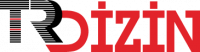
Türkiye’deki Farklı Sektörlere Ait Sera Gazı Emisyon Değerlerinin Çok Katmanlı Algılayıcılar ve Topluluk Öğrenmesi Yöntemleri ile Tahmin Edilmesi
Yıl: 2020 Cilt: 12 Sayı: 2 Sayfa Aralığı: 464 - 478 Metin Dili: Türkçe DOI: 10.29137/umagd.646038 İndeks Tarihi: 29-04-2021
Türkiye’deki Farklı Sektörlere Ait Sera Gazı Emisyon Değerlerinin Çok Katmanlı Algılayıcılar ve Topluluk Öğrenmesi Yöntemleri ile Tahmin Edilmesi
Öz: Küresel ısınmaya neden olan karbondioksit (CO2), Nitröz oksit (N2O) ve Metan (CH4) çeşitli sektörler tarafından oluşturulan sera gazlarıdır. Birleşmiş milletler iklim değişikliği çerçeve sözleşmesince (UNFCCC) Türkiye’nin de içinde olduğu ülkelerin çeşitli sektörleri tarafından oluşturulan sera gazı emisyon değerleri kayıt altına alınarak takip edilmektedir. Ülkelerin oluşturdukları sera gazı emisyon değerleri zaman içinde birçok etkene göre farklılık oluşturabilir. Bu değerin tahmin edilmesi ülkeler açısından önemlidir. Çalışmada 1990-2014 yılları arasındaki farklı sektörlerdeki sera gazı emisyon değerleri ile yapay sinir ağı modeli ve topluluk öğrenme yöntemleri kullanılarak en iyi tahmin modelini oluşturmak için çeşitli deneyler gerçekleştirilmiştir. Çok katmanlı algılayıcı (ÇKA) modeli ile en yüksek R2 performans değeri üretim, enerji endüstrisi, yerleşim ve ulaşım sektörleri içinsırasıyla 0.86, 0.93, 0.91 ve 0.95 olarak bulunmuştur. Topluluk öğrenme yöntemlerine dayanan algoritmalar da ise ortalama R2 değerleri bakımından en iyi performansı Extra Tree regresyon ile kurulan model sağlamıştır. Kullanılan tahmin modellerinde en iyi performans Çok Katmanlı Algılayıcı tabanlı model ile elde edilmiştir. Çalışmada yer alan sektörlerden 2020 yılında doğaya salınacağı öngörülen sera gazı emisyon değerleri açısından geçmiş dönemi kapsayan salınım değerlerinin ortalamaları ile önemli farklılıklar olduğu görülmektedir. Ayrıca geçmiş döneme oranla üretim sektörü alanında ortalama %36, enerji endüstrisi sektöründe %64 ve ulaşım sektöründe %63 oranında bir artış öngörülmektedir.
Anahtar Kelime: Prediction with Ensemble Learning Methods and Multi-layer Perceptions of Greenhouse Gas Emission Belonging to Different Sectors in Turkey
Öz: Global warming causatives such as carbon dioxide (CO2), Nitrous oxide (N2O) and Methane (CH4) are greenhouse gases created by various sectors. Greenhouse gas emission values created by various sectors of countries including Turkey are registered and tracked by the United Nations framework agreement on climate change (UNFCCC). The greenhouse gas emission values created by the countries may vary over time depending on many factors. Estimating this value is important for countries. In the study, various experiments were carried out to create the best estimation model with artificial neural network model and community learning methods by using greenhouse gas emission values in different sectors between 1990-2014. The highest R2 performance value with the multi-layer sensor (MLS) model was 0.86, 0.93, 0.91 and 0.95 respectively for the production, energy industry, residential and transportation sectors. Algorithms based on community learning methods also provided the best performance in terms of average R2 values with a model established by Extra Tree regression. The best performance in the prediction models used was achieved with the Multi-Layer Sensor-based model. It is observed that there are significant differences between the averages of the oscillation values covering the past period in terms of greenhouse gas emission values, which are projected to be released into nature in 2020 from the sectors involved in the study. In addition, an average increase of 36% is expected in the field of production sector, 64% in the energy industry sector and 63% in the transportation sector compared to the past period.
Anahtar Kelime: Belge Türü: Makale Makale Türü: Araştırma Makalesi Erişim Türü: Erişime Açık
- Abid, M. (2017). Does economic , fi nancial and institutional developments matter for environmental quality? A comparative analysis of EU and MEA countries. Journal of Environmental Management, 188(2), 183-194. https://doi.org/10.1016/j.jenvman.2016.12.007.
- Al-Ghobari, H. M., El-Marazky, M. S., Dewidar, A. Z., & Mattar, M. A. (2018). Prediction of wind drift and evaporation losses from sprinkler irrigation using neural network and multiple regression techniques. Agricultural Water Management, 195, 211-221.
- Ağaçayak, T., & Öztürk, L. (2017). Türkı̇ye ’ De Tarim Sektöründen Kaynaklanan Sera GaziEmı̇syonlarinin. İPM – MercatoPolı̇tı̇ka Notu, 3–15.
- Araabi, N. (2012). Prediction of Climate Change Induced Temperature Rise in Regional Scale Using Neural Network. International Journal of Environmental Research, 6(3), 677–688.
- Assareh, E., & Nedaei, M. (2018). A metaheuristic approach to forecast the global carbon dioxide emissions. International Journal of Environmental Studies, 75(1), 99–120. https://doi.org/10.1080/00207233.2017.1374075.
- Azid, A., Juahir, H., Toriman, M. E., Kamarudin, M. K. A., Saudi, A. S. M., Hasnam, C. N. C., … Yamin, M. (2014). Prediction of the level of air pollution using principal component analysis and artificial neural network techniques: A case study in Malaysia. Water, Air, and Soil Pollution, 225(8). https://doi.org/10.1007/s11270-014-2063-1.
- Baghban, A., Ahmadi, M. A., & Shahraki, B. H. (2015). Prediction carbon dioxide solubility in presence of various ionic liquids using computational intelligence approaches. Journal of Supercritical Fluids, 98, 50–64. https://doi.org/10.1016/j.supflu.2015.01.002.
- Behrang, M. A., Assareh, E., Assari, M. R., & Ghanbarzadeh, A. (2011). Using bees algorithm and artificial neural network to forecast world carbon dioxide emission. Energy Sources, Part A: Recovery, Utilization and Environmental Effects, 33(19), 1747–1759. https://doi.org/10.1080/15567036.2010.493920.
- Bolanča, T., Strahovnik, T., Ukić, Š., Stankov, M. N., & Rogošić, M. (2017). Modeling of policies for reduction of GHG emissions in energy sector using ANN: case study—Croatia (EU). Environmental Science and Pollution Research, 24(19), 16172–16185. https://doi.org/10.1007/s11356-017-9216-x.
- Breiman, L. (1996). Bagging Predictors. Machine Learning, 24(421), 123–140. https://doi.org/10.1007/BF00058655.
- Buisson, L., Grenouillet, G., Casajus, N., & Lek, S. (2010). Predicting the Potential Impacts of Climate Change on Stream Fish Ass javascript :; emblages. American Fisheries Society Symposium, 5, 327–346.
- Change, I. P. O. C. (2007). Climate Change 2007: The Physical Science Basis: Summary for Policymakers. Geneva: IPCC, 996. https://doi.org/10.1038/446727a.
- Chen, D., Li, Y., Grace, P., & Mosier, A. R. (2008). N2O emissions from agricultural lands: A synthesis of simulation approaches. Plant and Soil, 309(1–2), 169–189. https://doi.org/10.1007/s11104-008-9634-0.
- Chen, X. (Michael), Zahiri, M., & Zhang, S. (2017). Understanding ridesplitting behavior of on-demand ride services: An ensemble learning approach. Transportation Research Part C: Emerging Technologies, 76, 51–70. https://doi.org/10.1016/j.trc.2016.12.018.
- Choi, S., & Hur, J. (2020). An ensemble learner-based bagging model using past output data for photovoltaic forecasting. Energies, 13(6). https://doi.org/10.3390/en13061438.
- Çoban, O., & Şahbaz Kılınç, N. (2014). Yenı̇lenebı̇lı̇r enerjı̇ tüketı̇mı̇ ve karbon emı̇syonu ı̇lı̇şkı̇sı̇: tr örneğı̇ ∗. Sosyal Bilimler Enstitüsü Dergisi, (38), 195–208.
- Collins, M. A. T. (2007). Ensembles and probabilities: A new era in the prediction of climate change. Philosophical Transactions of the Royal Society A: Mathematical, Physical and Engineering Sciences, 365(1857), 1957–1970. https://doi.org/10.1098/rsta.2007.2068.
- Cui, H. Z., Sham, F. C., Lo, T. Y., & Lum, H. T. (2011). Appraisal of alternative building materials for reduction of CO2 emissions by case modeling. International Journal of Environmental Research, 5(1), 93–100.
- Dam, M. M. (2014). Sera Gazı Emisyonlarının Makroekonomik Değişkenlerle İlişkisi: OECD Ülkeleri için Panel Veri Analizi.Yüksek Lisans Tezi, Sosyal Bilimler Enstitüsü, Adnan Menderes Üniversitesi, 1–159.
- Ergün, S., & Atay Polat, M. (2017). G7 Ülkelerinde CO2 Emisyonu, Elektrik Tüketimi ve Büyüme İlişkisi. Siyaset, Ekonomi ve Yönetim Araştırmaları Dergisi, (2).
- Ersoy, A. E. (2017). Türkiye’nin Hayvansal Gübre Kaynaklı Sera Gazı Emı̇syonları Durumu ve Bı̇yogaz Enerjı̇si Potansı̇yelı̇. Yüksek Lisans Tezi, Fen Bilimleri Estitüsü, Hacettepe Üniversitesi, 1–127.
- Fan, J. L., Zhang, X., Zhang, J., & Peng, S. (2015). Efficiency evaluation of CO2 utilization technologies in China: A superefficiency DEA analysis based on expert survey. Journal of CO2 Utilization, 11(2015), 54–62. https://doi.org/10.1016/j.jcou.2015.01.004.
- Fu, W., Han, W., Xu, T., Kim, Y., Lyu, C., & Zheng, A. (2019). Exploring the Interconnection of Greenhouse Gas Emission and Socio-economic Factors. SAR Journal, 2(3), 115–120. https://doi.org/10.18421/SAR23-05.
- Güçlü, B. S. (2006). Kyoto protokolü ve türkđye’nđn protokol karşisinda durumu. Metalurji Dergisi, 142, 1-4.
- Gurney, K. (2014). An introduction to neural networks. London and New York: CRC press. Retrieved from http://www.ghbook.ir/index.php?name= های رسانه و فرهنگنوین&option=com_dbook&task=readonline&book_id=13650&page=73&chkhashk=ED9C9491B4&Itemid=218&lang=fa&tmpl= component.
- Hamzacebi, C., & Karakurt, I. (2015). Forecasting the energy-related CO<inf>2</inf> emissions of Turkey using a grey prediction model. Energy Sources, Part A: Recovery, Utilization and Environmental Effects, 37(9), 1023–1031. https://doi.org/10.1080/15567036.2014.978086.
- Harrington P (2012) Machine learning in action. Manning Publications Co.
- Hebb, D. O. (1949). The Organization of Behavior A Neuropsychological Theory. Central Institute of Education, New York: Wiley (Vol. 65). https://doi.org/10.1901/jaba.1992.25-575.
- IPCC. (2014). Climate Change 2014 Synthesis Report. Contribution of Working Groups I, II and III to the Fifth Assessment Report of the Intergovernmental Panel on Climate Change [Core Writing Team, R.K. Pachauri and L.A. Meyer (Eds.)]. IPCC,
- Geneva, Switzerland, (1), 151.https://doi.org/10.1177/0002716295541001010.
- Işık, N. (2014). Sosyo Ekonomi Ulaştırma Sektöründe CO2 Emisyonu ve Enerji Ar-Ge Harcamaları İlişkisi. Sosyoekonomi /, 2014–2(December), 322–346.
- Jatmiko, W., Purnomo, D. M. J., Alhamidi, M., Wibisono, A., Wisesa, H., Mursanto, P., … Addana, F. (2016). Algal growth rate modeling and prediction optimization using incorporation of MLP and CPSO algorithm. 2015 International Symposium on Micro-Nano Mechatronics and Human Science, MHS 2015, (November), 1–8. https://doi.org/10.1109/MHS.2015.7438293.
- Julian, D. (2016). Designing Machine Learning Systems with Python. PACKT Publishing open source community experience distilled (Vol. 53).
- Kainuma, M., Matsuoka, Y., & Morita, T. (2000). The AIM/end-use model and its application to forecast Japanese carbon dioxide emissions. European Journal of Operational Research, 122(2), 416–425. https://doi.org/10.1016/S0377-2217(99)00243- X.
- Kara, G., Yalınız, İ., & Sayar, M. (2019). Konya İli Hayvansal Gübre Kaynaklı Sera Gazı Emisyonları Durumu. Ulusal Çevre Bilimleri Araştırma Dergisi, 2(2), 57–60.
- Khan, M. J. U. R., & Awasthi, A. (2020). Machine learning model development for predicting road transport GHG emissions in Canada. WSB Journal of Business and Finance, 53(2), 55–72. https://doi.org/10.2478/wsbjbf-2019-0022.
- Khan, S., Rahmani, H., Shah, S. A. A., & Bennamoun, M. (2018). A Guide to Convolutional Neural Networks for Computer Vision. Synthesis Lectures on Computer Vision, 8(1), 1–207. https://doi.org/10.2200/s00822ed1v01y201712cov015.
- Khoshnevisan, B., Rafiee, S., Omid, M., Yousefi, M., & Movahedi, M. (2013). Modeling of energy consumption and GHG (greenhouse gas) emissions in wheat production in Esfahan province of Iran using artificial neural networks. Energy, 52, 333–338. https://doi.org/10.1016/j.energy.2013.01.028.
- Kolasa-Więcek, A. (2018). Neural Modeling of Greenhouse Gas Emission from Agricultural Sector in European Union Member Countries. Water, Air, and Soil Pollution, 229(6), 7–9. https://doi.org/10.1007/s11270-018-3861-7.
- Kovancılar, B. (2001). Küresel Isınma Sorununun Çözümünde Karbon Vergisi ve Etkinliği. Yönetim ve Ekonomı̇, 8(2), 10–13.
- Krstanoski, N. (2006). Defining The Policy For Reduction Of CO2 Emissions From The Road Transport Sector In Republic Of Macedonia, 1–15.
- Kumar, S., & Muhuri, P. K. (2019). A novel GDP prediction technique based on transfer learning using CO2 emission dataset. Applied Energy, 253(May), 113476. https://doi.org/10.1016/j.apenergy.2019.113476.
- Li, Y., Han, Y., Wang, J., & Zhao, Q. (2018). A MBCRF algorithm based on ensemble learning for building demand response considering the thermal comfort. Energies, 11(12). https://doi.org/10.3390/en11123495.
- Li, S., Zhou, R., & Ma, X. (2010). The forecast of CO2emissions in China based on RBF neural networks. 2010 2nd International Conference on Industrial and Information Systems, IIS 2010, 1, 319–322. https://doi.org/10.1109/INDUSIS.2010.5565845.
- Liao, C. H., Lu, C. S., & Tseng, P. H. (2011). Carbon dioxide emissions and inland container transport in Taiwan. Journal of Transport Geography, 19(4), 722–728. https://doi.org/10.1016/j.jtrangeo.2010.08.013.
- Lu, I. J., Lewis, C., & Lin, S. J. (2009). The forecast of motor vehicle, energy demand and CO2 emission from Taiwan’s road transportation sector. Energy Policy, 37(8), 2952–2961. https://doi.org/10.1016/j.enpol.2009.03.039.
- Mahesh, G. U. (2018). Prediction of Transportation Carbon Emission using Spatio-temporal Datasets and Multilayer Perceptron Neural Network. International Journal of New Innovations in Engineering and Technology Prediction, 8(2), 39–48.
- Mohammed, A. A., & Aung, Z. (2016). Ensemble learning approach for probabilistic forecasting of solar power generation. Energies, 9(12). https://doi.org/10.3390/en9121017.
- Nwulu, N. I., & Agboola, O. P. A. (2012). Modelling CO2 Emissions Using Artificial Neural Networks. AWER Procedia Information Technology & Computer Science, 2, 407–411.
- Özmen, M. T. (2009). Sera Gazı - Küresel Isınma ve Kyoto Protokolü. Türkiye Mühendislik Haberleri, 42–46.
- Pabuçcu, H., & Bayramoğlu, T. (2016). Yapay Sinir Ağları ile CO2 Emisyonu Tahmini: Türkiye Örneği. Gazi Üniversitesi İktisadi ve İdari Bilimler Fakültesi Dergisi, 18(3), 762–778.
- Parker, W. S. (2010). Predicting weather and climate: Uncertainty, ensembles and probability. Studies in History and Philosophy of Science Part B - Studies in History and Philosophy of Modern Physics, 41(3), 263–272. https://doi.org/10.1016/j.shpsb.2010.07.006.
- Priddy, K.L., & Keller, P.E., (2005). Artificial neural networks: An introduction. SPIE- The International Society for OpticalEngineering, Washington, USA.
- Quesada-Rubio, J. M., Villar-Rubio, E., Mondéjar-Jiménez, J., & Molina-Moreno, V. (2011). Carbon dioxide emissions vs. allocation rights: Spanish case analysis. International Journal of Environmental Research, 5(2), 469–474. https://doi.org/10.22059/ijer.2011.331.
- Rosenblat, F. (1958). The perceptron: a probabilistic model for information storage and organization in the brain. Psychological Review, 65(6), 386.
- Sagi, O., & Rokach, L. (2018). Ensemble learning: A survey.
- Saleh, C., Dzakiyullah, N. R., & Nugroho, J. B. (2016). Carbon dioxide emission prediction using support vector machine. IOP Conference Series: Materials Science and Engineering, 114(1). https://doi.org/10.1088/1757-899X/114/1/012148.
- Sangeetha, A., & Amudha, T. (2018). A Novel Bio-Inspired Framework for CO2 Emission Forecast in India. Procedia Computer Science, 125, 367–375. https://doi.org/10.1016/j.procs.2017.12.048.
- Solomon, S., Qin, D., Manningm, M., Marquis, M., Averyt, K., Tignor, M. B., Chen, Z. H. (2007). The Physical Science Basis. Contribution of Working Group I to the Fourth Assessment Report of the Intergovernmental Panel on Climate Change.
- Cambridge University Press: Cambridge, United Kingdom and New York, NY, USA. https://doi.org/10.1017/CBO9781107415324.Summary.
- Theodoridis, S., & Koutroumbas, K. (2009). Pattern recognition. 2003. Elsevier Inc.
- Uğuz, S. (2019). Makine öğrenmesi- Teorik yönleri ve Python uygulamaları ile bir yapay zeka ekolü. Nobel akademik yayın, 1,312.
- Wang, T., Li, H., Zhang, J., & Lu, Y. (2012). Influencing Factors of Carbon Emission in China’s Road Freight Transport. Procedia - Social and Behavioral Sciences, 43, 54–64. https://doi.org/10.1016/j.sbspro.2012.04.077.
- Yan, X., & Crookes, R. J. (2010). Energy demand and emissions from road transportation vehicles in China. Progress in Energy and Combustion Science, 36(6), 651–676. https://doi.org/10.1016/j.pecs.2010.02.003.
- Yılmaz, H., & Yılmaz, M. (2013). Forecastıng Co2 Emıssıons For Turkey By Usıng The Grey Predıctıon Method. Journal of Engineering and Natural Sciences, (444), 141–148.
- Zhou, J., Yu, X., & Yuan, X. (2018). Predicting the carbon price sequence in the shenzhen emissions exchange using a multiscale ensemble forecasting model based on ensemble empirical mode decomposition. Energies, 11(7), 1–17. https://doi.org/10.3390/en11071907.
- Zhou, Z. H. (2012). Ensemble methods: foundations and algorithms. CRC press.
- Zhu, B. (2012). A novel multiscale ensemble carbon price prediction model integrating empirical mode decomposition, genetic algorithm and artificial neural network. Energies, 5(2), 355–370. https://doi.org/10.3390/en5020355.
APA | ORAL O, Uğuz S (2020). Türkiye’deki Farklı Sektörlere Ait Sera Gazı Emisyon Değerlerinin Çok Katmanlı Algılayıcılar ve Topluluk Öğrenmesi Yöntemleri ile Tahmin Edilmesi. , 464 - 478. 10.29137/umagd.646038 |
Chicago | ORAL Okan,Uğuz Sinan Türkiye’deki Farklı Sektörlere Ait Sera Gazı Emisyon Değerlerinin Çok Katmanlı Algılayıcılar ve Topluluk Öğrenmesi Yöntemleri ile Tahmin Edilmesi. (2020): 464 - 478. 10.29137/umagd.646038 |
MLA | ORAL Okan,Uğuz Sinan Türkiye’deki Farklı Sektörlere Ait Sera Gazı Emisyon Değerlerinin Çok Katmanlı Algılayıcılar ve Topluluk Öğrenmesi Yöntemleri ile Tahmin Edilmesi. , 2020, ss.464 - 478. 10.29137/umagd.646038 |
AMA | ORAL O,Uğuz S Türkiye’deki Farklı Sektörlere Ait Sera Gazı Emisyon Değerlerinin Çok Katmanlı Algılayıcılar ve Topluluk Öğrenmesi Yöntemleri ile Tahmin Edilmesi. . 2020; 464 - 478. 10.29137/umagd.646038 |
Vancouver | ORAL O,Uğuz S Türkiye’deki Farklı Sektörlere Ait Sera Gazı Emisyon Değerlerinin Çok Katmanlı Algılayıcılar ve Topluluk Öğrenmesi Yöntemleri ile Tahmin Edilmesi. . 2020; 464 - 478. 10.29137/umagd.646038 |
IEEE | ORAL O,Uğuz S "Türkiye’deki Farklı Sektörlere Ait Sera Gazı Emisyon Değerlerinin Çok Katmanlı Algılayıcılar ve Topluluk Öğrenmesi Yöntemleri ile Tahmin Edilmesi." , ss.464 - 478, 2020. 10.29137/umagd.646038 |
ISNAD | ORAL, Okan - Uğuz, Sinan. "Türkiye’deki Farklı Sektörlere Ait Sera Gazı Emisyon Değerlerinin Çok Katmanlı Algılayıcılar ve Topluluk Öğrenmesi Yöntemleri ile Tahmin Edilmesi". (2020), 464-478. https://doi.org/10.29137/umagd.646038 |
APA | ORAL O, Uğuz S (2020). Türkiye’deki Farklı Sektörlere Ait Sera Gazı Emisyon Değerlerinin Çok Katmanlı Algılayıcılar ve Topluluk Öğrenmesi Yöntemleri ile Tahmin Edilmesi. Uluslararası Mühendislik Araştırma ve Geliştirme Dergisi, 12(2), 464 - 478. 10.29137/umagd.646038 |
Chicago | ORAL Okan,Uğuz Sinan Türkiye’deki Farklı Sektörlere Ait Sera Gazı Emisyon Değerlerinin Çok Katmanlı Algılayıcılar ve Topluluk Öğrenmesi Yöntemleri ile Tahmin Edilmesi. Uluslararası Mühendislik Araştırma ve Geliştirme Dergisi 12, no.2 (2020): 464 - 478. 10.29137/umagd.646038 |
MLA | ORAL Okan,Uğuz Sinan Türkiye’deki Farklı Sektörlere Ait Sera Gazı Emisyon Değerlerinin Çok Katmanlı Algılayıcılar ve Topluluk Öğrenmesi Yöntemleri ile Tahmin Edilmesi. Uluslararası Mühendislik Araştırma ve Geliştirme Dergisi, vol.12, no.2, 2020, ss.464 - 478. 10.29137/umagd.646038 |
AMA | ORAL O,Uğuz S Türkiye’deki Farklı Sektörlere Ait Sera Gazı Emisyon Değerlerinin Çok Katmanlı Algılayıcılar ve Topluluk Öğrenmesi Yöntemleri ile Tahmin Edilmesi. Uluslararası Mühendislik Araştırma ve Geliştirme Dergisi. 2020; 12(2): 464 - 478. 10.29137/umagd.646038 |
Vancouver | ORAL O,Uğuz S Türkiye’deki Farklı Sektörlere Ait Sera Gazı Emisyon Değerlerinin Çok Katmanlı Algılayıcılar ve Topluluk Öğrenmesi Yöntemleri ile Tahmin Edilmesi. Uluslararası Mühendislik Araştırma ve Geliştirme Dergisi. 2020; 12(2): 464 - 478. 10.29137/umagd.646038 |
IEEE | ORAL O,Uğuz S "Türkiye’deki Farklı Sektörlere Ait Sera Gazı Emisyon Değerlerinin Çok Katmanlı Algılayıcılar ve Topluluk Öğrenmesi Yöntemleri ile Tahmin Edilmesi." Uluslararası Mühendislik Araştırma ve Geliştirme Dergisi, 12, ss.464 - 478, 2020. 10.29137/umagd.646038 |
ISNAD | ORAL, Okan - Uğuz, Sinan. "Türkiye’deki Farklı Sektörlere Ait Sera Gazı Emisyon Değerlerinin Çok Katmanlı Algılayıcılar ve Topluluk Öğrenmesi Yöntemleri ile Tahmin Edilmesi". Uluslararası Mühendislik Araştırma ve Geliştirme Dergisi 12/2 (2020), 464-478. https://doi.org/10.29137/umagd.646038 |