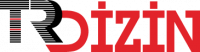
Pay Senedi Fiyat Yönünün Makine Öğrenmesi Yöntemleri ile Tahmini: Borsa İstanbul Örneği
Yıl: 2021 Cilt: 12 Sayı: 1 Sayfa Aralığı: 89 - 110 Metin Dili: Türkçe DOI: 10.20409/berj.2021.312 İndeks Tarihi: 17-06-2021
Pay Senedi Fiyat Yönünün Makine Öğrenmesi Yöntemleri ile Tahmini: Borsa İstanbul Örneği
Öz: Bu çalışmada Borsa İstanbul 30 Endeksinde ve Kurumsal Yönetim Endeksinde bulunan beşimalat sanayi şirketinin 2010/3 ve 2020/3 dönemine ait verilerle üç ay sonraki ortalaması alınmışpay senedi fiyat yönü tahmin edilmiştir. Veri seti, örnek kapsamındaki şirketlerin üçer aylık dokuzmali tablo verisi ve üç aylık ortalaması alınmış beş makroekonomik değişkenden oluşmaktadır.Tahmin yöntemleri olarak Yapay Sinir Ağları, Sınıflandırma ve Regresyon Ağacı ile K- En YakınKomşu Algoritması kullanılmıştır. Çalışmada kullanılan tüm yöntemlerde 10 katlı çaprazdoğrulama yöntemi kullanılmıştır. Yapay Sinir Ağları ile Sınıflandırma ve Regresyon Ağacıanalizlerinde en iyi parametrelerin ve performans kriterlerinin belirlenmesi fonksiyonu kullanılarakverilen parametre aralıkları doğrultusunda en iyi sonuç veren modeller elde edilmiştir. Analizsonucuna göre pay senedi fiyat yününü Yapay Sinir Ağları %98,05, Sınıflandırma ve RegresyonAğacı %96,10 ve K- En Yakın Komşu Algoritması %92,20 oranında genel sınıflandırma doğruluğuelde etmiştir. Sınıflandırma ve Regresyon Ağacının oluşturulmasında verileri ikiye bölen önemlideğişkenler olarak “Net Kâr Marjı”, “Fiyat/Kazanç Oranı”, “Hisse Başına Kâr Oranı”, “CDS Primi (üçaylık ortalama)” ve “Tüketici Güven Endeksi” bulunmuştur. Bu bulgular, yatırımcıların buçalışmada kullanılan modelleri kullanabileceklerini ve/veya hali hazırda kullanmış olduklarımodellere dâhil edebileceklerini göstermektedir.
Anahtar Kelime: Predicting Direction of Stock Price Using Machine Learning Techniques: The Sample of Borsa Istanbul
Öz: In this study, stock price with the calculated next three-month average of five manufacturing industry companies in the Borsa İstanbul 30 Index and the Corporate Governance Index was predicted with the data of the 2010/3 and 2020/3 periods. The dataset consisted of quarterly nine financial statements and five macroeconomic variables with a three-month average of the sample companies. Artificial Neural Networks, Classification and Regression Tree, and K- Nearest Neighbor Algorithm were used as prediction methods. A 10-fold cross-validation method was used in all methods in the study. In Artificial Neural Networks, Classification and Regression Tree analysis, the models that gave the best results in line with the given parameter ranges were obtained by using the determining the best parameters and performance criteria function. According to the results of the analysis, general classification accuracy was achieved 98.05% for Artificial Neural Networks, 96.10% for Classification and Regression Tree, and 92.20% K-Nearest Neighbor Algorithm. “Net Profit Margin”, “Price/Earning”, “Profit Per Share”, “CDS Premium (3-month average)”, “Consumer Confidence Index” were found as important variables that divided the data into two in the creation of the Classification and Regression Tree (CART) analysis. This result shows that the models used in this study can be incorporated into the models used by investors.
Anahtar Kelime: Belge Türü: Makale Makale Türü: Araştırma Makalesi Erişim Türü: Erişime Açık
- Akay, E. Ç. (2018). Ekonometride yeni bir ufuk: Büyük veri ve makine öğrenmesi. Social Sciences Research Journal, 7(2), 41-53.
- Akcan, A., & Kartal, C. (2011). İMKB sigorta endeksini oluşturan şirketlerin hisse senedi fiyatlarının yapay sinir ağları ile tahmini. Muhasebe ve Finansman Dergisi, (51), 27-40.
- Akpınar, H. (2014). Data veri madenciliği veri analizi. İstanbul: Papatya Yayınları. Anbalagan, T., & Maheswari, S. U. (2015). Classification and prediction of stock market index based on fuzzy metagraph. Procedia Computer Science, (47), 214-221.
- Ataseven, B. (2013). Yapay sinir ağları ile öngörü modellemesi. Öneri Dergisi, 10(39), 101-115.
- Barak, S., & Modarres, M. (2015). Developing an approach to evaluate stocks by forecasting effective features with data mining methods. Expert Systems with Applications, (42), 1325-1339.
- Chen, Y., & Hao, Y. (2017). A feature weighted support vector machine and K-nearest neighbor algorithm for stock market indices prediction. Expert Systems with Applications, (80), 340-355.
- Çelik, U., Akçetin, E., & Gök, M. (2017). Rapidminer ile veri madenciliği. İstanbul: Pusula Yayınları.
- Dhanalakshmi, S., & Subramanian, C. (2014). An analysis of data mining applications for fraud detection in securities market. International Journal of Data Mining Techniques and Applications, (3), 326-335.
- Elmas, Ç. (2018). Yapay zeka uygulamaları. Ankara: Seçkin Yayıncılık.
- Enke, D., & Thawornwong, S. (2005). The use of data mining and neural networks for forecasting stock market returns. Expert Systems with Applications, (29), 927-940.
- Gaganis, C. (2009). Classification techniques for the identification of falsified financial statements: A comparative analysis. Intelligent Systems in Accounting, Finance and Management, (16), 207-229.
- Huang, W., Nakamori, Y., & Wang, S.-Y. (2005). Forecasting stock market movement direction with support vector machine. Computers & Operations Research, (32), 2513-2522.
- Imandoust, S. B., & Bolandraftar, M. (2014). Forecasting the direction of stock market index movement using three data mining techniques: The case of Tehran Stock Exchange. Int. Journal of Engineering Research and Applications, 4(6), 106-117.
- Jardin, P. D. (2016). A Two-stage classification technique for bankruptcy prediction. European Journal of Operational Research, (254), 236-252.
- Kara, Y., Boyacioglu, M. A., & Baykan, Ö. K. (2011). Predicting direction of stock price index movement using artificial neural networks and support vector machines: The sample of the Istanbul Stock Exchange. Expert Systems with Applications, (38), 5311-5319.
- Kılıç, S., (2015). Kappa testi. Journal of Mood Disorders, 5(3), 142-144.
- Li, A., Wu, J., & Liu, Z. (2017). Market manipulation detection based on classification methods. Procedia Computer Science, (122), 788-795.
- Liang, D., Tsai, C. F. & Wu, H. T. (2015). The effect of feature selection on financial distress prediction. Knowledge-Based Systems, (73), 289-297
- Nair, B. B., Mohandas, V., & Sakthivel, N. R. (2010). A Decision tree- rough set hybrid system for stock market trend prediction. International Journal of Computer Applications, 6(9), 1-6.
- Oliveira, F. A., Nobre, C. N., & Zárate, L. E. (2013). Applying artificial neural networks to prediction of stock price and improvement of the directional prediction index – Case study of PETR4, Petrobras, Brazil. Expert Systems with Applications, (40), 7596-7606.
- Özçalıcı, M. (2017). Aşırı öğrenme makineleri ile hisse senedi fiyat tahmini. Hacettepe Üniversitesi İktisadi ve İdari Bilimler Fakültesi Dergisi, 35(1), 67-88.
- Özdemir, A. K., Tolun, S., & Demirci, E. (2011). Endeks getirisi yönünün ikili sınıflandırma yöntemiyle tahmin edilmesi: İMKB 100 endeksi örneği. Niğde Üniversitesi İİBF Dergisi, 4(2), 45-59.
- Özkan, Y. (2016). Veri madenciliği yöntemleri, İstanbul: Papatya Yayınları.
- Öztemel, E. (2012). Yapay sinir ağları. İstanbul: Papatya Yayınları.
- Papuçcu, H. (2019). Borsa endeksi hareketlerinin makine öğrenme algoritmaları ile tahmini. Uluslararası İktisadi ve İdari İncelemeler Dergisi, (23), 179-190.
- Patel, J., Shah, S., Thakkar, P., & Kotecha, K. (2015). Predicting stock and stock price index movement using trend deterministic data preparation and machine learning techniques. Expert Systems with Applications, (42), 259- 268.
- Silahtaroğlu, G. (2016). Veri madenciliği kavram ve algoritmaları. İstanbul: Papatya Yayınları.
- Ulusoy, T. (2010). İMKB endeks öngörüsü için ileri beslemeli ağ mimarisine sahip yapay sinir ağı modellemesi. Uluslararası İktisadi ve İdari İncelemeler Dergisi, (5), 21-40.
- Yakut, E., & Gemici, E. (2017). LR, C5.0, CART, SVM yöntemlerini kullanarak hisse senedi getiri sınıflandırma tahmini yapılması ve kullanılan yöntemlerin karşılaştırılması: Türkiye’de BIST’de bir uygulama. Ege Akademik Bakış, 17(4), 461-479.
- https://tr.investing.com (Erişim Tarihi, 25.06.2020).
- https://www.kap.org.tr (Erişim Tarihi, 26.06.2020).
APA | AKSOY B (2021). Pay Senedi Fiyat Yönünün Makine Öğrenmesi Yöntemleri ile Tahmini: Borsa İstanbul Örneği. , 89 - 110. 10.20409/berj.2021.312 |
Chicago | AKSOY BARIS Pay Senedi Fiyat Yönünün Makine Öğrenmesi Yöntemleri ile Tahmini: Borsa İstanbul Örneği. (2021): 89 - 110. 10.20409/berj.2021.312 |
MLA | AKSOY BARIS Pay Senedi Fiyat Yönünün Makine Öğrenmesi Yöntemleri ile Tahmini: Borsa İstanbul Örneği. , 2021, ss.89 - 110. 10.20409/berj.2021.312 |
AMA | AKSOY B Pay Senedi Fiyat Yönünün Makine Öğrenmesi Yöntemleri ile Tahmini: Borsa İstanbul Örneği. . 2021; 89 - 110. 10.20409/berj.2021.312 |
Vancouver | AKSOY B Pay Senedi Fiyat Yönünün Makine Öğrenmesi Yöntemleri ile Tahmini: Borsa İstanbul Örneği. . 2021; 89 - 110. 10.20409/berj.2021.312 |
IEEE | AKSOY B "Pay Senedi Fiyat Yönünün Makine Öğrenmesi Yöntemleri ile Tahmini: Borsa İstanbul Örneği." , ss.89 - 110, 2021. 10.20409/berj.2021.312 |
ISNAD | AKSOY, BARIS. "Pay Senedi Fiyat Yönünün Makine Öğrenmesi Yöntemleri ile Tahmini: Borsa İstanbul Örneği". (2021), 89-110. https://doi.org/10.20409/berj.2021.312 |
APA | AKSOY B (2021). Pay Senedi Fiyat Yönünün Makine Öğrenmesi Yöntemleri ile Tahmini: Borsa İstanbul Örneği. Business and Economics Research Journal, 12(1), 89 - 110. 10.20409/berj.2021.312 |
Chicago | AKSOY BARIS Pay Senedi Fiyat Yönünün Makine Öğrenmesi Yöntemleri ile Tahmini: Borsa İstanbul Örneği. Business and Economics Research Journal 12, no.1 (2021): 89 - 110. 10.20409/berj.2021.312 |
MLA | AKSOY BARIS Pay Senedi Fiyat Yönünün Makine Öğrenmesi Yöntemleri ile Tahmini: Borsa İstanbul Örneği. Business and Economics Research Journal, vol.12, no.1, 2021, ss.89 - 110. 10.20409/berj.2021.312 |
AMA | AKSOY B Pay Senedi Fiyat Yönünün Makine Öğrenmesi Yöntemleri ile Tahmini: Borsa İstanbul Örneği. Business and Economics Research Journal. 2021; 12(1): 89 - 110. 10.20409/berj.2021.312 |
Vancouver | AKSOY B Pay Senedi Fiyat Yönünün Makine Öğrenmesi Yöntemleri ile Tahmini: Borsa İstanbul Örneği. Business and Economics Research Journal. 2021; 12(1): 89 - 110. 10.20409/berj.2021.312 |
IEEE | AKSOY B "Pay Senedi Fiyat Yönünün Makine Öğrenmesi Yöntemleri ile Tahmini: Borsa İstanbul Örneği." Business and Economics Research Journal, 12, ss.89 - 110, 2021. 10.20409/berj.2021.312 |
ISNAD | AKSOY, BARIS. "Pay Senedi Fiyat Yönünün Makine Öğrenmesi Yöntemleri ile Tahmini: Borsa İstanbul Örneği". Business and Economics Research Journal 12/1 (2021), 89-110. https://doi.org/10.20409/berj.2021.312 |