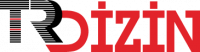
Prediction of Marketing Live Weights in Hair Goat Kids Using Artificial Neural Network
Yıl: 2022 Cilt: 28 Sayı: 6 Sayfa Aralığı: 739 - 746 Metin Dili: İngilizce DOI: 10.9775/kvfd.2022.28078 İndeks Tarihi: 15-05-2023
Prediction of Marketing Live Weights in Hair Goat Kids Using Artificial Neural Network
Öz: In this study, marketing live weights (120th day) were predicted using artificial neural network model according to the herd, gender, birth type, maternal age, birth weight, body weight at 60th day and weaning weight (90th day) measurements of 12983 hair goat kids born between 2018-2021 years. Artificial neural networks (ANN) have been frequently used as an alternative to classical regression analysis in recent years, especially in future estimation studies in the field of livestock, and also in many different fields. In this study, it was aimed to predict the marketing weights of hair goats according to the holding, gender, birth type, maternal age, birth, 60th day and weaning weights with the ANN model. For this purpose, the multi-layer feed-forward backpropagation algorithm the ANN model, in which the number of hidden layers is one and the numbers of hidden neurons are three, was used. This model performance metrics were obtained for training set as 0.98, 0.62 and 0.55; for validation set as 0.97, 0.62 and 0.55, respectively. According to these results, it was determined that ANN can be used successfully in terms of estimation of marketing live weight in Hair goat kids. Estimating the marketing weight will enable the economic cost calculations to be obtained from kids to be evaluated both based on Turkey and on the farm basis, and to reveal future projections.
Anahtar Kelime: Kıl Keçisi Oğlaklarında Pazarlama Canlı Ağırlığının Yapay Sinir Ağları Kullanılarak Tahminlenmesi
Öz: Bu çalışmada 2018-2021 yılları arasında doğan 12983 baş Kıl keçisi oğlaklarının sürü, cinsiyet, doğum tipi, ana yaşı, doğum ağırlığı, 60. gün canlı ağırlık ve sütten kesim canlı ağırlık (90. gün ağırlığı) ölçümlerinin dikkate alındığı yapay sinir ağları modelinde pazarlama canlı ağırlıkları (120. gün ağırlığı) tahminlenmiştir. Yapay sinir ağları (YSA) pek çok farklı alanda olduğu gibi son yıllarda hayvancılık alanında özellikle de geleceğe yönelik tahminleme çalışmalarında klasik regresyon analizine alternatif olarak sıklıkla kullanılmaya başlanan bir veri madenciliği yöntemidir. Çalışmada işletme, cinsiyet, doğum tipi, anne yaşı, doğum, 60. gün ve sütten kesim ağırlıklarına göre kıl keçisi pazarlama ağırlıklarının YSA modeli ile tahmin edilmesi amaçlanmıştır. Bu amaçla, gizli katman sayısının bir ve gizli nöron sayısının üç olduğu çok katmanlı ileri beslemeli geri yayılım algoritması YSA modeli kullanılmıştır. Bu model performans kriter değerleri eğitim seti için sırasıyla 0.98, 0.62 ve 0.55 ve doğrulama seti için 0.97, 0.62 ve 0.55 olarak elde edilmiştir. Bu sonuçlara göre, Kıl keçisi oğlaklarında pazarlama canlı ağırlığının tahmini bakımından YSA yönteminin başarıyla kullanılabileceği belirlenmiştir. Pazarlama ağırlığının tahmin edilebilmesi, oğlaklardan elde edilecek ekonomik maliyet hesaplarının hem Türkiye hem de çiftlik bazında önceden değerlendirilmesine ve geleceğe yönelik projeksiyonların ortaya çıkarılmasına olanak sağlayacaktır.
Anahtar Kelime: Belge Türü: Makale Makale Türü: Araştırma Makalesi Erişim Türü: Erişime Açık
- 1. Atac FE, Burcu H: The importance of hair goats in Turkey. J Agric Sci Tech A, 4, 364-369, 2014.
- 2. Kaymakci M: Koyunlarda Genetik Islah. In, İleri Koyun Yetiştiriciliği. 5th ed., 145-230, İzmir, 2016.
- 3. Elmaz O, Saatci M: Turkish air Goat, the Main Pillar of Goat Population in Turkey. In, Simões J, Gutiérrez C (Eds): Sustainable Goat Production in Adverse Environments: Volume II. 113-130, 2017. Springer, Cham. DOI: 10.1007/978-3-319-71294-9_9
- 4. TUIK: Hayvansal Üretim İstatistikleri, 2021. https://biruni.tuik.gov.tr/ medas, Accessed at: 09.02.2022.
- 5. Kaymakci M, Engindeniz S: Türkiye keçi yetiştiriciliği; sorunlar ve teknik-ekonomik çözümler, Ulusal Keçicilik Kongresi, 24 Haziran 2010, Çanakkale, 1-25, 2010.
- 6. HAYGEM: Hayvancılık Genel Müdürlüğü, 2022. Hayvancılık Verileri. https://www.tarimorman.gov.tr. HAYGEM. Pdf, Accessed: 05.07.2022.
- 7. Saatci M, Dewi IA, Ulutas Z: Variance components due to direct and maternal effects and estimation of breeding values for 12-week weight of Welsh Mountain lambs. Anim Sci, 69 (2): 345-352, 1999. DOI: 10.1017/ S1357729800050918
- 8. Eyduran E, Waheed A, Tariq MM, Iqbal F, Ahmad S: Prediction of live weight from morphological characteristics of commercial goat in Pakistan using factor and principal component scores in multiple linear regression. J Anim Plant Sci, 23, 1532-1540, 2013.
- 9. Yilmaz O, Cemal I, Karaca O: Estimation of mature live weight using some body measurements in Karya sheep. Trop Anim Health Prod, 45, 397- 403, 2013. DOI: 10.1007/s11250-012-0229-7
- 10. Khan MA, Tariq MM, Eyduran E, Tatliyer A, Rafeeq M, Abbas F, Rashid N, Awan MA, Javed K: Estimating body weight from several body measurements in Harnai sheep without multicollinearity problem. J Anim Plant Sci, 24 (1): 120-126, 2014.
- 11. Cam MA, Olfaz M, Soydan E: Possibilities of using morphometrics characteristics as a tool for body weight prediction in Turkish hair goats (Kilkeci). Asian J Anim Vet Adv, 5 (1): 52-59, 2010. DOI: 10.3923/ ajava.2010.52.59
- 12. Celik S: Comparing predictive performances of tree-based data mining algorithms and MARS algorithm in the prediction of live body weight from body traits in Pakistan goats. Pak J Zool, 51 (4): 1447-1456, 2019. DOI: 10.17582/journal.pjz/2019.51.4.1447.1456
- 13. Eyduran E, Karakus K, Karakus S, Cengiz F: Usage of factor scores for determining relationships among body weight and body measurements. Bulg J Agric Sci, 15 (4): 373-377, 2009.
- 14. Yakubu A: Fixing collinearity instability in the estimation of body weight from morphobiometrical traits of West African Dwarf goats. Trakia J Sci, 7 (2): 61-66, 2009.
- 15. Huma ZE, Iqbal F: Predicting the body weight of Balochi sheep using a machine learning approach. Turk J Vet Anim Sci, 43, 500-506, 2019. DOI: 10.3906/vet-1812-23
- 16. Mendes M, Akkartal E: Regression tree analysis for predicting slaughter weight in broilers. Ital J Anim Sci, 8 (4): 615-624, 2009. DOI: 10.4081/ ijas.2009.615
- 17. Njubi DM, Wakhungu JW, Badamana MS: Use of test-day records to predict first lactation 305-day milk yield using artificial neural network in Kenyan Holstein-Friesian dairy cows. Trop Anim Health Prod, 42 (4): 639- 644, 2010. DOI: 10.1007/s11250-009-9468-7
- 18. Pour Hamidi S, Mohammadabadi MR, Foozi MA, Nezamabadi-pour H: Prediction of breeding values for the milk production trait in Iranian Holstein cows applying artificial neural networks. J Livest Sci Technol, 5 (2): 53-61, 2017. DOI: 10.22103/JLST.2017.10043.1188
- 19. Grzesiak W, Lacroix R, Wójcik J, Blaszczyk P: A comparison of neural network and multiple regression predictions for 305-day lactation yield using partial lactation records. Can J Anim Sci, 83 (2): 307-310, 2003. DOI: 10.4141/A02-002
- 20. Sharma AK, Sharma RK, Kasana HS: Prediction of first lactation 305- day milk yield in Karan Fries dairy cattle using ANN modeling. Appl Soft Comput, 7 (3): 1112-1120, 2007. DOI: 10.1016/j.asoc.2006.07.002
- 21. Ghotbaldini H, Mohammadabadi M, Nezamabadi-pour H, Babenko OI, Bushtruk MV, Tkachenko SV: Predicting breeding value of body weight at 6-month age using Artificial Neural Networks in Kermani sheep breed. Acta Sci Anim Sci, 41, 1-9, 2019. DOI: 10.4025/actascianimsci.v41i1.45282
- 22. Moaeen-ud-Din M, Ahmad N, Iqbal A, Abdullah M: Evaluation of different formulas for weight estimation in Beetal, Teddi and crossbred (Beetal x Teddi) goats. J Anim Plant Sci, 16 (3-4): 74-78, 2006.
- 23. Ehret A, Hochstuhl D, Gianola D, Thaller G: Application of neural networks with back-propagation to genome-enabled prediction of complex traits in Holstein-Friesian and German Fleckvieh cattle. Genet Sel Evol, 47:22, 2015. DOI: 10.1186/s12711-015-0097-5
- 24. Lopes BF, Magnabosco CU, Passafaro TL, Brunes LC, Costa MFO, Eifert EC, Narciso MG, Rosa GJM, Lobo RB, Baldi F: Improving genomic prediction accuracy for meat tenderness in Nellore cattle using artificial neural networks. J Anim Breed Genet, 137 (5): 438-448, 2020. DOI: 10.1111/jbg.12468
- 25. Saini LM: Peak load forecasting using Bayesian regularization, Resilient and adaptive backpropagation learning based artificial neural networks. Electr Power Syst Res, 78 (7): 1302-1310, 2008. DOI: 10.1016/j.epsr.2007.11.003
- 26. Kucukonder H, Boyaci S, Akyuz A: A modeling study with an articial neural network: Developing estimation models for the tomato plant leaf area. Turk J Agric For, 40, 203-212, 2016. DOI: 10.3906/tar-1408-28
- 27. Jassim HSH: Artificial neural networks as a technique in construction engineering and management. Luleå University of Technology, 1-24, 2018. http://ltu.diva-portal.org/smash/get/diva2:1230835/FULLTEXT04.pdf; Accessed: 03.07.2022.
- 28. Haykin S: Neural networks and learning machines. 3rd ed., 906, Pearson Prentice Hall, New Jersey, 2009.
- 29. Grzesiak W, Zaborski D: Examples of the use of data mining methods in animal breeding. In, Karahoca A (Ed): Data Mining Applications in Engineering and Medicine. Chapter 13, 303-324, 2012. DOI: 10.5772/50893
- 30. Keskenler MF, Keskenler EF: Geçmişten günümüze yapay sinir ağları ve tarihçesi. Takvim-i Vekayi, 5 (2): 8-18, 2017.
- 31. Oztemel E: Yapay sinir ağları. Papatya Yayıncılık Eğitim, 232, İstanbul, 2012.
- 32. Takma C, Atıl H, Aksakal V: Çoklu doğrusal regresyon ve yapay sinir ağı modellerinin laktasyon süt verimlerine uyum yeteneklerinin karşılaştırılması. Kafkas Univ Vet Fak Derg, 18 (6): 941-944, 2012. DOI: 10.9775/kvfd.2012.6764
- 33. Eyduran E, Zaborski D, Waheed A, Celik S, Karadas K, Grzesiak W: Comparison of the predictive capabilities of several data mining algorithms and multiple linear regression in the prediction of body weight by means of body measurements in the indigenous Beetal Goat of Pakistan. Pak J Zool, 49 (1): 257-265, 2017.
- 34. Tasdemir S, Ozkan IA: ANN approach for estimation of cow weight depending on photogrammetric body dimensions. IJEG, 4 (1): 36-44, 2019. DOI: 10.26833/ijeg.427531
- 35. Tırınk C: Comparison of bayesian regularized neural network, random forest regression, support vector regression and multivariate adaptive regression splines algorithms to predict body weight from biometrical measurements in Thalli sheep. Kafkas Univ Vet Fak Derg, 28 (3): 411-419, 2022. DOI: 10.9775/kvfd.2022.27164
- 36. Behzadi MRB, Aslaminejad AA: A comparison of neural network and nonlinear regression predictions of sheep growth. J Anim Vet Adv, 9 (16): 2128-2131, 2010. DOI: 10.3923/javaa.2010.2128.2131
- 37. Ali M, Eyduran E, Tariq MM, Tirink C, Abbas F, Bajwa MA, Baloch MH, Nizamani AH, Waheed A, Awan MA, Shah SH, Ahmad Z, Jan S: Comparison of artificial neural network and decision tree algorithms used for predicting live weight at post weaning period from some biometrical characteristics in Harnai sheep. Pak J Zool, 47 (6): 1579-1585, 2015.
- 38. Norouzian MA, Vakili Alavijeh M: Comparison of artificial neural network and multiple regression analysis for prediction of fat tail weight of sheep. Iran J Appl Anim Sci, 6 (4): 895-900, 2016.
- 39. Karadas K, Tariq M, Tariq MM, Eyduran E: Measuring predictive performance of data mining and artificial neural network algorithms for predicting lactation milk yield in indigenous Akkaraman sheep. Pak J Zool, 49 (1): 1-7, 2017.
- 40. Yücedag N: İvesi koyunlarında laktasyon süt verimlerinin çoklu doğrusal regresyon ve yapay sinir ağı modelleriyle karşılaştırmalı incelenmesi. Yüksek Lisans Tezi, Ankara Üniv. Fen Bil. Enst., 2019.
- 41. Celik S, Eyduran E, Karadas K, Tariq MM: Comparison of predictive performance of data mining algorithms in predicting body weight in Mengali rams of Pakistan. R Bras Zootec, 46 (11): 863-872, 2017. DOI: 10.1590/S1806-92902017001100005
- 42. Ruhil AP, Raja TV, Gandhi RS: Preliminary study on prediction of body weight from morphometric measurements of goats through ANN models. J Indian Soc Agric Stat, 67 (1): 51-58, 2013.
- 43. Kaygısız F, Sezgin FH: Forecasting goat milk production ın turkey using artificial neural networks and box-jenkins models. Anim Rev, 4 (3): 45-52, 2017. DOI: 10.18488/journal.ar.2017.43.45.52
- 44. Kannan G, Gosukonda R, Mahapatra AK: Prediction of stress responses in goats: Comparison of artificial neural network and multiple regression models. Can J Anim Sci, 100 (1): 1-26, 2019. DOI: 10.1139/cjas-2019-0028
- 45. Khorshidi-Jalali M, Mohammadabadi MR, Esmailizadeh A, Barazandeh A, Babenko OI: Comparison of artificial neural network and regression models for prediction of body weight in Raini Cashmere goat. Iran J Appl Anim Sci, 9 (3): 453-461, 2019.
- 46. Cihan P, Gökçe E, Atakişi O, Kırmızıgül AH, Erdoğan HM: Prediction of Immunoglobulin G in lambs with artificial intelligence methods. Kafkas Univ Vet Fak Derg, 27 (1): 21-27, 2021. DOI: 10.9775/kvfd.2020.24642
- 47. Ekiz B, Baygul O, Yalcintan H, Ozcan M: Comparison of the decision tree, artificial neural network and multiple regression methods for prediction of carcass tissues composition of goat kids. Meat Sci, 161, 1-10, 2019. DOI: 10.1016/j.meatsci.2019.108011
APA | erdoğan ataç f, TAKMA Ç, Gevrekci Y, Ozis Altincekic S (2022). Prediction of Marketing Live Weights in Hair Goat Kids Using Artificial Neural Network. , 739 - 746. 10.9775/kvfd.2022.28078 |
Chicago | erdoğan ataç funda,TAKMA Çiğdem,Gevrekci Yakut,Ozis Altincekic Seniz Prediction of Marketing Live Weights in Hair Goat Kids Using Artificial Neural Network. (2022): 739 - 746. 10.9775/kvfd.2022.28078 |
MLA | erdoğan ataç funda,TAKMA Çiğdem,Gevrekci Yakut,Ozis Altincekic Seniz Prediction of Marketing Live Weights in Hair Goat Kids Using Artificial Neural Network. , 2022, ss.739 - 746. 10.9775/kvfd.2022.28078 |
AMA | erdoğan ataç f,TAKMA Ç,Gevrekci Y,Ozis Altincekic S Prediction of Marketing Live Weights in Hair Goat Kids Using Artificial Neural Network. . 2022; 739 - 746. 10.9775/kvfd.2022.28078 |
Vancouver | erdoğan ataç f,TAKMA Ç,Gevrekci Y,Ozis Altincekic S Prediction of Marketing Live Weights in Hair Goat Kids Using Artificial Neural Network. . 2022; 739 - 746. 10.9775/kvfd.2022.28078 |
IEEE | erdoğan ataç f,TAKMA Ç,Gevrekci Y,Ozis Altincekic S "Prediction of Marketing Live Weights in Hair Goat Kids Using Artificial Neural Network." , ss.739 - 746, 2022. 10.9775/kvfd.2022.28078 |
ISNAD | erdoğan ataç, funda vd. "Prediction of Marketing Live Weights in Hair Goat Kids Using Artificial Neural Network". (2022), 739-746. https://doi.org/10.9775/kvfd.2022.28078 |
APA | erdoğan ataç f, TAKMA Ç, Gevrekci Y, Ozis Altincekic S (2022). Prediction of Marketing Live Weights in Hair Goat Kids Using Artificial Neural Network. Kafkas Üniversitesi Veteriner Fakültesi Dergisi, 28(6), 739 - 746. 10.9775/kvfd.2022.28078 |
Chicago | erdoğan ataç funda,TAKMA Çiğdem,Gevrekci Yakut,Ozis Altincekic Seniz Prediction of Marketing Live Weights in Hair Goat Kids Using Artificial Neural Network. Kafkas Üniversitesi Veteriner Fakültesi Dergisi 28, no.6 (2022): 739 - 746. 10.9775/kvfd.2022.28078 |
MLA | erdoğan ataç funda,TAKMA Çiğdem,Gevrekci Yakut,Ozis Altincekic Seniz Prediction of Marketing Live Weights in Hair Goat Kids Using Artificial Neural Network. Kafkas Üniversitesi Veteriner Fakültesi Dergisi, vol.28, no.6, 2022, ss.739 - 746. 10.9775/kvfd.2022.28078 |
AMA | erdoğan ataç f,TAKMA Ç,Gevrekci Y,Ozis Altincekic S Prediction of Marketing Live Weights in Hair Goat Kids Using Artificial Neural Network. Kafkas Üniversitesi Veteriner Fakültesi Dergisi. 2022; 28(6): 739 - 746. 10.9775/kvfd.2022.28078 |
Vancouver | erdoğan ataç f,TAKMA Ç,Gevrekci Y,Ozis Altincekic S Prediction of Marketing Live Weights in Hair Goat Kids Using Artificial Neural Network. Kafkas Üniversitesi Veteriner Fakültesi Dergisi. 2022; 28(6): 739 - 746. 10.9775/kvfd.2022.28078 |
IEEE | erdoğan ataç f,TAKMA Ç,Gevrekci Y,Ozis Altincekic S "Prediction of Marketing Live Weights in Hair Goat Kids Using Artificial Neural Network." Kafkas Üniversitesi Veteriner Fakültesi Dergisi, 28, ss.739 - 746, 2022. 10.9775/kvfd.2022.28078 |
ISNAD | erdoğan ataç, funda vd. "Prediction of Marketing Live Weights in Hair Goat Kids Using Artificial Neural Network". Kafkas Üniversitesi Veteriner Fakültesi Dergisi 28/6 (2022), 739-746. https://doi.org/10.9775/kvfd.2022.28078 |