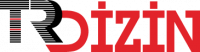
Evrişimsel sinir ağı ve iki-boyutlu karmaşık Gabor dönüşümü kullanılarak hiperspektral görüntü sınıflandırma
Yıl: 2020 Cilt: 35 Sayı: 1 Sayfa Aralığı: 443 - 456 Metin Dili: Türkçe DOI: 10.17341/gazimmfd.479086 İndeks Tarihi: 07-01-2021
Evrişimsel sinir ağı ve iki-boyutlu karmaşık Gabor dönüşümü kullanılarak hiperspektral görüntü sınıflandırma
Öz: Bu çalışmada 2-boyutlu karmaşık Gabor filtreleme ve derin evrişimsel sinir ağları kullanılarak yeni birhiperspektral görüntü sınıflandırma yöntemi önerilmiştir. Spesifik olarak, derin bir öğrenme modeli olarak,evrişimsel sinir ağının kendine özgü üst düzey özellikler çıkarması amaçlanmaktadır. Derin öğrenilen veGabor özellik çıkarma metodolojileri giriş hiperspekral örnekler üzerinde eş zamanlı olarakgerçekleştirilmiştir. Gabor özellikleri hiperspektral görüntünün sadece ilk üç temel bileşeni üzerindekarmaşık Gabor filtreleme uygulanarak hesaplanmaktadır. Önerilen hibrit model Gabor dönüşümünükenarlar, köşeler ve doku gibi bölgesel görüntü özelliklerini elde etmek için kullanmaktadır. GörüntülerinGabor özellikleri çoklu yönelimlerde ve frekanslarda hesaplanır. Sonra derin özellikler ve Gabor özellikleridaha güçlü ve ayırt edici özellik vektörü elde etmek için birleştirilir. Hibrit özellik vektörü hiperspektralgörüntü sınıflandırmak için softmax sınıflandırıcıya giriş olarak kullanılır. Önerilen derin öğrenmemimarisinin parametreleri küçük bir eğitim kümesi kullanılarak optimize edilmiştir. Böylece önerilenevrişimsel sinir ağının ezberleme problemi bir dereceye kadar azaltılmıştır. İki popüler hiperspektral veri setiüzerinde gerçekleştirilen deneyler önerilen yöntemin bazı geleneksel yöntemlerden daha iyi sınıflandırmaperformansı elde edebildiğini göstermiştir. Sınıflandırma sonuçları önerilen hibrit modelin hiperspektralgörüntülerin sınıflandırılması ve özelliklerinin çıkarılması için etkili bir yöntem olduğunu göstermektedir.
Anahtar Kelime: Hyperspectral image classification using convolutional neural network and twodimensional complex Gabor transform
Öz: In this paper, a new hyperspectral image classification method based on 2-dimensional complex Gabor filtering and deep convolutional neural networks is proposed. Specifically, as a deep learning model, convolutional neural network is aimed to extract distinctive high-level features. Deep-learned and Gabor feature extraction methodologies are simultaneously performed on the input hyperspectral samples. Gabor features are calculated by implementing complex Gabor filtering only on the first three principal components of the hyperspectral image. The proposed hybrid model uses Gabor transform to obtain local image features, such as edges, corners and texture. The Gabor features of the images are calculated at multiple orientations and frequencies. Then, deep features and Gabor features are fused to obtain a more robust and discriminative feature vector. Hybrid feature vector is used as input to a softmax classifier for hyperspectral image classification. The parameters of the proposed deep learning architecture are optimized using a small training set. Thus, the over-fitting problem of the proposed convolutional neural network has been reduced to some extent. Experiments performed on two popular hyperspectral datasets show that the proposed method can achieve better classification performance than some conventional methods. Classification results demonstrates that the proposed hybrid model is an efficient method for feature extraction and classification of hyperspectral images.
Anahtar Kelime: Belge Türü: Makale Makale Türü: Araştırma Makalesi Erişim Türü: Erişime Açık
- Kang, X., Zhang, X., Li, S., Li, K., Li, J., Benediktsson, J.A., Hyperspectral Anomaly Detection With Attribute and Edge-Preserving Filters, IEEE Trans. Geosci. Remote Sens., 55 (10), 5600–5611, 2017.
- 2 Lanthier, Y., Bannari, A., Haboudane, D., Miller, J.R., Tremblay, N., Hyperspectral Data Segmentation and Classification in Precision Agriculture: A Multi-Scale Analysis, IEEE International Geoscience and Remote Sensing Symposium, 585-588, 2008.
- 3 Hörig, B., Kühn, F., Oschütz, F., Lehmann, F., HyMap hyperspectral remote sensing to detect hydrocarbons, Int. J. Remote Sens., 22 (8), 1413–1422, 2001.
- 4 Blanzieri, E., Melgani, F., Nearest Neighbor Classification of Remote Sensing Images With the Maximal Margin Principle, IEEE Trans. Geosci. Remote Sens., 46 (6), 1804–1811, 2008.
- 5 Melgani, F., Bruzzone, L., Classification of hyperspectral remote sensing images with support vector machines, IEEE Trans. Geosci. Remote Sens., 42 (8), 1778–1790, 2004.
- 6 Ratle, F., Camps-Valls, G., Weston, J., Semisupervised Neural Networks for Efficient Hyperspectral Image Classification, IEEE Trans. Geosci. Remote Sens., 48 (5), 2271–2282, 2010.
- 7 Kang, X., Li, S., Fang, L., Benediktsson, J.A., Intrinsic Image Decomposition for Feature Extraction of Hyperspectral Images, IEEE Trans. Geosci. Remote Sens., 53 (4), 2241–2253, 2015.
- 8 Prasad, S., Bruce, L.M., Limitations of Principal Components Analysis for Hyperspectral Target Recognition, IEEE Geosci. Remote Sens. Lett., , 5 (4), 625–629, 2008.
- 9 Tarabalka, Y., Fauvel, M., Chanussot, J., Benediktsson, J.A., SVM- and MRF-Based Method for Accurate Classification of Hyperspectral Images, IEEE Geosci. Remote Sens. Lett., 7 (4), 736–740, 2010.
- 10 Li, L., Wang, C., Li, W., Chen, J., Hyperspectral image classification by AdaBoost weighted composite kernel extreme learning machines, Neurocomputing, 275, 1725–1733, 2018.
- 11 Li, W., Du, Q., Zhang, F., Hu, W., Hyperspectral Image Classification by Fusing Collaborative and Sparse Representations, IEEE J. Sel. Top. Appl. Earth Obs. Remote Sens., 9 (9), 4178–4187, 2016.
- 12 Debba, P., van Ruitenbeek, F.J.A., van der Meer, F.D., Carranza, E.J.M., Stein, A., Optimal field sampling for targeting minerals using hyperspectral data, Remote Sens. Environ., 99 (4), 373–386, 2005.
- 13 Li, Y., Xie, W., Li, H., Hyperspectral image reconstruction by deep convolutional neural network for classification, Pattern Recognit., 63, 371–383, 2016.
- 14 Yu, S., Jia, S., Xu, C., Convolutional neural networks for hyperspectral image classification, Neurocomputing, 219 (5), 88–98, 2017.
- 15 Chen, Y., Lin, Z., Zhao, X., Wang, G., Gu, Y., Deep Learning-Based Classification of Hyperspectral Data, IEEE J. Sel. Top. Appl. Earth Obs. Remote Sens., 7 (6), 2094–2107, 2014.
- 16 Hu, W., Huang, Y., Wei, L., Zhang, F., Li, H., Deep Convolutional Neural Networks for Hyperspectral Image Classification, J. Sensors, 2015, 1–12, 2015.
- 17 Zabalza, J., Ren, J., Zheng, J., et al., Novel segmented stacked autoencoder for effective dimensionality reduction and feature extraction in hyperspectral imaging, Neurocomputing, 185, 1–10, 2016.
- 18 Li, W., Wu, G., Zhang, F., Du, Q., Hyperspectral Image Classification Using Deep Pixel-Pair Features, IEEE Trans. Geosci. Remote Sens., 55 (2), 844–853, 2017.
- 19 Kang, X., Li, C., Li, S., Lin, H., Classification of Hyperspectral Images by Gabor Filtering Based Deep Network, IEEE J. Sel. Top. Appl. Earth Obs. Remote Sens., 11 (4), 1166–1178, 2018.
- 20 Shi, C., Pun, C.-M., 3D multi-resolution wavelet convolutional neural networks for hyperspectral image classification, Inf. Sci., 420, 49–65, 2017.
- 21 Cai, L., Zhu, J., Zeng, H., Chen, J., Cai, C., Ma, K.-K., HOG-assisted deep feature learning for pedestrian gender recognition, J. Franklin Inst., 355 (4), 1991– 2008, 2018.
- 22 Altun, A.A., Allahverdi, N., A new approach to recognition of fingerprints enhanced by filtering techniques with artifıcial neural networks, Journal of the Faculty of Engineering and Architecture of Gazi University, 22(2), 227–236, 2007.
- 23 Kim, J., Um, S., Min, D., Fast 2D Complex Gabor Filter With Kernel Decomposition, IEEE Trans. Image Process., 27 (4), 1713–1722, 2018.
- 24 Bernardino, A., Santos-Victor, J., Fast IIR Isotropic 2-D Complex Gabor Filters With Boundary Initialization, IEEE Trans. Image Process., 15 (11), 3338–3348, 2006.
- 25 Kavitha, K., Arivazhagan, S., Fuzzy inspired image classification algorithm for hyperspectral data using three-dimensional log-Gabor features, Opt. - Int. J. Light Electron Opt., 125 (20), 6236–6241, 2014.
- 26 Zhang, J., Zhao, H., Liang, J., Continuous rotation invariant local descriptors for texton dictionary-based texture classification, Comput. Vis. Image Underst., 117, (1), 56–75, 2013.
- 27 Kaya, A., Keçeli, A.S., Can, A.B., Examination of various classification strategies in classification of lung nodule characteristics, Journal of the Faculty of Engineering and Architecture of Gazi University, 34(2), 709-725, 2019 https: //doi. org/ 10.17341/ gazimmfd.416530.
- 28 Bergstra, J., Bastien, F., Breuleux, O., et al., Theano: Deep Learning on GPUs with Python - LISA - Publications - Aigaion 2.0’, in Proceedings of the NIPS, Big Learning Workshop, 712–721, 2011.
- 29 Li, W., Chen, C., Su, H., Du, Q., Local Binary Patterns and Extreme Learning Machine for Hyperspectral Imagery Classification, IEEE Trans. Geosci. Remote Sens., 53, (7), 3681–3693, 2015.
APA | HANBAY K (2020). Evrişimsel sinir ağı ve iki-boyutlu karmaşık Gabor dönüşümü kullanılarak hiperspektral görüntü sınıflandırma. , 443 - 456. 10.17341/gazimmfd.479086 |
Chicago | HANBAY Kazım Evrişimsel sinir ağı ve iki-boyutlu karmaşık Gabor dönüşümü kullanılarak hiperspektral görüntü sınıflandırma. (2020): 443 - 456. 10.17341/gazimmfd.479086 |
MLA | HANBAY Kazım Evrişimsel sinir ağı ve iki-boyutlu karmaşık Gabor dönüşümü kullanılarak hiperspektral görüntü sınıflandırma. , 2020, ss.443 - 456. 10.17341/gazimmfd.479086 |
AMA | HANBAY K Evrişimsel sinir ağı ve iki-boyutlu karmaşık Gabor dönüşümü kullanılarak hiperspektral görüntü sınıflandırma. . 2020; 443 - 456. 10.17341/gazimmfd.479086 |
Vancouver | HANBAY K Evrişimsel sinir ağı ve iki-boyutlu karmaşık Gabor dönüşümü kullanılarak hiperspektral görüntü sınıflandırma. . 2020; 443 - 456. 10.17341/gazimmfd.479086 |
IEEE | HANBAY K "Evrişimsel sinir ağı ve iki-boyutlu karmaşık Gabor dönüşümü kullanılarak hiperspektral görüntü sınıflandırma." , ss.443 - 456, 2020. 10.17341/gazimmfd.479086 |
ISNAD | HANBAY, Kazım. "Evrişimsel sinir ağı ve iki-boyutlu karmaşık Gabor dönüşümü kullanılarak hiperspektral görüntü sınıflandırma". (2020), 443-456. https://doi.org/10.17341/gazimmfd.479086 |
APA | HANBAY K (2020). Evrişimsel sinir ağı ve iki-boyutlu karmaşık Gabor dönüşümü kullanılarak hiperspektral görüntü sınıflandırma. Gazi Üniversitesi Mühendislik Mimarlık Fakültesi Dergisi, 35(1), 443 - 456. 10.17341/gazimmfd.479086 |
Chicago | HANBAY Kazım Evrişimsel sinir ağı ve iki-boyutlu karmaşık Gabor dönüşümü kullanılarak hiperspektral görüntü sınıflandırma. Gazi Üniversitesi Mühendislik Mimarlık Fakültesi Dergisi 35, no.1 (2020): 443 - 456. 10.17341/gazimmfd.479086 |
MLA | HANBAY Kazım Evrişimsel sinir ağı ve iki-boyutlu karmaşık Gabor dönüşümü kullanılarak hiperspektral görüntü sınıflandırma. Gazi Üniversitesi Mühendislik Mimarlık Fakültesi Dergisi, vol.35, no.1, 2020, ss.443 - 456. 10.17341/gazimmfd.479086 |
AMA | HANBAY K Evrişimsel sinir ağı ve iki-boyutlu karmaşık Gabor dönüşümü kullanılarak hiperspektral görüntü sınıflandırma. Gazi Üniversitesi Mühendislik Mimarlık Fakültesi Dergisi. 2020; 35(1): 443 - 456. 10.17341/gazimmfd.479086 |
Vancouver | HANBAY K Evrişimsel sinir ağı ve iki-boyutlu karmaşık Gabor dönüşümü kullanılarak hiperspektral görüntü sınıflandırma. Gazi Üniversitesi Mühendislik Mimarlık Fakültesi Dergisi. 2020; 35(1): 443 - 456. 10.17341/gazimmfd.479086 |
IEEE | HANBAY K "Evrişimsel sinir ağı ve iki-boyutlu karmaşık Gabor dönüşümü kullanılarak hiperspektral görüntü sınıflandırma." Gazi Üniversitesi Mühendislik Mimarlık Fakültesi Dergisi, 35, ss.443 - 456, 2020. 10.17341/gazimmfd.479086 |
ISNAD | HANBAY, Kazım. "Evrişimsel sinir ağı ve iki-boyutlu karmaşık Gabor dönüşümü kullanılarak hiperspektral görüntü sınıflandırma". Gazi Üniversitesi Mühendislik Mimarlık Fakültesi Dergisi 35/1 (2020), 443-456. https://doi.org/10.17341/gazimmfd.479086 |