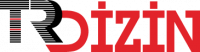
Psikiyatride Makine Öğrenmesi Yöntemlerinin Kullanımı
Yıl: 2021 Cilt: 13 Sayı: 2 Sayfa Aralığı: 332 - 353 Metin Dili: Türkçe DOI: 10.18863/pgy.779987 İndeks Tarihi: 29-07-2022
Psikiyatride Makine Öğrenmesi Yöntemlerinin Kullanımı
Öz: Yapay zeka ve veri analizinde gün geçtikçe daha popüler hale gelen makine öğrenmesi yöntemleri birçok farklı alanda veriden öğrenmeyi sağlamaktadır. Sağlık alanında yapılan çalışmalarda bu yöntemler sağlık çalışanlarına ve hekimlere destek sunmaktadır. Psikiyatri de bu alanlardan bir tanesidir. Hastalıkların tanı, hastalık seyrinin tahmini veya bir tedaviye verilecek yanıtın gözlemlenmesi gibi problemlere makine öğrenmesi yöntemleri destek sağlamaktadır. Bu çalışma kapsamında psikiyatri alanında yapılmış olan makine öğrenmesi çalışmaları incelenmiştir. Çalışmanın amacı, makine öğrenmesi yöntemlerinin psikiyatri alanında kullanımının araştırılmasıdır. Özellikle elektroensefalografi (EEG) verisi kullanılan araştırmalara odaklanılmıştır. Bu amaçla, psikiyatride alanında yapılan makine öğrenmesi ile ilgili olan SCOPUS ve Google Scholar kaynaklarındaki yayınlar incelenmiştir. Literatürdeki genel durumun ortaya konması amacıyla, psikiyatri alanında makine öğrenmesi yöntemlerinden yararlanan çalışmalara incelenmiştir. Sonrasında ise daha detaylı bir şekilde psikiyatri alanında makine öğrenmesi ve EEG verisi kullanılarak yapılan araştırmalar incelenmiştir. Bu çalışmanın psikiyatride makine öğrenmesi ile ilgili yapılan yayınlar ve özellikle EEG verisi kullanılan yayınların derlenmesi açısından araştırmacılara faydalı olabileceği umulmaktadır.
Anahtar Kelime: Use of Machine Learning Methods in Psychiatry
Öz: Machine learning methods, which are becoming more and more popular in artificial intelligence and data analysis, provide learning from data in many different fields. In the studies conducted in the field of health, these methods support healthcare professionals and physicians. Psychiatry is one of these areas. Machine learning methods provide support to problems such as diagnosis, prediction of disease course or monitoring response to a treatment. In this study, machine learning studies in the field of psychiatry are examined.The aim of the study is to examine the studies of machine learning in the field of psychiatry and especially the studies conducted using electroencephalography (EEG) data. Accordingly, studies on machine learning in the field of psychiatry in SCOPUS and Google Scholar sources were examined. In order to reveal the general situation in the literature, studies using machine learning methods in the field of psychiatry were examined. Afterwards, studies using both machine learning methods and EEG data in psychiatry were examined. It is hoped that this study will be useful to researchers in terms of the publications about machine learning in psychiatry and especially the publications using EEG data.
Anahtar Kelime: Belge Türü: Makale Makale Türü: Derleme Erişim Türü: Erişime Açık
- Ahmadlou M, Rostami R, Sadeghi V (2012) Which attention-deficit/hyperactivity disorder children will be improved through neurofeedback therapy? A graph theoretical approach to neocortex neuronal network of ADHD. Neurosci Lett, 516:156-160.
- Al-Kaysi AM, Al-Ani A, Loo CK, Breakspear M, Boonstra TW (2016) Predicting brain stimulation treatment outcomes of depressed patients through the classification of EEG oscillations. Annu Int Conf IEEE Eng Med Biol Soc: 5266-5269. 2016 38th Annual International Conference of the IEEE Engineering in Medicine and Biology Society (EMBC)
- Bailey NW, Hoy KE, Rogasch NC, Thomson RH, McQueen S, Elliot D et al. (2018) Responders to rTMS for depression show increased fronto-midline theta and theta connectivity compared to non-responders. Brain Stimul, 11:190-203.
- Balaban ME, Kartal E (2018) Veri Madencilği ve Makine Öğrenmesi Temel Algoritmaları ve R Dili ile Uygulamaları (2 baskı.). İstanbul, Çağlayan Kitapevi.
- Barzilay R, Israel N, Krivoy A, Sagy R, Kamhi-Nesher S, Loebstein O et al. (2019) Predicting affect classification in mental status examination using machine learning face action recognition system: a pilot study in schizophrenia patients. Front Psychiatry, 10:288.
- Bedi G, Carrillo F, Cecchi GA, Slezak DF, Sigman M, Mota NB et al. (2015) Automated analysis of free speech predicts psychosis onset in high-risk youths. NPJ Schizophr, 1:15030.
- Bishop, C (2006). Pattern Recognition and Machine Learning. Information Science and Statistics. New York, Springer-Verlag.
- Brodersen, KH, Deserno, L, Schlagenhauf, F, Zhihao, L, Penny, WD, Buhmann, JM ve Stephan, KE (2014). Dissecting psychiatric spectrum disorders by generative embedding. Neuroimage Clin, 4:98-111.
- Cao H, Meyer-Lindenberg A, Schwarz E (2018) Comparative evaluation of machine learning strategies for analyzing big data in psychiatry. Int J Mol Sci, 19:3387.
- Dipnall J F, Pasco J A, Berk M, Williams LJ, Dodd S, Jacka FN et al. (2017) Why so GLUMM? Detecting depression clusters through graphing lifestyle-environs using machine-learning methods (GLUMM). Eur Psychiatry, 39:40-50.
- Dowd EC, Frank MJ, Collins A, Gold JM, Barch DM (2016) Probabilistic reinforcement learning in patients with schizophrenia: relationships to anhedonia and avolition. Biol Psychiatry Cogn Neurosci Neuroimaging, 1:460-473.
- Edgcomb J, Shaddox T, Hellemann G, Brooks JO (2019) High-risk phenotypes of early psychiatric readmission in bipolar disorder with comorbid medical ıllness. Psychosomatics, 60:563-573.
- Efron B, Tibshirani R (1993) An Introduction to the Bootstrap. New York, Chapman & Hall.
- Ellis RJ, Wang Z, Genes N, Ma’ayan A (2019) Predicting opioid dependence from electronic health records with machine learning. BioData Mining, 12:3.
- Ergüzel TT, Noyan CO, Eryılmaz G, Ünsalver BÖ, Cebi M, Tas C et al. (2019) Binomial logistic regression and artificial neural network methods to classify opioid-dependent subjects and control group using quantitative EEG power measures. Clin EEG Neurosci, 50:303-310.
- Ergüzel TT, Özekes S, Gültekin S, Tarhan N, Hızlı Sayar G, Bayram A (2015a) Neural network based response prediction of rTMS in major depressive disorder using QEEG cordance. Psychiatry Investig, 12:61-65.
- Ergüzel TT, Özekes S, Sayar GH, Tan O, Tarhan N (2015b) A hybrid artificial intelligence method to classify trichotillomania and obsessive compulsive disorder. Neurocomputing, 161:220-228.
- Ergüzel TT, Taş C, Çebi M (2015c) A wrapper-based approach for feature selection and classification of major depressive disorder– bipolar disorders. Comput Biol Med, 64:127-137.
- Ergüzel TT, Tarhan N (2018) Machine learning approaches to predict repetitive transcranial magnetic stimulation treatment response in major depressive disorder. In Proceedings of SAI Intelligent Systems Conference (IntelliSys) 2016 (Eds Y Bi, S Kapoor, R Bhatia):391-401. Cham: Springer International Publishing.
- Eugene A R, Masiak J, Eugene B (2018) Predicting lithium treatment response in bipolar patients using gender-specific gene expression biomarkers and machine learning. F1000Res, 7:474.
- Flach P (2012). Machine Learning: The Art and Science of Algorithms That Make Sense of Data. Cambridge, Cambridge University Press.
- Fond G, Bulzacka E, Boucekine M, Schürhoff F, Berna F, Godin O et al. (2019) Machine learning for predicting psychotic relapse at 2years in schizophrenia in the national FACE-SZ cohort. Prog Neuropsychopharmacol Biol Psychiatry, 92:8-18.
- Galatzer-Levy IR, Karstoft, K-I, Statnikov A, Shalev AY (2014) Quantitative forecasting of PTSD from early trauma responses: A Machine Learning application. J Psychiatr Res, 59:68-76.
- Han D-H, Lee S, Seo DC (2020) Using machine learning to predict opioid misuse among U.S. adolescents. Prev Med, 130:105886.
- Han J, Kamber M, Pei J (2012) Data Mining: Concepts and Techniques (3rd edition.). New York, Morgan Kaufman Publishers.
- Hatton CM, Paton L W, McMillan D, Cussens J, Gilbody S, Tiffin PA (2019) Predicting persistent depressive symptoms in older adults: A machine learning approach to personalised mental healthcare. J Affect Disord, 246:857-860.
- Hosseinifard B, Moradi MH, Rostami,R (2013) Classifying depression patients and normal subjects using machine learning techniques and nonlinear features from EEG signal. Comput Methods Programs Biomed, 109:339-345.
- Johannesen JK, Bi J, Jiang R, Kenney JG, Chen C-M A (2016) Machine learning identification of EEG features predicting working memory performance in schizophrenia and healthy adults. Neuropsychiatr Electrophysiol, 2:3.
- Just MA, Pan L, Cherkassky VL, McMakin DL, Cha C, Nock MK et al. (2017) Machine learning of neural representations of suicide and emotion concepts identifies suicidal youth. Nat Hum Behav, 1:911-919.
- Karstoft K-I, Galatzer-Levy IR, Statnikov A, Li Z, Shalev AY, For members of the Jerusalem Trauma Outreach and Prevention Study (J-TOPS) group (2015) Bridging a translational gap: Using machine learning to improve the prediction of PTSD. BMC Psychiatry, 15:30.
- Khodayari-Rostamabad A, Hasey GM, MacCrimmon DJ, Reilly JP, Bruin H de (2010a) A pilot study to determine whether machine learning methodologies using pre-treatment electroencephalography can predict the symptomatic response to clozapine therapy. Clin Neurophysiol, 121:1998-2006.
- Khodayari-Rostamabad A, Reilly JP, Hasey G, de Bruin H, MacCrimmon D (2010b) Using pre-treatment EEG data to predict response to SSRI treatment for MDD. Annu Int Conf IEEE Eng Med Biol Soc:6103-6106. 2010 Annual International Conference of the IEEE Engineering in Medicine and Biology, Buenos Aires, Arjantin.
- Khodayari-Rostamabad A, Reilly JP, Hasey GM, de Bruin H, MacCrimmon D (2011) Using pre-treatment electroencephalography data to predict response to transcranial magnetic stimulation therapy for major depression. Annu Int Conf IEEE Eng Med Biol Soc: 6418- 6421. 2011 Annual International Conference of the IEEE Engineering in Medicine and Biology Society, Boston, IEEE.
- Khodayari-Rostamabad A, Reilly JP, Hasey GM, de Bruin H, Maccrimmon DJ (2013) A machine learning approach using EEG data to predict response to SSRI treatment for major depressive disorder. Clin Neurophysiol, 124:1975-1985.
- Kohavi R (1995). A Study of Cross-Validation and Bootstrapfor Accuracy Estimation and Model Selection. Proceedings of the 14th International Joint Conference on Artificial Intelligence:1137–1145. International Joint Conference on Artificial Intelligence.
- Kuang D, He L (2014) Classification on ADHD with Deep Learning. 2014 International Conference on Cloud Computing and Big Data: 27-32. 2014 International Conference on Cloud Computing and Big Data, Wuhan, China: IEEE.
- Kutlu Y (2010) Modelden bağımsız yöntemler kullanılarak EEG ve EKG içindeki anormal örüntülerin çok katlı sınıflandırılması. (Doktora tezi). İzmir, Dokuz Eylül Üniversitesi.
- Li Y, Rosenfeld B, Pessin H, Breitbart W (2017) Bayesian Nonparametric Clustering of Patients with Advanced Cancer on Anxiety and Depression. 2017 16th IEEE International Conference on Machine Learning and Applications (ICMLA):674-678. 2017 16th IEEE International Conference on Machine Learning and Applications (ICMLA), Cancun, Meksika, IEEE.
- Mellem MS, Liu Y, Gonzalez H, Kollada M, Martin WJ, Ahammad P (2020) Machine learning models ıdentify multimodal measurements highly predictive of transdiagnostic symptom severity for mood, anhedonia, and anxiety. Biol Psychiatry Cogn Neurosci Neuroimaging, 5(1):56-67.
- Mohammadi M, Al-Azab F, Raahemi B, Richards G, Jaworska N, Smith D et al. (2015) Data mining EEG signals in depression for their diagnostic value. BMC Med Inform Decis Mak, 15:108.
- Mohri M, Rostamizadeh A, Talwalkar A (2012) Foundations of Machine Learning. Boston, MIT Press. Mosteller F, Tukey J (1968) Data analysis, including statistics. Handbook of Social Psychology, vol. 2 (Eds G Lindzey, E Aronson):80-203. Addison Wesley.
- Mueller A, Candrian G, Kropotov J D, Ponomarev V A, Baschera G-M (2010) Classification of ADHD patients on the basis of independent ERP components using a machine learning system. Nonlinear Biomed Phys, 4(Suppl 1): S1.
- Mumtaz W, Xia L, Ali SSA, Yasin MAM, Hussain M, Malik AS (2017a) Electroencephalogram (EEG)-based computer-aided technique to diagnose major depressive disorder (MDD). Biomed Signal Process Control, 31:108-115.
- Mumtaz W, Xia L, Yasin MAM, Ali SSA, Malik AS (2017b) A wavelet-based technique to predict treatment outcome for major depressive disorder. PLoS One, 12:e0171409.
- Mwangi B, Wu M-J, Cao B, Passos IC, Lavagnino L, Keser Z et al. (2016) Individualized prediction and clinical staging of bipolar disorders using neuroanatomical biomarkers. Biol Psychiatry Cogn Neurosci Neuroimaging, 1:186-194.
- Nouretdinov I, Costafreda SG, Gammerman A, Chervonenkis A, Vovk V, Vapnik V et al. (2011) Machine learning classification with confidence: Application of transductive conformal predictors to MRI-based diagnostic and prognostic markers in depression. Neuroimage, 56:809-813.
- Öztoprak H, Toycan M, Alp YK, Arıkan O, Doğutepe E, Karakaş S (2017) Machine-based classification of ADHD and nonADHD participants using time/frequency features of event-related neuroelectric activity. Clin Neurophysiol, 128:2400-2410.
- Papini S, Pisner D, Shumake J, Powers MB, Beevers CG, Rainey EE et al. (2018) Ensemble machine learning prediction of posttraumatic stress disorder screening status after emergency room hospitalization. J Anxiety Disord, 60:35-42.
- Perez Arribas I, Goodwin G M, Geddes JR, Lyons T, Saunders KEA (2018) A signature-based machine learning model for distinguishing bipolar disorder and borderline personality disorder. Transl Psychiatry, 8:274.
- Perlis RH, Iosifescu DV, Castro VM, Murphy SN, Gainer VS, Minnier J et al. (2012) Using electronic medical records to enable large-scale studies in psychiatry: Treatment resistant depression as a model. Psychol Med, 42:41-50.
- Perlis R (2013) A clinical risk stratification tool for predicting treatment resistance in major depressive disorder. Biol Psychiatry, 74:7-14.
- Pinaya WHL, Mechelli A, Sato JR (2019) Using deep autoencoders to identify abnormal brain structural patterns in neuropsychiatric disorders: A large‐scale multi‐sample study. Hum Brain Mapp, 40:944-954.
- Qin S, Young C B, Duan X, Chen T, Supekar K, Menon V (2014) Amygdala subregional structure and intrinsic functional connectivity predicts individual differences in anxiety during early childhood. Biol Psychiatry, 75:892-900.
- Ramyead A, Studerus E, Kometer M, Uttinger M, Gschwandtner U, Fuhr P et al. (2016) Prediction of psychosis using neural oscillations and machine learning in neuroleptic-naïve at-risk patients. World J Biol Psychiatry, 17:285-295.
- Redlich R, Opel N, Grotegerd D, Dohm K, Zaremba D, Burger C et al. (2016) Prediction of individual response to electroconvulsive therapy via machine learning on structural magnetic resonance imaging data. JAMA Psychiatry, 73:557-564.
- Sato JR, Biazoli C E, Salum G A, Gadelha A, Crossley N, Vieira G et al. (2018) Association between abnormal brain functional connectivity in children and psychopathology: A study based on graph theory and machine learning. World J Biol Psychiatry, 19:119-129.
- Stamate D, Katrinecz A, Stahl D, Verhagen SJW, Delespaul PAEG, van Os J et al. (2019) Identifying psychosis spectrum disorder from experience sampling data using machine learning approaches. Schizophr Res, 209:156-163.
- Sohn S, Kocher J.-P A, Chute C G, Savova G K (2011) Drug side effect extraction from clinical narratives of psychiatry and psychology patients. J Am Med Inform Assoc, 18(Suppl 1):144-149.
- Stone M (1974) Cross-validatory choice and assessment of statistical predictions. J R Stat Soc Series B Stat Methodol, 36:111–147.
- Suhasini A, Palanivel S, Ramalingam V (2011) Multimodel decision support system for psychiatry problem. Expert Syst Appl, 38:4990- 4997.
- Tenev A, Markovska-Simoska S, Kocarev L, Pop-Jordanov J, Müller A, Candrian G (2014) Machine learning approach for classification of ADHD adults. Int J Psychophysiol, 93:162-166.
- Viviano JD, Buchanan R W, Calarco N, Gold JM, Foussias G, Bhagwat N et al. (2018) Resting-state connectivity biomarkers of cognitive performance and social function in ındividuals with schizophrenia spectrum disorder and healthy control subjects. Biol Psychiatry, 84:665-674.
- Walsh CG, Ribeiro JD, Franklin JC (2018) Predicting suicide attempts in adolescents with longitudinal clinical data and machine learning. J Child Psychol Psychiatry, 59:1261-1270.
- Walss-Bass C, Suchting R, Olvera RL, Williamson DE (2018) Inflammatory markers as predictors of depression and anxiety in adolescents: statistical model building with component-wise gradient boosting. J Affect Disord, 234:276-281.
- Yoon JH, Nguyen DV, McVay LM, Deramo P, Minzenberg MJ, Ragland JD et al. (2012) Automated classification of fMRI during cognitive control identifies more severely disorganized subjects with schizophrenia. Schizophr Res, 135:28-33.
- Zhang X, Hu B, Zhou L, Moore P, Chen J. (2013) An EEG based pervasive depression detection for females. In Pervasive Computing and the Networked World, Lecture Notes in Computer Science 7719 (Eds Q Zu, B Hu, A Elçi): 848-861. Berlin, Springer.
- Zhao K, So HC (2019) Drug repositioning for schizophrenia and depression/anxiety disorders: a machine learning approach leveraging expression data. IEEE J Biomed Health Inform, 23:1304-1315.
- Zhao S, Zhao Q, Zhang X, Peng H, Yao Z, Shen J et al. (2017) Wearable EEG-based real-time system for depression monitoring. In Brain Informatics. BI 2017. Lecture Notes in Computer Science, vol 10654 (Eds Y Zeng, Y He, JH Kotaleski, M Martone, B Xu, H Peng et al.):190-201. Cham: Springer.
- Zilcha-Mano S, Roose SP, Brown PJ ve Rutherford BR (2018) A machine learning approach to ıdentifying placebo responders in late-life depression trials. Am J Geriatr Psychiatry, 26:669-677.
APA | Emre İ, Tas C, Erol C (2021). Psikiyatride Makine Öğrenmesi Yöntemlerinin Kullanımı. , 332 - 353. 10.18863/pgy.779987 |
Chicago | Emre İlkim Ecem,Tas Cumhur,Erol Cigdem Psikiyatride Makine Öğrenmesi Yöntemlerinin Kullanımı. (2021): 332 - 353. 10.18863/pgy.779987 |
MLA | Emre İlkim Ecem,Tas Cumhur,Erol Cigdem Psikiyatride Makine Öğrenmesi Yöntemlerinin Kullanımı. , 2021, ss.332 - 353. 10.18863/pgy.779987 |
AMA | Emre İ,Tas C,Erol C Psikiyatride Makine Öğrenmesi Yöntemlerinin Kullanımı. . 2021; 332 - 353. 10.18863/pgy.779987 |
Vancouver | Emre İ,Tas C,Erol C Psikiyatride Makine Öğrenmesi Yöntemlerinin Kullanımı. . 2021; 332 - 353. 10.18863/pgy.779987 |
IEEE | Emre İ,Tas C,Erol C "Psikiyatride Makine Öğrenmesi Yöntemlerinin Kullanımı." , ss.332 - 353, 2021. 10.18863/pgy.779987 |
ISNAD | Emre, İlkim Ecem vd. "Psikiyatride Makine Öğrenmesi Yöntemlerinin Kullanımı". (2021), 332-353. https://doi.org/10.18863/pgy.779987 |
APA | Emre İ, Tas C, Erol C (2021). Psikiyatride Makine Öğrenmesi Yöntemlerinin Kullanımı. Psikiyatride Güncel Yaklaşımlar, 13(2), 332 - 353. 10.18863/pgy.779987 |
Chicago | Emre İlkim Ecem,Tas Cumhur,Erol Cigdem Psikiyatride Makine Öğrenmesi Yöntemlerinin Kullanımı. Psikiyatride Güncel Yaklaşımlar 13, no.2 (2021): 332 - 353. 10.18863/pgy.779987 |
MLA | Emre İlkim Ecem,Tas Cumhur,Erol Cigdem Psikiyatride Makine Öğrenmesi Yöntemlerinin Kullanımı. Psikiyatride Güncel Yaklaşımlar, vol.13, no.2, 2021, ss.332 - 353. 10.18863/pgy.779987 |
AMA | Emre İ,Tas C,Erol C Psikiyatride Makine Öğrenmesi Yöntemlerinin Kullanımı. Psikiyatride Güncel Yaklaşımlar. 2021; 13(2): 332 - 353. 10.18863/pgy.779987 |
Vancouver | Emre İ,Tas C,Erol C Psikiyatride Makine Öğrenmesi Yöntemlerinin Kullanımı. Psikiyatride Güncel Yaklaşımlar. 2021; 13(2): 332 - 353. 10.18863/pgy.779987 |
IEEE | Emre İ,Tas C,Erol C "Psikiyatride Makine Öğrenmesi Yöntemlerinin Kullanımı." Psikiyatride Güncel Yaklaşımlar, 13, ss.332 - 353, 2021. 10.18863/pgy.779987 |
ISNAD | Emre, İlkim Ecem vd. "Psikiyatride Makine Öğrenmesi Yöntemlerinin Kullanımı". Psikiyatride Güncel Yaklaşımlar 13/2 (2021), 332-353. https://doi.org/10.18863/pgy.779987 |