43 (6), 1351–1362, 2005.
- 7. Hanbay K., Hyperspectral image classification using convolutional neural network and twodimensional complex Gabor transform, Journal of the Faculty of Engineering and Architecture of Gazi University, 35 (1), 443-456, 2019.
- 8. Hong, D., Gao, L., Yao, J., Zhang, B., Plaza, A., Chanussot, J, Graph convolutional networks for hyperspectral image classification. IEEE Transactions on Geoscience and Remote Sensing, 2020.
- 9. Y. Tarabalka, J. Chanussot, and J. A. Benediktsso, Classification based marker selection for watershed transform of hyperspectral images, in IEEE International on Geoscience and Remote Sensing Symposium, IGARSS’09, 3, III–105, 2009.
- 10. B. Fulkerson, A. Vedaldi, and S. Soatto, Class segmentation and object localization with superpixel neighborhoods, in IEEE 12th International Conference on Computer Vision (ICCV), 670–677, 2009.
- 11. K.-Y. Chang, T.-L. Liu, H.-T. Chen, and S.-H. Lai, Fusing generic objectness and visual saliency for salient object detection, in IEEE International Conference on Computer Vision (ICCV), 914–921, 2011.
- 12. S. Wang, H. Lu, F. Yang, and M.-H. Yang, Superpixel tracking, in IEEE International Conference on Computer Vision (ICCV), 1323– 1330, 2011.
- 13. Karaca A, Güllü M., Detection of forest fire in Menderes district using a superpixel segmentation based search method, Journal of the Faculty of Engineering and Architecture of Gazi University, 34 (2), 1061-1076, 2019.
- 14. Felzenszwalb, P.F., & Huttenlocher, D.P., Efficient graph-based image segmentation. International journal of computer vision, 59 (2), 167-181, 2004.
- 15. Vedaldi, A., & Soatto, S., Quick shift and kernel methods for mode seeking. In European conference on computer vision, Springer, Berlin, Heidelberg, 705-718, October 2008.
- 16. R. Achanta, A. Shaji, K. Smith, A. Lucchi, P. Fua, and S. Susstrunk, Slic superpixels compared to state-of-the-art superpixel methods, IEEE Transaction on Pattern Analysis and Machine Intelligence, 34, 2274– 2282, 2012.
- 17. Huang, W., Huang, Y., Wang, H., Liu, Y., & Shim, H. J., Local binary patterns and superpixel-based multiple kernels for hyperspectral image classification. IEEE Journal of Selected Topics in Applied Earth Observations and Remote Sensing, 13, 4550-4563, 2020.
- 18. Zhang, Y., Jiang, X., Wang, X., Cai, Z., Spectral-spatial hyperspectral image classification with superpixel pattern and extreme learning machine. Remote Sensing, 11 (17), 1983, 2019.
- 19. L. Fang, S. Li, W. Duan, J. Ren, and J. A. Benediktsson, Classification of hyperspectral images by exploiting spectral–spatial information of superpixel via multiple kernels, IEEE Transaction on Geoscience and Remote Sensing, 53(12), 6663–6674, 2015.
- 20. Zhang, Y., Liu, K., Dong, Y., Wu, K., & Hu, X. (2019). Semisupervised classification based on SLIC segmentation for hyperspectral image. IEEE Geoscience and Remote Sensing Letters, 17 (8), 1440-1444.
- 21. Zhang, X., Chew, S. E., Xu, Z., & Cahill, N. D., SLIC superpixels for efficient graph-based dimensionality reduction of hyperspectral imagery. In Algorithms and Technologies for Multispectral, Hyperspectral, and Ultraspectral Imagery XXI, International Society for Optics and Photonics, 705-718, May.2015.
- 22. Zhao, P., Zhou, S., Yang, Y., & Hu, Y., Classification method of hyperspectral remote sensing image based on SLIC and active learning. Computer Engineering & Applications, 3, 2017.
- 23. M. Ester, H.-P. Kriegel, J. Sander, X. Xu, et al., A density-based algorithm for discovering clusters in large spatial databases with noise., in KDD, 96, 226–231, 1996.
- 24. Zhang, F., Li, Z., Qu, X., & Zhang, X., Inverse halftoning algorithm based on slic superpixels and dbscan clustering. In International Conference on Intelligent Computing, Springer, Cham., 466-471, August 2018.
- 25. Albayrak, A., & Bilgin, G. (2019). Automatic cell segmentation in histopathological images via two-staged superpixel-based algorithms. Medical & biological engineering & computing, 57 (3), 653-665.
- 26. Hu, H., Liu, B., Guo, W., Zhang, Z., & Yu, W., Superpixel generation for SAR images based on DBSCAN clustering and probabilistic patch- based similarity. In 2017 IEEE International Geoscience and Remote Sensing Symposium (IGARSS), IEEE, 803-806, July, 2017.
- 27. Z. Wang and A. C. Bovik, A universal image quality index, IEEE Signal Processing Letters, 9 (3), 81–84, 2002.
- 28. J. Wang and C.-I. Chang, Independent component analysis-based dimensionality reduction with applications in hyperspectral image anal., IEEE Transaction on Geoscience and Remote Sensing, 44 (6), 1586– 1600, 2006.
- 29. Dell'Acqua, F., Gamba, P., & Ferrari, A., Exploiting spectral and spatial information for classifying hyperspectral data in urban areas. In IGARSS 2003. 2003 IEEE International Geoscience and Remote Sensing Symposium. Proceedings (IEEE Cat. No. 03CH37477), IEEE., 1, 464-466, July 2003.
- 30. Benediktsson, J. A., Palmason, J. A., & Sveinsson, J. R., Classification of hyperspectral data from urban areas based on extended morphological profiles. IEEE Transactions on Geoscience and Remote Sensing, 43 (3), 480-491, 2005.
- 31. Landgrebe, D. A., Signal theory methods in multispectral remote sensing, John Wiley & Sons, 24, 2003.
APA | SIGIRCI I, Bilgin G (2023). Hiperspektral görüntüler için değiştirilmiş SLIC tabanlı süperpiksel bölütleme. , 399 - 408. 10.17341/gazimmfd.835846 |
Chicago | SIGIRCI IBRAHIM ONUR,Bilgin Gokhan Hiperspektral görüntüler için değiştirilmiş SLIC tabanlı süperpiksel bölütleme. (2023): 399 - 408. 10.17341/gazimmfd.835846 |
MLA | SIGIRCI IBRAHIM ONUR,Bilgin Gokhan Hiperspektral görüntüler için değiştirilmiş SLIC tabanlı süperpiksel bölütleme. , 2023, ss.399 - 408. 10.17341/gazimmfd.835846 |
AMA | SIGIRCI I,Bilgin G Hiperspektral görüntüler için değiştirilmiş SLIC tabanlı süperpiksel bölütleme. . 2023; 399 - 408. 10.17341/gazimmfd.835846 |
Vancouver | SIGIRCI I,Bilgin G Hiperspektral görüntüler için değiştirilmiş SLIC tabanlı süperpiksel bölütleme. . 2023; 399 - 408. 10.17341/gazimmfd.835846 |
IEEE | SIGIRCI I,Bilgin G "Hiperspektral görüntüler için değiştirilmiş SLIC tabanlı süperpiksel bölütleme." , ss.399 - 408, 2023. 10.17341/gazimmfd.835846 |
ISNAD | SIGIRCI, IBRAHIM ONUR - Bilgin, Gokhan. "Hiperspektral görüntüler için değiştirilmiş SLIC tabanlı süperpiksel bölütleme". (2023), 399-408. https://doi.org/10.17341/gazimmfd.835846 |
APA | SIGIRCI I, Bilgin G (2023). Hiperspektral görüntüler için değiştirilmiş SLIC tabanlı süperpiksel bölütleme. Gazi Üniversitesi Mühendislik Mimarlık Fakültesi Dergisi, 38(1), 399 - 408. 10.17341/gazimmfd.835846 |
Chicago | SIGIRCI IBRAHIM ONUR,Bilgin Gokhan Hiperspektral görüntüler için değiştirilmiş SLIC tabanlı süperpiksel bölütleme. Gazi Üniversitesi Mühendislik Mimarlık Fakültesi Dergisi 38, no.1 (2023): 399 - 408. 10.17341/gazimmfd.835846 |
MLA | SIGIRCI IBRAHIM ONUR,Bilgin Gokhan Hiperspektral görüntüler için değiştirilmiş SLIC tabanlı süperpiksel bölütleme. Gazi Üniversitesi Mühendislik Mimarlık Fakültesi Dergisi, vol.38, no.1, 2023, ss.399 - 408. 10.17341/gazimmfd.835846 |
AMA | SIGIRCI I,Bilgin G Hiperspektral görüntüler için değiştirilmiş SLIC tabanlı süperpiksel bölütleme. Gazi Üniversitesi Mühendislik Mimarlık Fakültesi Dergisi. 2023; 38(1): 399 - 408. 10.17341/gazimmfd.835846 |
Vancouver | SIGIRCI I,Bilgin G Hiperspektral görüntüler için değiştirilmiş SLIC tabanlı süperpiksel bölütleme. Gazi Üniversitesi Mühendislik Mimarlık Fakültesi Dergisi. 2023; 38(1): 399 - 408. 10.17341/gazimmfd.835846 |
IEEE | SIGIRCI I,Bilgin G "Hiperspektral görüntüler için değiştirilmiş SLIC tabanlı süperpiksel bölütleme." Gazi Üniversitesi Mühendislik Mimarlık Fakültesi Dergisi, 38, ss.399 - 408, 2023. 10.17341/gazimmfd.835846 |
ISNAD | SIGIRCI, IBRAHIM ONUR - Bilgin, Gokhan. "Hiperspektral görüntüler için değiştirilmiş SLIC tabanlı süperpiksel bölütleme". Gazi Üniversitesi Mühendislik Mimarlık Fakültesi Dergisi 38/1 (2023), 399-408. https://doi.org/10.17341/gazimmfd.835846 |