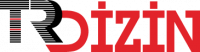
Hile Tespitinde Makine Öğrenmesi Yöntemlerinin Kullanılması ve Model Performanslarının Değerlendirilmesi
Yıl: 2022 Cilt: 14 Sayı: 4 Sayfa Aralığı: 3053 - 3065 Metin Dili: Türkçe DOI: 10.20491/isarder.2022.1549 İndeks Tarihi: 17-05-2023
Hile Tespitinde Makine Öğrenmesi Yöntemlerinin Kullanılması ve Model Performanslarının Değerlendirilmesi
Öz: Amaç – Çalışmada, hilenin verdiği zararın azaltılmasına yönelik teknoloji temelli araçlarla çözüm üretilmeye çalışılmıştır. İşletmelerde sıklıkla karşılaşılan hileli ödemelerin tespiti için makine öğrenimi yöntemleriyle bir model oluşturulması amaçlanmaktadır. Yöntem – Çalışmada, bir bankaya ait finansal ve finansal olmayanlar bilgilerle oluşturulan 594.643 adetlik yapay veri setinden yararlanılmıştır. Veri seti kullanılarak makine öğrenmesinin Karar Ağacı, Destek Vektör Makinesi, Lojistik Regresyon ve Yapay Sinir Ağları yöntemleriyle tahmin yapılmıştır. Yöntemlerin algoritmaları veri setinin %69’u ile önce eğitilmiş, sonra veri setinin %31’i ile tahmin yapması sağlanmıştır. Bulgular - Yöntemlerin oluşturduğu değerlere bakıldığında doğruluk metriği sırasıyla Karar Ağacı %99,42, Destek Vektör Makinesi %99,11, Lojistik Regresyon %98,95 ve Yapay Sinir Ağları %99,35 hesaplandığı görülmüştür. 1.620 tane hileli işlemi doğru tahmin ederek en fazla doğru tahmin yapan ve bu süreçleri en hızlı (1,32sn) gerçekleştiren yöntem karar ağacı olmuştur. Sonuçlara göre ortalama olarak en başarılı, en hızlı ve en çok doğru tahmin yapan modeli karar ağacının oluşturduğu tespit edilmiştir. Tartışma - Uygulamada kullanılan yöntemlere bağlı olarak veri setiyle yapılan model denemelerinde makine öğrenimi yöntemleri yanlış pozitif ve yanlış negatif değerleri üretmiştir. Her ne kadar bu değerleri sıfıra indirmek mümkün olmasa da azaltılması yönünde geliştirilmeye açıktır.
Anahtar Kelime: Using Machine Learning Methods to Detect Fraud and Evaluation of Model Performances
Öz: Purpose - It has been tried to produce a solution with technology-based tools to reduce the damage caused by fraud in this study. It is aimed to create a model with machine learning methods for the detection of fraudulent payments, which are frequently encountered in businesses. Design/methodology/approach - In the study, an artificial data set of 594.643 created with financial and non-financial information belonging to a bank was used. Using the data set, predictions were made with the Decision Tree, Support Vector Machine, Logistic Regression and Artificial Neural Networks methods of machine learning. Algorithms of the methods were first trained with 69% of the data set and then made predictions with 31% of the data set. Findings - Considering the values created by the methods, it was seen that the accuracy metric was 99.42% for Decision Tree, 99.11% for Support Vector Machine, 98.95% for Logistic Regression and 99.35% for Artificial Neural Networks. Decision tree was the method that predicted 1.620 fraudulent transactions correctly and made the most correct predictions and performed these processes in the fastest (1.32 seconds). According to the results, it has been determined that the decision tree is the model that makes the most successful, fastest and most accurate predictions on average. Discussion - Depending on the methods used in practice, machine learning methods produced false positive and false negative values in model trials with the data set. Although it is not possible to reduce these values to zero, it is open to development to reduce them.
Anahtar Kelime: Belge Türü: Makale Makale Türü: Araştırma Makalesi Erişim Türü: Erişime Açık
- Albrecht, W. Steve ve Conan C. Albrecht. “Chapter 49 Detecting Fraud” , D. R. Carmichael (Ed.), O. Ray Whittington (Ed.), Lynford Graham (Ed.), Accountant’s Handbook Volume Two: Special Industries and Special Topics”, New Jersey: John Wiley & Sons, 2007.
- Albrecht W. Steve, Chad Albrecht ve Conan C. Albrecht. “Current Trends in Fraud and its Detection”, Information Security Journal: A Global Perspective. Vol.17, No.1, March 2008.
- Aksoy,Barış. “Finansal Tablo Hilelerinin Makine Öğrenmesi Yöntemleri ve Lojistik Regresyon Kullanılarak Tahmin Edilmesi: Borsa İstanbul Örneği”, Maliye ve Finans Yazıları. S.115, 2021, s.29-60.
- Association of Certified Fraud Examiners (ACFE), “2018 Global Study on Occupational Fraud and Abuse”, Report to The Nation, USA: 2018, s.10.
- Baesens, Bart, Véronique Van Vlasselaer ve Wouter Verbeke, Fraud Analytics Using Descriptive, Predictive, and Social Network Techniques: A Guide to Data Science for Fraud Detection. First Edition. New Jersey: John Wiley & Sons, 2015.
- Bozkurt, Nejat. İşletmelerin Kara Deliği Hile. 3.Baskı. İstanbul: Alfa Yayınları, 2009.
- Chen, Suduan. “Detection of fraudulent financial statements using the hybrid data mining approach”, SpringerPlus. 5(89), 2016, pp. 1-16.
- Dutta,Ila, Shantanu Dutta ve Bijan Raahemi. “Detecting Financial Restatements Using Data Mining Techniques”, Expert Systems With Applications. V. 90, 2017, pp.374-393.
- Fernandez, George, Data Mining Using SAS Applications, USA: Chapman & Hall/ CRC Press, 2003.
- Fletcher Lu, J. Efrim Boritz ve Dominic Covvey. “Adaptive Fraud Detection Using Benford’s Law”, Conference: Advances in Artificial Intelligence, 19th Conference of the Canadian Society for Computational Studies of Intelligence. Québec, Canada: Springer-Verlag, 7-9 June 2006, s.348-349.
- Golden, Thomas W., Steven L. Skalak, Mona M. Clayton ve Jessica S. Pill. A Guide to Forensic Accounting Investigation. Second Edition. New Jersey: John Wiley & Sons, 2011.
- I, Lægreid. “Automatic Fraud Detection — Does it Work?”, Annals of Actuarial Science. Volume 2, Issue 02, September 2007, pp 271 – 288.
- Jackson, Cecil W. Detecting Accounting Fraud: Analysis and Ethics. Global Edition. England: Pearson Education Limited, 2015.
- Jan, Chyan-long. “An effective financial statements fraud detection model for the sustainable development of financial markets: evidence from Taiwan”, Sustainability. 10(513), 2018,1-14.
- Jans, Mieke, Nadine Lybaert ve Koen Vanhoof, “Data Mining for Fraud Detection: Toward an Improvement on Internal Control Systems?”, 30. Eurapean Accounting Association Congress. Lisbon, 2007,pp.,1-27. https://documentserver.uhasselt.be/bitstream/1942/7872/1/EAA%20lisbon.pdf, [17.06.2022].
- Kantardzic, Mehmed. Data Mining: Concepts, Models, Methods, and Algorithms. Third Edition, New Jersey, John Wiley & Sons,2020.
- Kaggle. Synthetic Data From A Financial Payment System, 2017, https://www.kaggle.com/ealaxi/banksim1 [16Şubat 2022].
- Kırlıoğlu, Hilmi ve İsmail Fatih Ceyhan. “Mali Tablo Denetiminde Ön Analitik İnceleme Tekniği Olarak Veri Madenciliğinin Kullanımı: Borsa İstanbul Uygulaması”, Akademik Yaklaşımlar Dergisi, 5(1), 2014, s.13- 36.
- Kirkos, Efstathios, Charalambos Spathis & Yannis Manolopoulos. “Data mining techniques for the detection of fraudulent financial statements”, Expert Systems with Applications. V. 32, 2007,pp, 995-1003.
- Kotu, Vijay ve Bala Deshpande, Data Science Concepts and Practice, Second Edition, United States, Morgan Kaufmann Publishers, 2019.
- Kudyba, Stephan. Big Data, Mining, and Analytics: Components of Strategic Decision Making. Florida: CRC Press, Taylor & Francis Group. 2014.
- Larose, Daniel T. Discovering Knowledge In Data: An Introduction to Data Mining. New Jersey: John Wiley & Sons, 2005.
- Layton, Robert. Learning Data Mining with Python, First Edition, Birmingham, UK, Packt Publishing Ltd,2015.
- Liou, F. M. Fraudulent Financial Reporting Detection and Business Failure Prediction Models: A Comparison, Managerial Auditing Journal. 23(7), 2008.650-662.
- Memı̇ ş, S, Engı̇ noğlu ve S, Erkan, U. A Data Classification Method in Machine Learning Based on Normalised Hamming Pseudo-Similarity of Fuzzy Parameterized Fuzzy Soft Matrices. Bilge International Journal of Science and Technology Research. Özel Sayı (3) 2019,1-8.
- Padgett, Simon. Profiling the Fraudster: Removing The Mask To Prevent And Detect Fraud. First Edition. New Jersey: John Wiley & Sons, 2015.
- Pehlivanlı, Davut. Hile Denetimi Metodoloji ve Raporlama. İstanbul: Beta Yayınları, 2011.
- Resmî Gazete, “Kişisel verilerin korunması kanunu” 07 Nisan 2016, Sayısı: 29677.
- Roiger, Richard J. Data Mining: A Tutorial-Based Primer. USA: CRC Press, Taylor & Francis Group. 2017.
- Stamler, Rodney T., Hans J. Marschdorf ve Mario Possamai. Fraud Prevention and Detection: Warning Signs and the Red Flag System. USA: Taylor & Francis Group, 2014.
- Statement on Auditing Standards (SAS) No. 99, Consideration of Fraud in a Financial Statement Audit, American Institute of Certified Public Accountants, 2002, s.1721.
- S V S, Lakshmi ve Selvani Deepthi Kavila. “Machine Learning For Credit Card Fraud Detection System”, International Journal of Applied Engineering Research. V.13/4, 2018, pp.16819-16824.
- Terzi, Serkan. “Hile ve Usulsüzlüklerin Tespitinde Veri Madenciliğinin Kullanımı”, Muhasebe ve Finansman Dergisi (MUFAD). Sayı.54, Nisan, 2012, s.51-64.
- Wells, Joseph T. Corporate Fraud Handbook: Prevention and Detection, Fifth Edition, New Jersey: John Wiley & Sons,2017.
APA | GUR O, TARHAN MENGİ B (2022). Hile Tespitinde Makine Öğrenmesi Yöntemlerinin Kullanılması ve Model Performanslarının Değerlendirilmesi. , 3053 - 3065. 10.20491/isarder.2022.1549 |
Chicago | GUR ONDER,TARHAN MENGİ BANU Hile Tespitinde Makine Öğrenmesi Yöntemlerinin Kullanılması ve Model Performanslarının Değerlendirilmesi. (2022): 3053 - 3065. 10.20491/isarder.2022.1549 |
MLA | GUR ONDER,TARHAN MENGİ BANU Hile Tespitinde Makine Öğrenmesi Yöntemlerinin Kullanılması ve Model Performanslarının Değerlendirilmesi. , 2022, ss.3053 - 3065. 10.20491/isarder.2022.1549 |
AMA | GUR O,TARHAN MENGİ B Hile Tespitinde Makine Öğrenmesi Yöntemlerinin Kullanılması ve Model Performanslarının Değerlendirilmesi. . 2022; 3053 - 3065. 10.20491/isarder.2022.1549 |
Vancouver | GUR O,TARHAN MENGİ B Hile Tespitinde Makine Öğrenmesi Yöntemlerinin Kullanılması ve Model Performanslarının Değerlendirilmesi. . 2022; 3053 - 3065. 10.20491/isarder.2022.1549 |
IEEE | GUR O,TARHAN MENGİ B "Hile Tespitinde Makine Öğrenmesi Yöntemlerinin Kullanılması ve Model Performanslarının Değerlendirilmesi." , ss.3053 - 3065, 2022. 10.20491/isarder.2022.1549 |
ISNAD | GUR, ONDER - TARHAN MENGİ, BANU. "Hile Tespitinde Makine Öğrenmesi Yöntemlerinin Kullanılması ve Model Performanslarının Değerlendirilmesi". (2022), 3053-3065. https://doi.org/10.20491/isarder.2022.1549 |
APA | GUR O, TARHAN MENGİ B (2022). Hile Tespitinde Makine Öğrenmesi Yöntemlerinin Kullanılması ve Model Performanslarının Değerlendirilmesi. İşletme Araştırmaları Dergisi, 14(4), 3053 - 3065. 10.20491/isarder.2022.1549 |
Chicago | GUR ONDER,TARHAN MENGİ BANU Hile Tespitinde Makine Öğrenmesi Yöntemlerinin Kullanılması ve Model Performanslarının Değerlendirilmesi. İşletme Araştırmaları Dergisi 14, no.4 (2022): 3053 - 3065. 10.20491/isarder.2022.1549 |
MLA | GUR ONDER,TARHAN MENGİ BANU Hile Tespitinde Makine Öğrenmesi Yöntemlerinin Kullanılması ve Model Performanslarının Değerlendirilmesi. İşletme Araştırmaları Dergisi, vol.14, no.4, 2022, ss.3053 - 3065. 10.20491/isarder.2022.1549 |
AMA | GUR O,TARHAN MENGİ B Hile Tespitinde Makine Öğrenmesi Yöntemlerinin Kullanılması ve Model Performanslarının Değerlendirilmesi. İşletme Araştırmaları Dergisi. 2022; 14(4): 3053 - 3065. 10.20491/isarder.2022.1549 |
Vancouver | GUR O,TARHAN MENGİ B Hile Tespitinde Makine Öğrenmesi Yöntemlerinin Kullanılması ve Model Performanslarının Değerlendirilmesi. İşletme Araştırmaları Dergisi. 2022; 14(4): 3053 - 3065. 10.20491/isarder.2022.1549 |
IEEE | GUR O,TARHAN MENGİ B "Hile Tespitinde Makine Öğrenmesi Yöntemlerinin Kullanılması ve Model Performanslarının Değerlendirilmesi." İşletme Araştırmaları Dergisi, 14, ss.3053 - 3065, 2022. 10.20491/isarder.2022.1549 |
ISNAD | GUR, ONDER - TARHAN MENGİ, BANU. "Hile Tespitinde Makine Öğrenmesi Yöntemlerinin Kullanılması ve Model Performanslarının Değerlendirilmesi". İşletme Araştırmaları Dergisi 14/4 (2022), 3053-3065. https://doi.org/10.20491/isarder.2022.1549 |