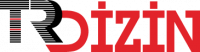
Adaptif Öğrenme Sözlüğü Temelli Duygu Analiz Algoritması Önerisi
Yıl: 2018 Cilt: 11 Sayı: 3 Sayfa Aralığı: 245 - 253 Metin Dili: Türkçe DOI: 10.17671/gazibtd.342419 İndeks Tarihi: 18-07-2019
Adaptif Öğrenme Sözlüğü Temelli Duygu Analiz Algoritması Önerisi
Öz: Teknolojik ilerlemeler bilinen anlamdaki veri kavramını geliştirmekte veçeşitli tipte, büyük boyutlarda veri setlerini ulaşılabilir kılmaktadır.Özellikle kişisel cihazların ve sosyal medya kanallarının yaygınlaşması ilebireyler yaşamları ve duyguları hakkında çeşitli bilgiler paylaşmaktadırlar. Buda araştırmacılara anket gibi tekniklerle elde edilmesi güç boyutlarda verisağlayan kaynaklar oluşmasını sağlamaktadır. Metin verileri de hem sosyal medyaaracılığı ile en çok biriken veri çeşidinden biri olması hem de içerdiğiduygular bakımından önem taşımaktadır. Kişilerin duygularını ifade etmek veyagörüşlerini bildirmek amacıyla yazdıkları metinlerin analiz edilerek anlamlıbilgilere dönüştürülmesi ve değer yaratılması veri analitiğinin amaçlarından birisidir.Bu doğrultuda veri setinin analizi kadar çıktıların yorumlanarak değeryaratılması da büyük önem taşımaktadır. Bu amaçla çalışmada çıktılarıyorumlayabilecek fakat yazılım altyapısı güçlü olmayan araştırmacıların dakolaylıkla faydalanabileceği bir model önerilmiştir. Böylece çıktıların değerbulacağı işletme, ekonomi ve sosyoloji gibi sosyal bilimler alanlarındanaraştırmacıların gelişen teknoloji ve veri setlerini değerlendirmesihedeflenmiştir. Yarı denetimli öğrenme ile pozitif ve negatif duygu sözlüklerigenişletilmiş sözlük temelli duygu analizi tekniği kullanılan ve performansıyüksek, uygulama anlamında çeşitli düzeyde yazılım bilgisine sahip kişilerehitap eden bir duygu analizi modeli sunulmuştur.
Anahtar Kelime: Konular:
Adaptive Learning Lexicon Based Sentiment Analysis Proposal
Öz: Technological improvements cause to enlarge data sources, types and volume. Especially personnel devices and social media, which are valuable external resources, provides large datasets that is inaccessible to collect with survey or other traditional techniques. Mostly acquired type, Text data includes mysterious information about people’s opinion or idea. Hence, gathering, analyzing the text data and interpreting the results creates significant value for the researchers from such as business, economy and sociology. Proposed algorithm assumes that each dataset needs specific opinion lexicons, so semi-supervised sentiment analysis algorithm is generated. Another feature of the proposed algorithm is simplicity. Without an expertise on programming, this algorithm can be applied for the social sciences researchers. Thus, the study introduces a model that makes sentiment analysis possible for lots of researcher who have little expertise on programming but can interpret the results and creates value.
Anahtar Kelime: Konular:
Belge Türü: Makale Makale Türü: Araştırma Makalesi Erişim Türü: Erişime Açık
- A. Kapucugil-İkiz, G. Özdağoğlu, “Text mining as a supporting process for VoC clarification”, Alphanumeric Journal, 3(1), 25-40, 2015.
- B. Pang, L. Lee, “Seeing stars: Exploiting class relationships for sentiment categorization with respect to rating scales, In Proceedings of the 43rd annual meeting on association for computational linguistics”, Proceedings of the 43rd annual meeting on association for computational linguistics, 115-124, Haziran 2005.
- M. Thelwall, K. Buckley, G. Paltoglou, D. Cai, A. Kappas, “Sentiment strength detection in short informal text”, Journal of the American Society for Information Science and Technology, 61(12), 2544-2558, 2010.
- A. Neviarouskaya, H. Prendinger, M. Ishizuka, “Textual affect sensing for sociable and expressive online communication”, International Conference on Affective Computing and Intelligent Interaction, 218-229, 2007.
- W. He, S. Zha, L. Li, “Social media competitive analysis and text mining: A case study in the pizza industry”, International Journal of Information Management, 33(3), 464-472, 2013.
- M. M. Mostafa, “More than words: Social networks’ text mining for consumer brand sentiments”, Expert Systems with Applications, 40(10), 4241-4251, 2013.
- M. Ghıassı, J. Skinner, D. Zımbra, “Twitter brand sentiment analysis: A hybrid system using n-gram analysis and dynamic artificial neural network” Expert Systems with applications, 40(16), 6266- 6282, 2013.
- X. Zhang, H. Fuehres, P. A. Gloor, “Predicting stock market indicators through twitter - I hope it is not as bad as I fear-” Procedia-Social and Behavioral Sciences, 26, 55-62, 2011.
- J. Bollen, H. Mao, X. Zeng, “Twitter mood predicts the stock market”, Journal of Computational Science, 2(1), 1-8, 2011.
- A. Mittal, A. Goel, Stock prediction using twitter sentiment analysis, Standford University, CS229, 2012.
- S. Bouktif, M. A. Awad, “Ant colony based approach to predict stock market movement from mood collected on Twitter”, Proceedings of the 2013 IEEE/ACM International Conference on Advances in Social Networks Analysis and Mining, 837-845, 2013.
- A. Porshnev, I. Redkin, A. Shevchenko, “Machine learning in prediction of stock market indicators based on historical data and data from twitter sentiment analysis”, In 2013 IEEE 13th International Conference on Data Mining Workshops, 440-444, 2013.
- F. Corea, “Can Twitter Proxy the Investors' Sentiment? The Case for the Technology Sector”, Big Data Research, 4, 70-74, 2016.
- S. Asur, B. A. Huberman, “Predicting the future with social media”, Proceedings of the 2010 IEEE/WIC/ACM International Conference on Web Intelligence and Intelligent Agent Technology-Volume 01, 492-499, 2010.
- H. Rui, Y. Liı, A. Whinston, “Whose and what chatter matters? The effect of tweets on movie sales”, Decision Support Systems, 55(4), 863-870, 2013.
- T. Kim, J. Hong, P. Kang, “Box office forecasting using machine learning algorithms based on SNS data”, International Journal of Forecasting, 31(2), 364-390, 2015.
- A. Culotta, “Towards detecting influenza epidemics by analyzing Twitter messages”, In Proceedings of the first workshop on social media analytics - ACM, 115-122, 2010.
- E. Aramaki, S. Maskawa, M. Morıta, “Twitter catches the flu: detecting influenza epidemics using Twitter”, In Proceedings of the conference on empirical methods in natural language processing (Association for Computational Linguistics), 1568-1576, 2011.
- T. Bodnar, M. Salathé, “Validating Models For Disease Detection Using Twitter”, In Proceedings Of The 22nd International Conference on World Wide Web - ACM, 699-702, 2013.
- M. Meral, B. Diri, “Sentiment analysis on Twitter”, IEEE 22nd. International Conference On Signal Processing and Communications Applications, 690-693, 2014.
- Ö. Çoban, B. Özyer, G. T. Özyer, “Sentiment analysis for Turkish Twitter feeds”, IEEE 23th International Conference On Signal Processing and Communications Applications, 2388-2391, 2015.
- A. B. Elıaçık, N. Erdogan, “Mikro Bloglardaki Finans Toplulukları için Kullanıcı Ağırlıklandırılmış Duygu Analizi Yöntemi”, UYMS, 2015.
- C. Akarsu, B. Diri, “Turkish TV rating prediction with Twitter”, IEEE 24th International Conference On Signal Processing and Communication Application, 345-348, 2016.
- D. Kayahan, A. Sergin, B. Diri, “Determination of TV programme ratings by twitter”, IEEE 21st International Conference On Signal Processing and Communications Applications, 1-4, 2013.
- S. Stieglitz, L. Dang-Xuan, A. Bruns, C. Neuberger, “Social media analytics”, Wirtschaftsinformatik, 56(2), 101-109, 2014.
- R. Xia, C. Zong, S. Li, “Ensemble of feature sets and classification algorithms for sentiment classification”, Information Sciences, 181(6), 1138-1152, 2011.
- M. N. Rahman, A. Esmailpour, J. Zhao, “Machine Learning with Big Data An Efficient Electricity Generation Forecasting System”, Big Data Research, 5, 9-15, 2016.
- B. Liu, “Sentiment analysis and opinion mining”, Synthesis lectures on human language technologies, 5(1), 1–167, 2012.
- W. Medhat, A. Hassan, H. Korashy, “Sentiment analysis algorithms and applications: A survey”, Ain Shams Engineering Journal, 5(4), 1093-1113, 2014.
- C. Catal, M. Nangir, “A sentiment classification model based on multiple classifiers”, Applied Soft Computing, 50, 135-141, 2017.
- K. Çalış, O. Gazdağı, O. Yıldız, “Reklam İçerikli Epostaların Metin Madenciliği Yöntemleri ile Otomatik Tespiti”, Bilişim Teknolojileri Dergisi, 6(1), 1-7, 2013.
- B. Liu, “Sentiment analysis and subjectivity”, In Handbook of Natural Language Processing, Cilt 2, Chapman and Hall/CRC, 627-666, 2010.
- L. Zhang, Sentiment analysis on Twitter with stock price and significant keyword correlation, Doktora Tezi, The University of Texas at Austing, Department of Computer Science, 2013.
APA | KARAÖZ AKIN B, GÜRSOY ŞİMŞEK U (2018). Adaptif Öğrenme Sözlüğü Temelli Duygu Analiz Algoritması Önerisi. , 245 - 253. 10.17671/gazibtd.342419 |
Chicago | KARAÖZ AKIN Burcu,GÜRSOY ŞİMŞEK U. Tuğba Adaptif Öğrenme Sözlüğü Temelli Duygu Analiz Algoritması Önerisi. (2018): 245 - 253. 10.17671/gazibtd.342419 |
MLA | KARAÖZ AKIN Burcu,GÜRSOY ŞİMŞEK U. Tuğba Adaptif Öğrenme Sözlüğü Temelli Duygu Analiz Algoritması Önerisi. , 2018, ss.245 - 253. 10.17671/gazibtd.342419 |
AMA | KARAÖZ AKIN B,GÜRSOY ŞİMŞEK U Adaptif Öğrenme Sözlüğü Temelli Duygu Analiz Algoritması Önerisi. . 2018; 245 - 253. 10.17671/gazibtd.342419 |
Vancouver | KARAÖZ AKIN B,GÜRSOY ŞİMŞEK U Adaptif Öğrenme Sözlüğü Temelli Duygu Analiz Algoritması Önerisi. . 2018; 245 - 253. 10.17671/gazibtd.342419 |
IEEE | KARAÖZ AKIN B,GÜRSOY ŞİMŞEK U "Adaptif Öğrenme Sözlüğü Temelli Duygu Analiz Algoritması Önerisi." , ss.245 - 253, 2018. 10.17671/gazibtd.342419 |
ISNAD | KARAÖZ AKIN, Burcu - GÜRSOY ŞİMŞEK, U. Tuğba. "Adaptif Öğrenme Sözlüğü Temelli Duygu Analiz Algoritması Önerisi". (2018), 245-253. https://doi.org/10.17671/gazibtd.342419 |
APA | KARAÖZ AKIN B, GÜRSOY ŞİMŞEK U (2018). Adaptif Öğrenme Sözlüğü Temelli Duygu Analiz Algoritması Önerisi. Bilişim Teknolojileri Dergisi, 11(3), 245 - 253. 10.17671/gazibtd.342419 |
Chicago | KARAÖZ AKIN Burcu,GÜRSOY ŞİMŞEK U. Tuğba Adaptif Öğrenme Sözlüğü Temelli Duygu Analiz Algoritması Önerisi. Bilişim Teknolojileri Dergisi 11, no.3 (2018): 245 - 253. 10.17671/gazibtd.342419 |
MLA | KARAÖZ AKIN Burcu,GÜRSOY ŞİMŞEK U. Tuğba Adaptif Öğrenme Sözlüğü Temelli Duygu Analiz Algoritması Önerisi. Bilişim Teknolojileri Dergisi, vol.11, no.3, 2018, ss.245 - 253. 10.17671/gazibtd.342419 |
AMA | KARAÖZ AKIN B,GÜRSOY ŞİMŞEK U Adaptif Öğrenme Sözlüğü Temelli Duygu Analiz Algoritması Önerisi. Bilişim Teknolojileri Dergisi. 2018; 11(3): 245 - 253. 10.17671/gazibtd.342419 |
Vancouver | KARAÖZ AKIN B,GÜRSOY ŞİMŞEK U Adaptif Öğrenme Sözlüğü Temelli Duygu Analiz Algoritması Önerisi. Bilişim Teknolojileri Dergisi. 2018; 11(3): 245 - 253. 10.17671/gazibtd.342419 |
IEEE | KARAÖZ AKIN B,GÜRSOY ŞİMŞEK U "Adaptif Öğrenme Sözlüğü Temelli Duygu Analiz Algoritması Önerisi." Bilişim Teknolojileri Dergisi, 11, ss.245 - 253, 2018. 10.17671/gazibtd.342419 |
ISNAD | KARAÖZ AKIN, Burcu - GÜRSOY ŞİMŞEK, U. Tuğba. "Adaptif Öğrenme Sözlüğü Temelli Duygu Analiz Algoritması Önerisi". Bilişim Teknolojileri Dergisi 11/3 (2018), 245-253. https://doi.org/10.17671/gazibtd.342419 |