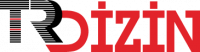
Makine öğrenmesi ve derin öğrenme yöntemleri kullanılarak e-perakende sektörüne yönelik talep tahmini
Yıl: 2022 Cilt: 37 Sayı: 3 Sayfa Aralığı: 1325 - 1340 Metin Dili: Türkçe DOI: 10.17341/gazimmfd.944081 İndeks Tarihi: 29-07-2022
Makine öğrenmesi ve derin öğrenme yöntemleri kullanılarak e-perakende sektörüne yönelik talep tahmini
Öz: Elektronik perakendecilik (e-perakende), perakendeci ile müşterilerin web ve mobil uygulamalar aracılığıyla iletişimde bulunduğu bir perakendecilik çeşididir. E-perakende işletmelerinin artan rekabet ortamında büyümeye devam edebilmesi ve rakip işletmelerden farklılaşarak ön plana çıkabilmesi için değişen tüketici taleplerine zamanında ve doğru bir şekilde cevap verebilmesi gerekmektedir. Ülkemizdeki yerel süpermarket verileri ile e-perakende sektörüne yönelik Makine Öğrenmesi ve Derin Öğrenme algoritmalarını kullanan bir talep tahmin çalışması (bildiğimiz kadarıyla) henüz yapılmamıştır. Bu çalışmada yerel bir süpermarketin son iki yıllık e-ticaret verileri (web sitesi ve mobil uygulama) ve ürün satışını etkileyen faktörler (TÜFE ve yıllık işsizlik oranı) göz önüne alınarak belirli kategorideki ürünlere yönelik talep tahmin edilmiştir. Talep tahmin modeli için altı farklı yapay zekâ algoritmasının (Derin Öğrenme, Yapay Sinir Ağları, Gauss Süreç Regresyonu, Regresyon Ağacı, Destek Vektör Regresyonu ve Ağaç Toplulukları) yirmi dört farklı yöntemi eğitilmiş ve test edilmiştir. Sonuçlar, korelasyon katsayısı (R), Kök Ortalama Kare Hata (KOKH) ve Ortalama Mutlak Hata (OMH) kriterleri ile değerlendirilmiştir.En iyi sonuç Uzun ve Kısa Vadeli Hafıza Ağları kullanılarak alınmıştır (KOKH=0,0353; OMH=0,0164; R=0,9742). Elde edilen sonuçlar ürün tedarikinin doğru miktarlarda yapılmasında, satış kampanyalarında ve pazarlama stratejilerinde kullanılarak e-perakende satışının başarısını artırabilecektir.
Anahtar Kelime: Demand forecasting for e-retail sector using machine learning and deep learning methods
Öz: Electronic retailing (e-retail) is a type of retailing where the retailer and customers communicate via web and mobile applications. In order for e-retail businesses to continue to grow in an increasingly competitive environment and to stand out from competing businesses, they need to be able to respond to changing consumer demands in a timely and accurate manner. A demand forecasting study using Machine Learning and Deep Learning algorithms for the e-retail sector with local supermarkets' data in Turkey (as far as we know) has not been carried out yet. In this study, the demand for a certain category of products was estimated by considering the two-year e-commerce data (website and mobile application) of a local supermarket for the years 2019-2020 and the factors affecting product sales (holidays, CPI value and unemployment rate). Twenty-four different methods of six different artificial intelligence algorithms (Deep Learning, Artificial Neural Networks, Gaussian Process Regression, Regression Tree, Support Vector Regression and Ensemble Learning) were used to obtain the best demand forecasting model. The obtained results were evaluated using correlation coefficient (R), Root Mean Square Error (RMSE), and Mean Absolute Error (MAE) criteria. The best result was obtained using Long Short-Term Memory Networks (RMSE= 0.0353; MAE= 0.0164; R = 0.9742). The results obtained will be able to increase the success of e-retail sales by using the product supply in the right quantities, in sales campaigns, and in marketing strategies.
Anahtar Kelime: Belge Türü: Makale Makale Türü: Araştırma Makalesi Erişim Türü: Erişime Açık
- 1. Öztürk, İ., Türkiye’de perakende sektörü, Journal of Social Sciences, 3 (1), 69-81, 2006.
- 2. Tevetoğlu, M., Kel, H.A., COVID-19 pandemisi, dijital perakendecilik ve sözleşme hukukuna etkisi, Yapay Zeka Çağında Hukuk, 1 (1), 66-78, 2021.
- 3. Yücesoy, M. Temizlik kağıtları sektöründe yapay sinir ağları ile talep tahmini, İstanbul Teknik Üniversitesi, Fen Bilimleri Enstitüsü, İstanbul, 2011.
- 4. Torun H., Canbulut G., Analysis of two stage supply chain coordination under fuzzy demand, Journal of the Faculty of Engineering and Architecture of Gazi University, 34 (3), 1315-1328, 2019.
- 5. Ünlü, R., Zaman serileri tahminlenmesinde makine öğrenimi ve derin öğrenme tekniklerinin kıyaslanması: Türkiye elektirik üretimi için en iyi tahmin modelinin seçilmesine yönelik bir vaka çalışması, Süleyman Demirel Üniversitesi Fen Bilimleri Enstitüsü Dergisi, 23 (2), 359-370, 2019.
- 6. Rahman, S., Rabiul Alam, M.G., Mahbubur Rahman, M., Deep Learning Based Ensemble Method for Household Energy Demand Forecasting of Smart Home, International Conference on Computer and Information Technology, Dakka-Bangladeş, 18-20 Aralık, 19472446, 2019.
- 7. Es H., Kalender Y.F., Hamzaçebi C., Forecasting the net energy demand of Turkey by artificial neural networks, Journal of the Faculty of Engineering and Architecture of Gazi University, 29 (3), 495-504, 2014.
- 8. Sönmez, O., Zengin, K., Yiyecek ve içecek işletmelerinde talep tahmini: yapay sinir ağları ve regresyon yöntemleriyle bir karşılaştırma, European Journal of Science and Technology, Özel Sayı, 302-308, 2019.
- 9. Yergök, D., Acı, M., An alternative approach for daily demand prediction in mass meal production: Regression Learner, European Journal of Science and Technology, Özel Sayı, 64-73, 2019.
- 10. Huber, J., Stuckenschmidt, H., Daily retail demand forecasting using machine learning with emphasis on calendric special days, International Journal of Forecasting, 36 (4), 1420-1438, 2020.
- 11. Satoglu, S.I., Eren, U., Perakende Giyim Sektöründe Yapay Sinir Ağları ile Talep Tahmini, Endüstri ve İşletme Mühendisliği Kurultayı, İstanbul-Türkiye, 1-7, 17-18 Kasım, 2017.
- 12. Arslankaya, S., Bir Lojistik Firmasında Zaman Serileri Analizi ve Yapay Sinir Ağları ile Talep Tahminin Karşılaştırılması, International Symposium on Innovative Approaches in Engineering and Natural Sciences, 239-245, 22-24 Kasım, 2019.
- 13. Kaya, K.S., Yıldırım, Ö., A prediction model for automobile sales in turkey using deep neural network, Endüstri Mühendisliği, 31 (1), 57-74, 2020.
- 14. Türk, E., Kiani, F., Yapay sinir ağları ile talep tahmini yapma: Beyaz eşya üretim planlaması için YSA uygulaması, İstanbul Sabahattin Zaim Üniversitesi Fen Bilimleri Enstitüsü Dergisi, 1 (1), 30-37, 2019.
- 15. Law, R., Li, G., Fong, D.K.C., Han, X., Tourism demand forecasting: A deep learning approach, Annals of Tourism Research, 75, 410-423, 2019.
- 16. Claveria, O., Monte, E., Torra, S., Regional tourism demand forecasting with machine learning models: Gaussian process regression vs. neural network models in a multiple-input multiple-output setting, SSRN Electronic Journal, 1 (1), 1-26, 2018.
- 17. Xu, C., Ji, J., Liu, P., The station-free sharing bike demand forecasting with a deep learning approach and large-scale datasets, Transportation Research Part C: Emerging Technologies, 95, 47-60, 2018.
- 18. Jiang, S., Chin, K.S., Wang, L., Qu, G., Tsui, K.L., Modified genetic algorithm-based feature selection combined with pre-trained deep neural network for demand forecasting in outpatient department, Expert Systems with Applications, 82, 216-230, 2017.
- 19. Shi, H., Wang, H., Huang, Y., Zhao, L., Qin, C., Liu, C., A hierarchical method based on weighted extreme gradient boosting in ECG heartbeat classification, Computer Methods and Programs in Biomedicine, 171, 1-10, 2019.
- 20. Du, X.F., Leung, S.C.H., Zhang, J.L., Lai, K.K., Demand forecasting of perishable farm products using support vector machine, International Journal of Systems Science, 44 (3), 556-567, 2013.
- 21. Slimani, I., El Farissi, I., Achchab, S., Artificial Neural Networks for Demand Forecasting: Application Using Moroccan Supermarket Data, International Conference on Intelligent Systems Design and Applications, PortoPortekiz, 266-271, 14-16 Kasım, 2016.
- 22. Qu, T., Zhang, J.H., Chan, F.T.S., Srivastava, R.S., Tiwari, M.K., Park, W.Y., Demand prediction and price optimization for semi-luxury supermarket segment, Computers and Industrial Engineering, 113, 91-102, 2017.
- 23. Neelakantam, G., Onthoni, D.D., Sahoo, P.K., Fog computing enabled locality based product demand prediction and decision making using reinforcement learning, Electronics, 10 (3), 1-16, 2021.
- 24. Bellendorf, J., Mann, Z.Á., Classification of optimization problems in fog computing, Future Generation Computer Systems, 107, 158-176, 2020.
- 25. Kaggle Supermarket sales, https://www.kaggle.com/aungpyaeap/supermarketsales, Yayın tarihi Mayıs 27, 2019. Erişim tarihi Aralık 12, 2021.
- 26. Wolters, J., Huchzermeier, A., Joint in-season and outof-season promotion demand forecasting in a retail environment, Journal of Retailing, Baskıda, DOI: 10.1016/j.jretai.2021.01.003, 2022.
- 27. BigMart Sales Data, https://www.kaggle.com/brijbhushannanda1979/bigma rt-sales-data, Yayın tarihi Ocak 16, 2018. Erişim tarihi Aralık 12, 2021.
- 28. Malik, N., Singh, K., Sales prediction model for Big Mart, Parichay: Maharaja Surajmal Institute Journal of Applied Research, 3 (1), 22-32, 2020.
- 29. Sekban, J., Applying machine learning algorithms in sales prediction, Yüksek Lisans Tezi, Kadir Has Universitesi, Fen Bilimleri Enstitüsü, İstanbul, 2019.
- 30. Agbonlahor, O.V., A comparative study on machine learning and deep learning techniques for predicting big Mart item outlet sales, Yüksek Lisans Tezi, Dublin Business School, Dublin, 2020.
- 31. Punam, K., Pamula, R., Jain, P.K., A Two-Level Statistical Model for Big Mart Sales Prediction, International Conference on Computing, Power and Communication Technologies, Yeni Delhi-Hindistan, 617-620, 28-29 Eylül, 2018.
- 32. Çelik, Ş., Şengül, T., Şengül, Y.A., İnci, H., Tüketici fiyat indeksini etkileyen bitkisel ve hayvansal üretim değerlerinin çok değişkenli uyarlanabilir regresyon uzanımları ile incelenmesi: Türkiye örneği, Journal of Awareness, 3 (5), 399-408, 2018.
- 33. Uysal, D., Erdoğan, S., Enflasyon ile İşsizlik Oranı Arasındaki İlişki ve Türkiye Örneği (1980-2002), Sosyal Ekonomik Araştırmalar Dergisi, 3 (6), 35-46, 2003.
- 34. Graupe, D., Principles of Artificial Neural Networks, Editör: Chen, W.K., World Scientific Publishing, Hackensack, NJ, A.B.D., 3, 9-17, 2013.
- 35. Razavi, S., Tolson, B.A., A new formulation for feedforward neural networks, IEEE Transactions on Neural Networks, 22 (10), 1588-1598, 2011.
- 36. MATLAB Function fitting neural network, https://www.mathworks.com/help/deeplearning/ref/fitn et.html, Yayın tarihi Mart 13, 2021. Erişim tarihi Aralık 12, 2021.
- 37. Alkhasawneh, M.S., Tay, L.T., A hybrid intelligent system integrating the cascade forward neural network with elman neural network, Arabian Journal for Science and Engineering, 43 (12), 6737-6749, 2018.
- 38. Öztemel, E., Yapay Sinir Ağları, Papatya Bilim Yayınevi, İstanbul, Türkiye, 59-74, 2012.
- 39. Şeker, A., Diri, B., Balık, H.H., A review about deep learning methods and applications, Gazi Journal of Engineering Sciences, 3 (3), 47-64, 2017.
- 40. Babu, G.S., Zhao, P., Li, X.L., Deep convolutional neural network based regression approach for estimation of remaining useful life, International Conference on Database Systems for Advanced Applications, DallasA.B.D., 214-228, 16-18 Nisan, 2016.
- 41. Goodfellow, I., Bengio, Y., Courville, A., Deep Learning, Editör: Dietterich, T., The MIT Press, Massachusetts, A.B.D., 2016.
- 42. Şişmanoğlu, G., Koçer, F., Önde, M.A., Şahingöz, O.K., Derin öğrenme yöntemleri ile borsada fiyat tahmini, Bitlis Eren Üniversitesi Fen Bilimleri Dergisi, 9 (1), 434-445, 2020.
- 43. Sherratt, F., Plummer, A., Iravani, P., Understanding LSTM network behaviour of imu-based locomotion mode recognition for applications in prostheses and wearables, Sensors, 21 (4), 1-23, 2021.
- 44. Şeker, A., Yüksek, A.G., Stacked autoencoder method for fabric defect detection, Cumhuriyet Science Journal, 38 (2), 342-342, 2017.
- 45. Liu, K., Hu, X., Wei, Z., Li, Y., Jiang, Y., Modified gaussian process regression models for cyclic capacity prediction of lithium-ion batteries, IEEE Transactions on Transportation Electrification, 5 (4), 1225-1236, 2019.
- 46. Fang, D., Zhang, X., Yu, Q., Jin, T.C., Tian, L., A novel method for carbon dioxide emission forecasting based on improved Gaussian processes regression, Journal of Cleaner Production, 173, 143-150, 2018.
- 47. Zhao, J., Guo, H., Han, M., Tang, H., Li, X., Gaussian process regression for prediction of sulfate content in lakes of China, Journal of Engineering and Technological Sciences, 51 (2), 198-215, 2019.
- 48. MATLAB, Choose Regression Model Options, https://www.mathworks.com/help/stats/choose regression-model-options.html, Yayın tarihi Mart 15, 2018. Erişim tarihi Aralık 12, 2021.
- 49. Pisner, D.A., Schnyer, D.M., Support Vector Machine, Machine Learning: Methods and Applications to Brain Disorders, Cilt 7, Editör: Mechelli, A., Academic Press, Londra, İngiltere, 101-121, 2019.
- 50. Awad, M., Khanna, R., Awad, M., Khanna, R., Support Vector Regression, Efficient Learning Machines, Apress, 4, 67-80, 2015.
- 51. Onan, A., A clustering based classifier ensemble approach to corporate bankruptcy prediction, Alphanumeric Journal, 6 (2), 365-376, 2018.
- 52. Yılmaz, H., Random forests yönteminde kayıp veri probleminin incelenmesi ve sağlık alanında bir uygulama, Yüksek Lisans Tezi, Eskişehir Osmangazi Üniversitesi, Sağlık Bilimleri Enstitüsü, Eskişehir, 2014.
- 53. Mosavi, A., Sajedi Hosseini, F., Choubin, B., Goodarzi, M., Dineva, A.A., Rafiei Sardooi, E., Ensemble boosting and bagging based machine learning models for groundwater potential prediction, Water Resources Management, 35 (1), 23-37, 2021.
- 54. Machine Learning with MATLAB, https://www.mathworks.com/solutions/machinelearning.html, Yayın tarihi Mart 15, 2018. Erişim tarihi Aralık 12, 2021.
- 55. Chai, T., Draxler, R.R., Root mean square error (RMSE) or mean absolute error (MAE)? Arguments against avoiding RMSE in the literature, Geoscientific Model Development, 7 (3), 1247-1250, 2014.
- 56. Cleophas, T.J., Zwinderman, A.H., Bayesian Pearson Correlation Analysis, Modern Bayesian Statistics in Clinical Research, Cilt 11, Springer International Publishing, Cham, İsviçre, 111-118, 2018.
- 57. Asuero, A.G., Sayago, A., González, A.G., The correlation coefficient: An overview, Critical Reviews in Analytical Chemistry, 36 (1), 41-59, 2007.
- 58. Karayiğit, H., Acı, Ç.İ., Akdağlı, A., Detecting abusive Instagram comments in Turkish using convolutional neural network and machine learning methods, Expert Systems with Applications, 174 (1), 114802, 2021.
APA | ACI M, Ayyıldız Doğansoy G (2022). Makine öğrenmesi ve derin öğrenme yöntemleri kullanılarak e-perakende sektörüne yönelik talep tahmini. , 1325 - 1340. 10.17341/gazimmfd.944081 |
Chicago | ACI Mehmet,Ayyıldız Doğansoy Gamze Makine öğrenmesi ve derin öğrenme yöntemleri kullanılarak e-perakende sektörüne yönelik talep tahmini. (2022): 1325 - 1340. 10.17341/gazimmfd.944081 |
MLA | ACI Mehmet,Ayyıldız Doğansoy Gamze Makine öğrenmesi ve derin öğrenme yöntemleri kullanılarak e-perakende sektörüne yönelik talep tahmini. , 2022, ss.1325 - 1340. 10.17341/gazimmfd.944081 |
AMA | ACI M,Ayyıldız Doğansoy G Makine öğrenmesi ve derin öğrenme yöntemleri kullanılarak e-perakende sektörüne yönelik talep tahmini. . 2022; 1325 - 1340. 10.17341/gazimmfd.944081 |
Vancouver | ACI M,Ayyıldız Doğansoy G Makine öğrenmesi ve derin öğrenme yöntemleri kullanılarak e-perakende sektörüne yönelik talep tahmini. . 2022; 1325 - 1340. 10.17341/gazimmfd.944081 |
IEEE | ACI M,Ayyıldız Doğansoy G "Makine öğrenmesi ve derin öğrenme yöntemleri kullanılarak e-perakende sektörüne yönelik talep tahmini." , ss.1325 - 1340, 2022. 10.17341/gazimmfd.944081 |
ISNAD | ACI, Mehmet - Ayyıldız Doğansoy, Gamze. "Makine öğrenmesi ve derin öğrenme yöntemleri kullanılarak e-perakende sektörüne yönelik talep tahmini". (2022), 1325-1340. https://doi.org/10.17341/gazimmfd.944081 |
APA | ACI M, Ayyıldız Doğansoy G (2022). Makine öğrenmesi ve derin öğrenme yöntemleri kullanılarak e-perakende sektörüne yönelik talep tahmini. Gazi Üniversitesi Mühendislik Mimarlık Fakültesi Dergisi, 37(3), 1325 - 1340. 10.17341/gazimmfd.944081 |
Chicago | ACI Mehmet,Ayyıldız Doğansoy Gamze Makine öğrenmesi ve derin öğrenme yöntemleri kullanılarak e-perakende sektörüne yönelik talep tahmini. Gazi Üniversitesi Mühendislik Mimarlık Fakültesi Dergisi 37, no.3 (2022): 1325 - 1340. 10.17341/gazimmfd.944081 |
MLA | ACI Mehmet,Ayyıldız Doğansoy Gamze Makine öğrenmesi ve derin öğrenme yöntemleri kullanılarak e-perakende sektörüne yönelik talep tahmini. Gazi Üniversitesi Mühendislik Mimarlık Fakültesi Dergisi, vol.37, no.3, 2022, ss.1325 - 1340. 10.17341/gazimmfd.944081 |
AMA | ACI M,Ayyıldız Doğansoy G Makine öğrenmesi ve derin öğrenme yöntemleri kullanılarak e-perakende sektörüne yönelik talep tahmini. Gazi Üniversitesi Mühendislik Mimarlık Fakültesi Dergisi. 2022; 37(3): 1325 - 1340. 10.17341/gazimmfd.944081 |
Vancouver | ACI M,Ayyıldız Doğansoy G Makine öğrenmesi ve derin öğrenme yöntemleri kullanılarak e-perakende sektörüne yönelik talep tahmini. Gazi Üniversitesi Mühendislik Mimarlık Fakültesi Dergisi. 2022; 37(3): 1325 - 1340. 10.17341/gazimmfd.944081 |
IEEE | ACI M,Ayyıldız Doğansoy G "Makine öğrenmesi ve derin öğrenme yöntemleri kullanılarak e-perakende sektörüne yönelik talep tahmini." Gazi Üniversitesi Mühendislik Mimarlık Fakültesi Dergisi, 37, ss.1325 - 1340, 2022. 10.17341/gazimmfd.944081 |
ISNAD | ACI, Mehmet - Ayyıldız Doğansoy, Gamze. "Makine öğrenmesi ve derin öğrenme yöntemleri kullanılarak e-perakende sektörüne yönelik talep tahmini". Gazi Üniversitesi Mühendislik Mimarlık Fakültesi Dergisi 37/3 (2022), 1325-1340. https://doi.org/10.17341/gazimmfd.944081 |