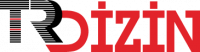
Fleet Type Planning for Private Airline Transportation After Covid-19
Yıl: 2023 Cilt: 11 Sayı: 2 Sayfa Aralığı: 475 - 485 Metin Dili: İngilizce DOI: 10.29109/gujsc.1178375 İndeks Tarihi: 07-07-2023
Fleet Type Planning for Private Airline Transportation After Covid-19
Öz: The global impact of the COVID-19 pandemic has done great damage to air transportation. Demand for airline transportation has declined for reasons such as quarantine practices by countries, curfews, the economic recession, and the transfer of meetings to digital platforms. This situation has also led to a change in individuals' preferences for air transport. The most noticeable change in air transport is the tendency of individuals to use air transport privately to minimize the health risks that face-to-face contact can pose. Individuals who avoid commercial air transport where public transportation is available have shifted to private airline transportation. For these reasons, a forecast study was conducted in this study so that a private airline could provide accurate flight schedules in the future. For the forecast study, the number of aircraft types for 2022 was determined by obtaining data on the number of aircraft by passenger capacity, the number of flights, and the number of passengers for 2019-2021 from the airline company. Support Vector Machines (SVM), Gaussian Process Regression (GPR), Regression Trees, and Ensemble Learning models from machine learning methods were used for the forecasting study. The performance evaluation of the models used was compared. The model results with the highest performance evaluation were used. According to the results obtained, it has been found that there will be an increase of approximately 7% in the number of flights for 2022 compared to the pre- pandemic period. The findings provided important information for the company's future fleet planning.
Anahtar Kelime: Covid-19 Sonrası Özel Havayolu Taşımacılığı İçin Filo Türü Planlaması
Öz: COVID-19 salgınının küresel etkisi hava taşımacılığına büyük zarar vermiştir. Ülkelerin karantina uygulamaları, sokağa çıkma yasakları, ekonomik durgunluk, toplantıların dijital platformlara taşınması gibi nedenlerle havayolu taşımacılığına olan talep azalmıştır. Bu durum bireylerin hava taşımacılığına yönelik tercihlerinin de değişmesine neden olmuştur. Hava taşımacılığındaki en dikkat çekici değişim, bireylerin yüz yüze temasıyla oluşabilecek sağlık risklerini en aza indirmek için hava taşımacılığını özel olarak kullanma eğilimidir. Toplu taşımanın mevcut olduğu yerlerde ticari hava taşımacılığından kaçınan bireyler, özel havayolu taşımacılığına yönelmiştir. Bu nedenlerle, bu çalışmada özel bir havayolu şirketinin gelecekte doğru uçuş programları sağlayabilmesi için bir tahmin çalışması yapılmıştır. Tahmin çalışması için havayolu şirketinden 2019-2021 yılları için yolcu kapasitesine göre uçak sayısı, uçuş sayısı ve yolcu sayısı verileri temin edilerek 2022 yılı için uçak tipi sayısı belirlenmiştir. Tahmin çalışması için makine öğrenmesi yöntemlerinden Destek Vektör Makineleri (DVM), Gauss Süreç Regresyonu (GSR), Regresyon Ağaçları ve Topluluk Öğrenme modelleri kullanılmıştır. Kullanılan modellerin performans değerlendirmesi karşılaştırılmıştır. En yüksek performans değerlendirmesine sahip model sonuçları kullanılmıştır. Elde edilen sonuçlara göre 2022 yılı için uçuş sayılarında pandemi öncesi döneme göre yaklaşık %7'lik bir artış olacağı tespit edilmiştir. Bulgular, şirketin gelecekteki filo planlaması için önemli bilgiler sağlamıştır.
Anahtar Kelime: Belge Türü: Makale Makale Türü: Araştırma Makalesi Erişim Türü: Erişime Açık
- [1] Meriç, S., COVID-19’un Dünya ve Türk sivil havacılık sektörüne ekonomik etkileri. Atlas Journal, 2021. 7(40): p. 1699-1710.
- [2] Adeniran, A. and M. Stephens, The dynamics for evaluating forecasting methods for international air passenger demand in Nigeria. Journal of tourism & hospitality, 2018. 7(4): p. 1-11.
- [3] Atay, M., Y. Eroğlu, and S. Ulusam Seçkiner, Yapay sinir ağları ve adaptif nörobulanık sistemler ile 3. Istanbul havalimanı talep tahmini ve türk hava yolları iç hat filo optimizasyonu. Journal of Industrial Engineering (Turkish Chamber of Mechanical Engineers), 2019. 30(2).
- [4] Efendigil, T. and Ö.E. Eminler, Havacılık sektöründe talep tahminin önemi: yolcu talebi üzerine bir tahmin modeli. Yaşar Üniversitesi E-Dergisi, 2017. 12: p. 14-30.
- [5] Jiang, X., L. Zhang, and X.M. Chen, Short- term forecasting of high-speed rail demand: A hybrid approach combining ensemble empirical mode decomposition and gray support vector machine with real-world applications in China. Transportation Research Part C: Emerging Technologies, 2014. 44: p. 110-127.
- [6] Sun, Y., B. Leng, and W. Guan, A novel wavelet-SVM short-time passenger flow prediction in Beijing subway system. Neurocomputing, 2015. 166: p. 109-121.
- [7] Jafari, N., The chaos on US domestic airline passenger demand forecasting caused by COVID-19. International Journal of Business Forecasting and Marketing Intelligence, 2022. 7(3): p. 241-258.
- [8] Marie-Sainte, S.L., T. Saba, and S. Alotaibi, Air passenger demand forecasting using particle swarm optimization and firefly algorithm. Journal of Computational and Theoretical Nanoscience, 2019. 16(9): p. 3735- 3743.
- [9] Dursun, Ö.O. and S. Toraman, Uzun kısa vadeli bellek yöntemi ile havayolu yolcu tahmini. Journal of Aviation, 2021. 5(2): p. 241-248.
- [10] Pandit, P.K. and M.A. Akhtar Hasin. Business model of aircraft fleet planning using ANN in the road to a digitalized supply chain management: smart and digital solutions for supply chain management. Proceedings of the Hamburg International Conference of Logistics (HICL), Vol. 25. 2018. Berlin: epubli GmbH.
- [11] Wild, G., et al. Machine learning for air transport planning and management. in AIAA AVIATION 2022 Forum. 2022.
- [12] Bao, Y., T. Xiong, and Z. Hu, Forecasting air passenger traffic by support vector machines with ensemble empirical mode decomposition and slope-based method. Discrete Dynamics in Nature and Society, 2012. 2012.
- [13] Guo, X., Y. Grushka-Cockayne, and B. De Reyck, Forecasting airport transfer passenger flow using real-time data and machine learning. Manufacturing & Service Operations Management, 2021.
- [14] Laik, M.N., M. Choy, and P. Sen. Predicting airline passenger load: A case study. in 2014 IEEE 16th Conference on Business Informatics. 2014. IEEE.
- [15] Wilson, A. and R. Adams. Gaussian process kernels for pattern discovery and extrapolation. in International conference on machine learning. 2013. PMLR.
- [16] Mishra, N. and S. Silakari, Predictive analytics: a survey, trends, applications, oppurtunities & challenges. International Journal of Computer Science and Information Technologies, 2012. 3(3): p. 4434-4438.
- [17] Kumar, V. and M. Garg, Predictive analytics: a review of trends and techniques. International Journal of Computer Applications, 2018. 182(1): p. 31-37.
- [18] Indriasari, E., et al. Application of predictive analytics at financial institutions: A systematic literature review. in 2019 8th International Congress on Advanced Applied Informatics (IIAI-AAI). 2019. IEEE.
- [19] Sharma, N., R. Sharma, and N. Jindal, Machine learning and deep learning applications-a vision. Global Transitions Proceedings, 2021. 2(1): p. 24-28.
- [20] Zhang, L., et al., A review of machine learning in building load prediction. Applied Energy, 2021. 285: p. 116452.
- [21] Jordan, M.I. and T.M. Mitchell, Machine learning: Trends, perspectives, and prospects. Science, 2015. 349(6245): p. 255-260.
- [22] Nguyen, G., et al., Machine learning and deep learning frameworks and libraries for large- scale data mining: a survey. Artificial Intelligence Review, 2019. 52(1): p. 77-124.
- [23] Quan, Q., et al., Research on water temperature prediction based on improved support vector regression. Neural Computing and Applications, 2020: p. 1-10.
- [24] Zhang, F. and L.J. O'Donnell, Support vector regression, in Machine Learning. 2020, Elsevier. p. 123-140.
- [25] Çoban, F. and L. Demir, Yapay sinir ağları ve destek vektör regresyonu ile talep tahmini: Gıda işletmesinde bir uygulama. Dokuz Eylül Üniversitesi Mühendislik Fakültesi Fen ve Mühendislik Dergisi, 2021. 23(67): p. 327- 338.
- [26] Dewangan, C.L., S. Singh, and S. Chakrabarti, Combining forecasts of day-ahead solar power. Energy, 2020. 202: p. 117743.
- [27] Fairbrother, J., et al., GaussianProcesses. jl: A Nonparametric Bayes package for the Julia Language. arXiv preprint arXiv:1812.09064, 2018.
- [28] Liu, K., et al., Gaussian process regression with automatic relevance determination kernel for calendar aging prediction of lithium-ion batteries. IEEE Transactions on Industrial Informatics, 2019. 16(6): p. 3767-3777.
- [29] Mehmet, A. and G.A. Doğansoy, Makine öğrenmesi ve derin öğrenme yöntemleri kullanılarak e-perakende sektörüne yönelik talep tahmini. Gazi Üniversitesi Mühendislik Mimarlık Fakültesi Dergisi, 2022. 37(3): p. 1325-1340.
- [30] Zounemat-Kermani, M., et al., Ensemble machine learning paradigms in hydrology: A review. Journal of Hydrology, 2021. 598: p. 126266.
- [31] Zhou, Z.-H., Ensemble methods: foundations and algorithms. 2012: CRC press.
- [32] Kapucu, C. and M. Çubukçu, Fotovoltaik sistemlerde topluluk öğrenmesi temelli hata tespiti. Bilişim Teknolojileri Dergisi, 2019. 12(2): p. 83-91.
- [33] Zulkifly, Z., K.A. Baharin, and C.K. Gan, Improved Machine Learning Model Selection Techniques for Solar Energy Forecasting Applications. International Journal of Renewable Energy Research (IJRER), 2021. 11(1): p. 308-319.
- [34] Gao, K., et al., Julia language in machine learning: Algorithms, applications, and open issues. Computer Science Review, 2020. 37: p. 100254.
- [35] Aguila-Torres, D.S., J.A. Galaviz-Aguilar, and J.R. Cárdenas-Valdez. Reliable comparison for power amplifiers nonlinear behavioral modeling based on regression trees and random forest. in 2022 IEEE International Symposium on Circuits and Systems (ISCAS). 2022. IEEE.
- [36] Chicco, D., M.J. Warrens, and G. Jurman, The coefficient of determination R-squared is more informative than SMAPE, MAE, MAPE, MSE and RMSE in regression analysis evaluation. PeerJ Computer Science, 2021. 7: p. e623.
- [37] Sevinç, A. and K. Buket, Derin öğrenme ve istatistiksel modelleme yöntemiyle sıcaklık tahmini ve karşılaştırılması. Avrupa Bilim ve Teknoloji Dergisi, 2021(28): p. 1222-1228.
- [38] Suau-Sanchez, P., A. Voltes-Dorta, and N. Cugueró-Escofet, An early assessment of the impact of COVID-19 on air transport: Just another crisis or the end of aviation as we know it? Journal of Transport Geography, 2020. 86: p. 102749.
- [39] Wild, P., F. Mathys, and J. Wang, Impact of political and market-based measures on aviation emissions and passenger behaviors (a Swiss case study). Transportation Research Interdisciplinary Perspectives, 2021. 10: p. 100405.
- [40] Zhang, L., et al., The impact of COVID-19 on airline passenger travel behavior: An exploratory analysis on the Chinese aviation market. Journal of Air Transport Management, 2021. 95: p. 102084.
- [41] Ozbilen, B., K.M. Slagle, and G. Akar, Perceived risk of infection while traveling during the COVID-19 pandemic: Insights from Columbus, OH. Transportation Research Interdisciplinary Perspectives, 2021. 10: p. 100326.
- [42] Sobieralski, J.B. and S. Mumbower, Jet-setting during COVID-19: Environmental implications of the pandemic induced private aviation boom. Transportation Research Interdisciplinary Perspectives, 2022. 13: p. 100575.
APA | KILIÇ R, ALBAYRAK ÜNAL Ö, Erkayman B (2023). Fleet Type Planning for Private Airline Transportation After Covid-19. , 475 - 485. 10.29109/gujsc.1178375 |
Chicago | KILIÇ Raziye,ALBAYRAK ÜNAL Özge,Erkayman Burak Fleet Type Planning for Private Airline Transportation After Covid-19. (2023): 475 - 485. 10.29109/gujsc.1178375 |
MLA | KILIÇ Raziye,ALBAYRAK ÜNAL Özge,Erkayman Burak Fleet Type Planning for Private Airline Transportation After Covid-19. , 2023, ss.475 - 485. 10.29109/gujsc.1178375 |
AMA | KILIÇ R,ALBAYRAK ÜNAL Ö,Erkayman B Fleet Type Planning for Private Airline Transportation After Covid-19. . 2023; 475 - 485. 10.29109/gujsc.1178375 |
Vancouver | KILIÇ R,ALBAYRAK ÜNAL Ö,Erkayman B Fleet Type Planning for Private Airline Transportation After Covid-19. . 2023; 475 - 485. 10.29109/gujsc.1178375 |
IEEE | KILIÇ R,ALBAYRAK ÜNAL Ö,Erkayman B "Fleet Type Planning for Private Airline Transportation After Covid-19." , ss.475 - 485, 2023. 10.29109/gujsc.1178375 |
ISNAD | KILIÇ, Raziye vd. "Fleet Type Planning for Private Airline Transportation After Covid-19". (2023), 475-485. https://doi.org/10.29109/gujsc.1178375 |
APA | KILIÇ R, ALBAYRAK ÜNAL Ö, Erkayman B (2023). Fleet Type Planning for Private Airline Transportation After Covid-19. Gazi Üniversitesi Fen Bilimleri Dergisi Part C: Tasarım ve Teknoloji, 11(2), 475 - 485. 10.29109/gujsc.1178375 |
Chicago | KILIÇ Raziye,ALBAYRAK ÜNAL Özge,Erkayman Burak Fleet Type Planning for Private Airline Transportation After Covid-19. Gazi Üniversitesi Fen Bilimleri Dergisi Part C: Tasarım ve Teknoloji 11, no.2 (2023): 475 - 485. 10.29109/gujsc.1178375 |
MLA | KILIÇ Raziye,ALBAYRAK ÜNAL Özge,Erkayman Burak Fleet Type Planning for Private Airline Transportation After Covid-19. Gazi Üniversitesi Fen Bilimleri Dergisi Part C: Tasarım ve Teknoloji, vol.11, no.2, 2023, ss.475 - 485. 10.29109/gujsc.1178375 |
AMA | KILIÇ R,ALBAYRAK ÜNAL Ö,Erkayman B Fleet Type Planning for Private Airline Transportation After Covid-19. Gazi Üniversitesi Fen Bilimleri Dergisi Part C: Tasarım ve Teknoloji. 2023; 11(2): 475 - 485. 10.29109/gujsc.1178375 |
Vancouver | KILIÇ R,ALBAYRAK ÜNAL Ö,Erkayman B Fleet Type Planning for Private Airline Transportation After Covid-19. Gazi Üniversitesi Fen Bilimleri Dergisi Part C: Tasarım ve Teknoloji. 2023; 11(2): 475 - 485. 10.29109/gujsc.1178375 |
IEEE | KILIÇ R,ALBAYRAK ÜNAL Ö,Erkayman B "Fleet Type Planning for Private Airline Transportation After Covid-19." Gazi Üniversitesi Fen Bilimleri Dergisi Part C: Tasarım ve Teknoloji, 11, ss.475 - 485, 2023. 10.29109/gujsc.1178375 |
ISNAD | KILIÇ, Raziye vd. "Fleet Type Planning for Private Airline Transportation After Covid-19". Gazi Üniversitesi Fen Bilimleri Dergisi Part C: Tasarım ve Teknoloji 11/2 (2023), 475-485. https://doi.org/10.29109/gujsc.1178375 |