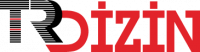
Spektral ayrıştırma ve komşu piksel ilişkisi temelli hiperspektral ve multispektral görüntülerin kaynaştırılması
Yıl: 2023 Cilt: 38 Sayı: 4 Sayfa Aralığı: 2385 - 2396 Metin Dili: Türkçe DOI: 10.17341/gazimmfd.915691 İndeks Tarihi: 29-09-2023
Spektral ayrıştırma ve komşu piksel ilişkisi temelli hiperspektral ve multispektral görüntülerin kaynaştırılması
Öz: Hiperspektral (HS) görüntüler yüksek spektral çözünürlüğe sahip oldukları halde, teknolojik kısıtlamalardan dolayı uzamsal çözünürlükleri düşüktür. Sınıflandırma başarımının arttırılması veya daha detaylı içerik elde edilmesi gibi gereksinimlerin karşılanabilmesi için bu tip görüntülerin yüksek spektral çözünürlük yanında yüksek uzamsal çözünürlüğe de sahip olmaları faydalıdır. Bu nedenle, HS görüntülerin Multispektral (MS) görüntüler ile kaynaştırılması son yıllarda popülerliğini koruyan bir konu olarak çalışılmaktadır. Literatürde Matris Ayrıştırması (MA) temelli görüntü kaynaştırmasında, komşu piksellerin etkisini dikkate alan bir çalışma ile karşılaşılmamıştır. Bu nedenle, bu çalışmada spektral ayrıştırma ve uzamsal komşuluk etkisini dikkate alan yeni bir kaynaştırma yaklaşımı önerilmektedir. Öncelikle, spektral ayrıştırma kullanılarak hiperspektral görüntüden son eleman ve katışım oranları çıkarılmaktadır. Çıkarılan son elemalar, multispektral görüntü algılayıcısının spektral yanıtı kullanılarak multispektral spektral çözünürlüğüne taşınmaktadır. Daha sonra, multispektral görüntüdeki her bir piksel için, son elemanlar ile komşuluk piksellerinin katışım oranları kestirilmektedir. Son olarak, multispektral görüntüden kestirilen katışım haritası ile hiperspektral görüntünün son elemanları ve komşuluk pikselleri kullanılarak hem uzamsal hem de spektral çözünürlüğü yüksek görüntü elde edilmektedir. Önerilen kaynaştırma yöntemi gerçek hiperspektral görüntüler üzerinde test edilmiştir ve deneysel çalışmalar literatürdeki çalışmalara göre başarımının daha yüksek olduğunu göstermektedir.
Anahtar Kelime: Hyperspectral and multispectral image fusion based on spectral decomposition and neighborhood pixel relation
Öz: Hyperspectral (HS) images have high spectral resolution, but their spatial resolution is low due to technological constraints. It is beneficial to have HS images that have high spatial resolution as well as high spectral resolution to increase classification accuracy or obtain more detailed content in this kind of image. Thus, HS and multispectral (MS) image fusion have become a very popular topic in recent years. In this study, spectral decomposition and neighborhood pixel relation-based hyperspectral and multispectral image fusion is proposed. Firstly, spectral decomposition is used to get endmembers and their abundance maps from the hyperspectral image. Hyperspectral endmembers are degraded to multispectral spectral resolution according to the spectral response model of the multispectral sensor. Then, abundance fractions of the endmembers and neighborhood pixels are estimated for each multispectral pixel. Finally, the abundance map of multispectral image and endmembers, and neighborhood pixels of the hyperspectral image are used to obtain an image with high spatial and spectral resolution. The proposed method is tested on real hyperspectral images and experimental results show that it gives the highest accuracy compared to studies in the literature.
Anahtar Kelime: Belge Türü: Makale Makale Türü: Araştırma Makalesi Erişim Türü: Erişime Açık
- 1. Hanbay K., Hyperspectral image classification using convolutional neural network and twodimensional complex Gabor transform, Journal of the Faculty of Engineering and Architecture of Gazi University, 35 (1), 443–456, 2020.
- 2. Souza Jr,C., Firestone L., Silva L.M., Roberts D., Mapping forest degradation in the Eastern Amazon from SPOT 4 through spectral mixture models, Remote Sens. Environment, 87 (4), 494–506, 2003.
- 3. Licciardi G.A., Villa A., Khan M.M., Chanussot J., Image fusion and spectral unmixing of hyperspectral images for spatial improvement of classification maps, IEEE Int. Conf. Geosci. Remote Sens. (IGARSS), Munich, Germany, 7290–729, 22-27 July 2012.
- 4. Simoes M., Bioucas Dias J., Almeida L., Chanussot J., A convex formulation for hyperspectral image superresolution via subspace-based regularization, IEEE Trans. Geosci. and Remote Sens., 53 (6), 3373- 3388, 2015.
- 5. Wei Q., Bioucas Dias J.M., Dobigeon N., Tourneret J.Y., Hyperspectral and multispectral image fusion based on a sparse representation, IEEE Trans. Geosci. and Remote Sens., 53 (7), 3658– 3668, Sept. 2015.
- 6. Liu J.G. Smoothing Filter-based Intensity Modulation: A spectral preserve image fusion technique for improving spatial details, International Journal of Remote Sensing, 21 (18), 3461–3472, Jan. 2000.
- 7. Laben C.A., Brower B. V., Process for enhancing the spatial resolution of multispectral imagery using pan-sharpening, Patent US 6 011 875 A, 2000.
- 8. Yokoya N., Yairi T., Iwasaki A., Coupled nonnegative matrix factorization unmixing for hyperspectral and multispectral data fusion, IEEE Trans. Geosci. Remote Sens., 50 (2), 528–537, Feb. 2012.
- 9. Li S., Dian R., Fang L., Bioucas-Dias J.M., Fusing hyperspectral and multispectral images via coupled sparse tensor factorization, IEEE Transactions on Image Processing, 27 (8), 4118–4130, 2018.
- 10. Eismann M.T., Resolution enhancement of hyperspectral imagery using maximum a posteriori estimation with a stochastic mixing model, IEEE Workshop on Advances in Techniques for Analysis of Remotely Sensed Data, Greenbelt, MD, USA, 282-289, 27-28 October 2003.
- 11. Thomas C., Ranchin T., Wald L., Chanussot J., Synthesis of multispectral images to high spatial resolution: a critical review of fusion methods based on remote sensing physics, IEEE Trans. Geosci. And Remote Sens., 46 (5), 1301–1312, May 2008.
- 12. Yokoya N., Grohnfeldt C., Chanussot J., Hyperspectral and Multispectral Data Fusion: A comparative review of the recent literature, IEEE Geoscience and Remote Sensing, 5 (2), 20-56, June. 2017.
- 13. Aiazzi B., Alparone L., Baronti S., Garzelli A., Selva M., MTFtailored multiscale fusion of high-resolution MS and Pan imagery, Photogramm. Eng. Remote Sens., 72 (5), 591–596, May 2006.
- 14. Selva M., Aiazzi B., Butera F., Chiarantini L., Baronti S., Hypersharpening: A first approach on SIM-GA data, IEEE J. Sel. Topics Appl. Earth Observ. Remote Sens., 8 (6), 3008–3024, Jun. 2015.
- 15. Liao W., Huang X., Coillie F., Gautama S., Pizurica A., Philips W., Liu H., Zhu T., Shimoni M., Moser G., Tuia D., Processing of multiresolution thermal hyperspectral and digital color data: Outcome of the 2014 IEEE GRSS data fusion contest, IEEE J. Sel. Topics Appl. Earth Observ. Remote Sens., 8 (6), 2984-2996, June 2015.
- 16. Wei Q., Dobigeon N., Tourneret J.Y., Fast fusion of multi-band images based on solving a Sylvester equation, IEEE Trans. Image Process., 24 (11), 4109–4121, Nov. 2015.
- 17. Akhtar N., Shafait F., Mian A., Sparse spatio-spectral representation for hyperspectral image super-resolution, European Conference on Computer Vision (ECCV), Zurich, Switzerland, 63–78, 6-12 September, 2014.
- 18. Lanaras C., Baltsavias E., Schindler K., Hyperspectral superresolution by coupled spectral unmixing, in Proc. IEEE International Conference on Computer Vision (ICCV), Santiago, Chile, 3586–3594, 07-13 December, 2015.
- 19. Alparone L., Wald L., Chanussot J., Thomas C., Gamba P., Bruce L.M., Comparison of pan sharpening algorithms: Outcome of the 2006 GRSS data fusion contest, IEEE Trans. Geosci. Remote Sens., 45 (10), 3012- 3021, Oct. 2007.
- 20. Du Q., Younan N., King R., Shah V., On the performance evaluation of pan-sharpening techniques, IEEE Geosci. Remote Sens. Lett., 4 (4), 518-522, Oct. 2007.
- 21. Chang C.I., Du Q., Estimation of number of spectrally distinct signal sources in hyperspectral imagery, IEEE Trans. on Geoscience and Remote Sensing, 42 (3), 608-619, March 2004.
- 22. Irmak H., Akar G.B., Yuksel S.E., A MAP-Based Approach for Hyperspectral Imagery Super-Resolution, IEEE Transactions on Image Processing, 27 (6), 2942-2951, June 2018.
- 23. Irmak H., Akar G.B., Yuksel S.E., Fusion based resolution enhancement in hyperspectral images, 25th Signal Processing and Communications Applications Conference (SIU), Antalya-Turkey, 1-4, 15-18 May, 2017.
- 24. Bioucas-Dias J., Nascimento J., Hyperspectral subspace identification, in IEEE Transactions on Geoscience and Remote Sensing, 46 (8), 2435- 2445, 2005.
- 25. Nascimento J., Bioucas-Dias J., Vertex component analysis: a fast algorithm to unmix hyperspectral data, in IEEE Transactions on Geoscience and Remote Sensing, 43 (8), 898-910, 2005.
- 26. Heinz D., Chang C.-I., Fully constrained least squares linear mixture analysis for material quantification in hyperspectral imagery, IEEE Trans. on Geoscience and Remote Sensing, 39 (3), 529-545, March 2001.
- 27. He K., Sun J., Tang X., Guided image filtering, IEEE Trans. Patt. Anal. Mach. Intell., 35 (6), 1397–1409, 2013.
- 28. Baumgardner M.F., Biehl L.L., Landgrebe D.A., 220 band aviris hyperspectral image data set: June 12, 1992 indian pine test site 3, Purdue University Research Repository, Sep 2015.
- 29. Computational Intelligence Group. Hyperspectral Remote Sensing Scenes. https://www.ehu.eus/ccwintco/index.php/Hyperspectral_Remote_Sensi ng_Scenes. Yayın tarihi Mayıs 20, 2011. Güncelleme tarihi Temmuz 12, 2021. Erişim tarihi Nisan 10, 2021.
- 30. Fauvel M., Benediktsson J.A., Chanussot J., Sveinsson J.R., Spectral and spatial classification of hyperspectral data using svms and morphological profiles. IEEE Trans. on Geoscience and Remote Sensing 46 (11), 3804–3814, 2008.
- 31. Li J., Bioucas-Dias J.M., Plaza A., Semisupervised hyperspectral image segmentation using multinomial logistic regression with active learning. IEEE Trans. on Geoscience and Remote Sensing 48 (11), 4085–4098, 2010.
- 32. Yokoya N., Iwasaki A., Airborne hyperspectral data over Chikusei, Space Appl. Lab., Univ. Tokyo, Japan, Tech. Rep. SAL-2016-05-27, May 2016.
- 33. Wald L., Ranchin T., Mangolini M., Fusion of satellite images of different spatial resolutions: Assessing the quality of resulting image, Photogrammetric Engineering and Remote Sensing, 63 (6), 691-699, 1997.
- 34. Roberta H.Y., Goetz A.F.H., Boardman J.W., Discrimination Among Semi-Arid Landscape Endmembers Using the Spectral Angle Mapper (SAM) Algorithm, Summaries of the 4 th JPL Airborne Earth Science Workshop, California-USA, 1, 147-149, 1992.
- 35. Wald L., Assessing the Quality of Synthesized Images, Data Fusion Definitions and Architectures Fusion of images of different spatial resolutions. Les Presses de l’Ecole des Mines, Paris, France, 159-163, 2002.
- 36. Eskicioglu A.M., Fisher P.S., Image quality measures and their performance, IEEE Transactions on Communications, 43 (12), 2959- 2965, 1995.
- 37. Garzelli A., Nencini F., Hypercomplex quality assessment of multi/hyperspectral images, IEEE Geosci. Remote Sens. Lett., 6 (4), 662–665, October 2009.
- 38. Çatalbaş M.C., Gülten A., A novel super-resolution approach for computed tomography images by inverse distance weighting method, Journal of the Faculty of Engineering and Architecture of Gazi University, 33 (2), 671–684, 2018.
- 39. Licciardi G.A., Veganzones M. A., Simoes M., Bioucas J., Chanussot J., Super-resolution of hyperspectral images using local spectral unmixing, IEEE Workshop on Hyperspectral Image and Signal Processing: Evolution in Remote Sensing (WHISPERS), Lausanne, Switzerland, 1-4, 24-27 June, 2014.
- 40. Mei S., He M., Wang Z., Feng D., Spatial Purity Based Endmember Extraction for Spectral Mixture Analysis, IEEE Transactions on Geoscience and Remote Sensing, 48 (9), 3434-3445, 2010.
APA | ÇEŞMECİ D, Urhan O, GÜLLÜ M (2023). Spektral ayrıştırma ve komşu piksel ilişkisi temelli hiperspektral ve multispektral görüntülerin kaynaştırılması. , 2385 - 2396. 10.17341/gazimmfd.915691 |
Chicago | ÇEŞMECİ DAVUT,Urhan Oğuzhan,GÜLLÜ MEHMET KEMAL Spektral ayrıştırma ve komşu piksel ilişkisi temelli hiperspektral ve multispektral görüntülerin kaynaştırılması. (2023): 2385 - 2396. 10.17341/gazimmfd.915691 |
MLA | ÇEŞMECİ DAVUT,Urhan Oğuzhan,GÜLLÜ MEHMET KEMAL Spektral ayrıştırma ve komşu piksel ilişkisi temelli hiperspektral ve multispektral görüntülerin kaynaştırılması. , 2023, ss.2385 - 2396. 10.17341/gazimmfd.915691 |
AMA | ÇEŞMECİ D,Urhan O,GÜLLÜ M Spektral ayrıştırma ve komşu piksel ilişkisi temelli hiperspektral ve multispektral görüntülerin kaynaştırılması. . 2023; 2385 - 2396. 10.17341/gazimmfd.915691 |
Vancouver | ÇEŞMECİ D,Urhan O,GÜLLÜ M Spektral ayrıştırma ve komşu piksel ilişkisi temelli hiperspektral ve multispektral görüntülerin kaynaştırılması. . 2023; 2385 - 2396. 10.17341/gazimmfd.915691 |
IEEE | ÇEŞMECİ D,Urhan O,GÜLLÜ M "Spektral ayrıştırma ve komşu piksel ilişkisi temelli hiperspektral ve multispektral görüntülerin kaynaştırılması." , ss.2385 - 2396, 2023. 10.17341/gazimmfd.915691 |
ISNAD | ÇEŞMECİ, DAVUT vd. "Spektral ayrıştırma ve komşu piksel ilişkisi temelli hiperspektral ve multispektral görüntülerin kaynaştırılması". (2023), 2385-2396. https://doi.org/10.17341/gazimmfd.915691 |
APA | ÇEŞMECİ D, Urhan O, GÜLLÜ M (2023). Spektral ayrıştırma ve komşu piksel ilişkisi temelli hiperspektral ve multispektral görüntülerin kaynaştırılması. Gazi Üniversitesi Mühendislik Mimarlık Fakültesi Dergisi, 38(4), 2385 - 2396. 10.17341/gazimmfd.915691 |
Chicago | ÇEŞMECİ DAVUT,Urhan Oğuzhan,GÜLLÜ MEHMET KEMAL Spektral ayrıştırma ve komşu piksel ilişkisi temelli hiperspektral ve multispektral görüntülerin kaynaştırılması. Gazi Üniversitesi Mühendislik Mimarlık Fakültesi Dergisi 38, no.4 (2023): 2385 - 2396. 10.17341/gazimmfd.915691 |
MLA | ÇEŞMECİ DAVUT,Urhan Oğuzhan,GÜLLÜ MEHMET KEMAL Spektral ayrıştırma ve komşu piksel ilişkisi temelli hiperspektral ve multispektral görüntülerin kaynaştırılması. Gazi Üniversitesi Mühendislik Mimarlık Fakültesi Dergisi, vol.38, no.4, 2023, ss.2385 - 2396. 10.17341/gazimmfd.915691 |
AMA | ÇEŞMECİ D,Urhan O,GÜLLÜ M Spektral ayrıştırma ve komşu piksel ilişkisi temelli hiperspektral ve multispektral görüntülerin kaynaştırılması. Gazi Üniversitesi Mühendislik Mimarlık Fakültesi Dergisi. 2023; 38(4): 2385 - 2396. 10.17341/gazimmfd.915691 |
Vancouver | ÇEŞMECİ D,Urhan O,GÜLLÜ M Spektral ayrıştırma ve komşu piksel ilişkisi temelli hiperspektral ve multispektral görüntülerin kaynaştırılması. Gazi Üniversitesi Mühendislik Mimarlık Fakültesi Dergisi. 2023; 38(4): 2385 - 2396. 10.17341/gazimmfd.915691 |
IEEE | ÇEŞMECİ D,Urhan O,GÜLLÜ M "Spektral ayrıştırma ve komşu piksel ilişkisi temelli hiperspektral ve multispektral görüntülerin kaynaştırılması." Gazi Üniversitesi Mühendislik Mimarlık Fakültesi Dergisi, 38, ss.2385 - 2396, 2023. 10.17341/gazimmfd.915691 |
ISNAD | ÇEŞMECİ, DAVUT vd. "Spektral ayrıştırma ve komşu piksel ilişkisi temelli hiperspektral ve multispektral görüntülerin kaynaştırılması". Gazi Üniversitesi Mühendislik Mimarlık Fakültesi Dergisi 38/4 (2023), 2385-2396. https://doi.org/10.17341/gazimmfd.915691 |