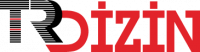
Derin öğrenme kullanılarak mobil cihazlar için gerçek zamanlı yangın ve duman tespiti
Yıl: 2023 Cilt: 38 Sayı: 4 Sayfa Aralığı: 2179 - 2190 Metin Dili: Türkçe DOI: 10.17341/gazimmfd.1041091 İndeks Tarihi: 29-09-2023
Derin öğrenme kullanılarak mobil cihazlar için gerçek zamanlı yangın ve duman tespiti
Öz: Yangın; ekolojik, sosyal ve ekonomik zararlara neden olan doğal afettir. Küresel ısınma ve patlayıcı/yanıcı kimyasalların kullanımının yaygınlaşması ile birlikte yangınlar insanlık için en önemli sorunlardan biri haline gelmiştir. Yangınların erken tespiti tahribatı en aza indirmek için kritik öneme sahiptir. Binalarda yangınların erken tespit edilmesini sağlayabilmek için ısı ve duman dedektörleri geliştirilmiştir. Ancak bu dedektörlerin kullanımı kapalı alanlar ile kısıtlıdır. Geniş alanlarda bu detektörlerin kullanılması mümkün değildir. Ayrıca bu dedektörler kurulum ve bakım açısından oldukça maliyetlidir. Bu nedenlerden dolayı görüntülerden yangın tespiti üzerine çalışmalar yapılmaya başlanmıştır. Görüntülerden yangın tespiti için yapılan son çalışmalarda genellikle derin öğrenme algoritmaları kullanılmaktadır. Bu çalışmalar kameralar üzerinden alınan görüntülerin güçlü sunucular üzerinde çalışan modeller ile analizine odaklanmaktadır. Mobil cihazlar ve nesnelerin internetindeki gelişmeler ile artık uç cihazlar üzerinde görüntüler analiz edilebilir. Yapılan çalışmada görüntülerin bir sunucuya aktarılmadan mobil cihaz üzerinde analiz edilmesini sağlayacak düşük işlem gücü gerektiren yangın ve duman tespit modeli geliştirilmiştir. MobileNet evrişimsel sinir ağı revize edilerek son 3 katman kaldırılmış ve yerine düzleştirme katmanı ile iki düğümden oluşan yoğun katman eklenmiştir. Yangın ve duman tespiti modeli için revize edilmiş MobileNet, orijinal MobileNet, MobileNetV2, EfficientNetB0 ve ShuffleNet evrişimsel sinir ağları kullanılarak geliştirilen modeller arasından en yüksek doğruluk oranına sahip yöntem belirlenmiştir. Modellerin başarı oranını artırabilmek ImageNet üzerinde ön eğitilmiş model ağırlıkları transfer öğrenme tekniği ile tekrar kullanılmıştır. Modelleri eğitmek ve test etmek için 43,355 görüntüden oluşan veri seti kullanılmıştır. Veri setinin %80’i eğitim %20’si test işlemi için kullanılmıştır. Yapılan test sonuçlarına göre revize edilmiş MobileNet ağı %98,37 ile en yüksek doğruluk oranına ulaşılmıştır.
Anahtar Kelime: Real-time fire and smoke detection for mobile devices using deep learning
Öz: Fire is a natural disaster that causes ecological, social and economic damage. With global warming and the widespread use of explosive/flammable chemicals, fires have become one of the most important problems for humanity. Early fire detection is critical to minimize destruction. For this reason, studies on fire detection through images have begun to be carried out in order to detect fires early. In recent studies for fire detection from images deep learning algorithms are generally used. These studies focus on the analysis of images taken from cameras with models running on powerful servers. With the developments in mobile devices and internet of things, images can analyzed on edge devices. In the study fire and smoke detection model which requires low processing power has been developed to enable the images to be analyzed on mobile device without transferring them to server. The MobileNet convolutional neural network was revised, the last 3 layers were removed and replaced with a flattening layer and a dense layer consisting of two nodes. Fire and smoke detection model, the method with the highest accuracy was determined among the models developed using revised MobileNet, original MobileNet, MobileNetV2, EfficientNetB0, ShuffleNet, NASNetMobile and PeleeNet convolutional neural networks. To train and test the models, 80% of the dataset consisting of 43,355 images was used for training and 20% for testing. According to test results, highest accuracy rate of 98.37% was achieved in the revised MobileNet.
Anahtar Kelime: Belge Türü: Makale Makale Türü: Araştırma Makalesi Erişim Türü: Erişime Açık
- 1. Brushlinsky N. N., Ahrens M., Sokolov S. V., Wagner P. (2021), World Fire Statistics, CTIF World Fire Statistics Center, https://www.statista.com/statistics/1264713/area-burned-by-wildfire-in- turkey/, Erişim tarihi Nisan 24, 2022.
- 2. N. Sönnichsen, Land burned by wildfires in Turkey 2009-2021, https://www.statista.com/statistics/1264713/area-burned-by-wildfire-in- turkey/, Erişim tarihi Nisan 24, 2022.
- 3. Bu F., Gharajeh M.S., Intelligent and vision-based fire detection systems: A survey, Image and Vision Computing, 91, 2019.
- 4. Geetha S., Abhishek C.S., Akshayanat C.S., Machine Vision Based Fire Detection Techniques: A Survey, Fire Technol, 57, 591-623, 2021.
- 5. Şafak E., Arslan Ç., Gözütok M., Köprülü T., Dağıtık Defter Teknolojileri ve Uygulama Alanları Üzerine Bir İnceleme, Avrupa Bilim ve Teknoloji Dergisi, 29, 36-45, 2021.
- 6. Utku, A., Doğru, İ.A., Permission based detection system for android malware, Journal of the Faculty of Engineering and Architecture of Gazi University, 32 (4), 1015-1024, 2017.
- 7. Öcal, H., Doğru, İ.A., Barışçı, N., Akıllı ve Geleneksel Giyilebilir Sağlık Cihazlarında Nesnelerin İnterneti, Politeknik Dergisi, (3), 695- 714, 2019.
- 8. Li P., Zhao W., Image fire detection algorithms based on convolutional neural networks, Case Studies in Thermal Engineering, 19, 2020.
- 9. Li C., Yang B., Ding H., Shi H., Jiang X., Sun J., Real-time video-based smoke detection with high accuracy and efficiency, Fire Safety Journal, 117, 2020.
- 10. Xu R., Lin H., Lu K., Cao L., Liu Y., A Forest Fire Detection System Based on Ensemble Learning, 12 (2), 2021.
- 11. He L., Gong X., Zhang S., Wang L., Li F., Efficient attention based deep fusion CNN for smoke detection in fog environment, Neurocomputing, 434, 224-238, 2021.
- 12. Muhammad K., Ahmad J., Mehmood I., Rho S., Baik S.W., Convolutional Neural Networks Based Fire Detection in Surveillance Videos, IEEE, 6, 18174-18183, 2018.
- 13. Majid S., Alenezi F., Masood S., Ahmad M., Gündüz E.S., Polat K., Attention based CNN model for fire detection and localization in real- world images, Expert Systems with Applications, 189, 2022.
- 14. Huang L., Liu G., Wang Y., Yuan H., Chen T., Fire detection in video surveillances using convolutional neural networks and wavelet transform, Engineering Applications of Artificial Intelligence, 110, 2022.
- 15. Zheng X., Chen F., Lou L., Cheng P., Huang Y., Real-Time Detection of Full-Scale Forest Fire Smoke Based on Deep Convolution Neural Network, Remote Sensing, 14 (3), 536, 2022.
- 16. Şafak E., Barışçı N., Age and Gender Prediction Using Convolutional Neural Networks, 2018 2nd International Symposium on Multidisciplinary Studies and Innovative Technologies (ISMSIT), Ankara, 2018.
- 17. Albawi S., Mohammed T. A., Al-Zawi S., Understanding of a convolutional neural network, International Conference on Engineering and Technology (ICET), 2017.
- 18. Arı A., Hanbay D., Tumor detection in MR images of regional convolutional neural networks, Journal of the Faculty of Engineering and Architecture of Gazi University, 34 (3), 1395-1408, 2019.
- 19. İnik, Ö., Ülker, E., Derin Öğrenme ve Görüntü Analizinde Kullanılan Derin Öğrenme Modelleri, Gaziosmanpaşa Bilimsel Araştirma Dergisi (GBAD), 6 (3), 85-104, 2017.
- 20. Cai S., Shu Y., Chen G., Ooi B.C., Wang W., Zhang M., Effective and Efficient Dropout for Deep Convolutional Neural Networks, CoRR, abs/1904.03392, 2020.
- 21. Zhang X., Lin T., Xu J., Luo X., Ying Y., ADeepSpectra: An end-to-end deep learning approach for quantitative spectral analysis, Analytica Chimica Acta, 48-57, 2019.
- 22. Krizhevsky A., Sutskever I., Hinton G., ImageNet classification with deep convolutional neural networks, NIPS’2012, 2012.
- 23. Khan A., Sohail A., Zahoora U., Qureshi A.S., A survey of the recent architectures of deep convolutional neural networks, 53, 5455–5516, 2020.
- 24. Fire Detection Dataset, https://mivia.unisa.it/datasets/video-analysis- datasets/fire-detection-dataset/. Erişim tarihi Aralık 20, 2021.
- 25. FIRESENSE, https://zenodo.org/record/836749#.YcDXMWhBzIV. Erişim tarihi Aralık 20, 2021.
- 26. Fire-Detection-Image-Dataset, https://github.com/cair/Fire-Detection- Image-Dataset. Erişim tarihi Aralık 20, 2021.
- 27. Rácz, A., Bajusz, D., Héberger, K., Effect of Dataset Size and Train/Test Split Ratios in QSAR/QSPR Multiclass Classification, Molecules, 26 (4), 2021.
- 28. Gülcü A., Kuş Z., Konvolüsyonel Sinir Ağlarında Hiper-Parametre Optimizasyonu Yöntemlerinin İncelenmesi, Gazi University Journal of Science Part C: Design and Technology, 7 (2), 503-522, 2019.
- 29. Zhang Z., Sabuncu M.R., Generalized Cross Entropy Loss for Training Deep Neural Networks with Noisy Labels, CoRR, abs/1805.07836v4, 2018.
- 30. Kingma D.P., Ba J.L., Adam: A Method for Stochastic Optimization, CoRR, abs/1412.6980v9, 2017.
- 31. Chen W., Gao L., Li X., Shen W., Lightweight convolutional neural network with knowledge distillation for cervical cells classification, Biomedical Signal Processing and Control, 71 (A), 103177, 2022.
- 32. Howard A. G., Zhu M., Chen B., Kalenichenko D., Wang W., Weyand T., Andreetto M., Adam H., MobileNets: Efficient Convolutional Neural Networks for Mobile Vision Applications, CoRR, abs/1704.04861, 2017.
- 33. Sandler M., Howard A., Zhu M., Zhmoginov A., Chen L.C., MobileNetV2: Inverted Residuals and Linear Bottlenecks, CoRR, abs/1801.04381, 2019.
- 34. Tan M., Le Q.V., EfficientNet: Rethinking Model Scaling for Convolutional Neural Networks, CoRR, abs/1905.11946, 2019.
- 35. Zhang X., Zhou X., Lin M., Sun J., ShuffleNet: An Extremely Efficient Convolutional Neural Network for Mobile Devices, CoRR, abs/1707.01083, 2017.
- 36. F. Saxen, P. Werner, S. Handrich, E. Othman, L. Dinges and A. Al- Hamadi, "Face Attribute Detection with MobileNetV2 and NasNet- Mobile," 2019 11th International Symposium on Image and Signal Processing and Analysis (ISPA), 176-180, 2019.
- 37. Wang R. J., Li X., Ling C. X., Pelee: A Real-Time Object Detection System on Mobile Devices, CoRR, abs/1804.06882v3, 2018.
- 38. Tensorflow. Tensorflow. https://www.tensorflow.org/. Erişim tarihi Aralık 20, 2021.
- 39. Janardhanan P., Project repositories for machine learning with TensorFlow, Procedia Computer Science, 188-196, 2020.
- 40. Şeker A., Diri B., Balık H.H., Derin Öğrenme Yöntemleri ve Uygulamaları Hakkında Bir İnceleme, Gazi Mühendislik Bilimleri Dergisi, 3 (3), 47-64, 2017.
- 41. Bozinovski S., Fulgosi A., The influence of pattern similarity and transfer of learning upon training of a base perceptron B2, Proc. Symp. Informatica 3-121-5, Bled, 1976.
- 42. Elmas B., Identifying species of trees through bark images by convolutional neural networks with transfer learning method, Journal of the Faculty of Engineering and Architecture of Gazi University, 36 (3), 1253-1270, 2021.
- 43. Zhuang F., Qi Z., Duan K., Xi D., Zhu Y., Zhu H., Xiong H., He Q., A Comprehensive Survey on Transfer Learning, IEEE, 109 (1), 43-76, 2021.
- 44. Fırıldak K., Talu M.F., Evrişimsel Sinir Ağlarında Kullanılan Transfer Öğrenme Yaklaşımlarının İncelenmesi, Journal of Computer Science, 4 (2), 88-95, 2019.
- 45. Bianco S., Cadene R., Celona L., Napoletano P., Benchmark Analysis of Representative Deep Neural Network Architectures, CoRR, abs/1810.00736v2, 2018.
APA | Şafak E, BARIŞÇI N (2023). Derin öğrenme kullanılarak mobil cihazlar için gerçek zamanlı yangın ve duman tespiti. , 2179 - 2190. 10.17341/gazimmfd.1041091 |
Chicago | Şafak Emre,BARIŞÇI Necaattin Derin öğrenme kullanılarak mobil cihazlar için gerçek zamanlı yangın ve duman tespiti. (2023): 2179 - 2190. 10.17341/gazimmfd.1041091 |
MLA | Şafak Emre,BARIŞÇI Necaattin Derin öğrenme kullanılarak mobil cihazlar için gerçek zamanlı yangın ve duman tespiti. , 2023, ss.2179 - 2190. 10.17341/gazimmfd.1041091 |
AMA | Şafak E,BARIŞÇI N Derin öğrenme kullanılarak mobil cihazlar için gerçek zamanlı yangın ve duman tespiti. . 2023; 2179 - 2190. 10.17341/gazimmfd.1041091 |
Vancouver | Şafak E,BARIŞÇI N Derin öğrenme kullanılarak mobil cihazlar için gerçek zamanlı yangın ve duman tespiti. . 2023; 2179 - 2190. 10.17341/gazimmfd.1041091 |
IEEE | Şafak E,BARIŞÇI N "Derin öğrenme kullanılarak mobil cihazlar için gerçek zamanlı yangın ve duman tespiti." , ss.2179 - 2190, 2023. 10.17341/gazimmfd.1041091 |
ISNAD | Şafak, Emre - BARIŞÇI, Necaattin. "Derin öğrenme kullanılarak mobil cihazlar için gerçek zamanlı yangın ve duman tespiti". (2023), 2179-2190. https://doi.org/10.17341/gazimmfd.1041091 |
APA | Şafak E, BARIŞÇI N (2023). Derin öğrenme kullanılarak mobil cihazlar için gerçek zamanlı yangın ve duman tespiti. Gazi Üniversitesi Mühendislik Mimarlık Fakültesi Dergisi, 38(4), 2179 - 2190. 10.17341/gazimmfd.1041091 |
Chicago | Şafak Emre,BARIŞÇI Necaattin Derin öğrenme kullanılarak mobil cihazlar için gerçek zamanlı yangın ve duman tespiti. Gazi Üniversitesi Mühendislik Mimarlık Fakültesi Dergisi 38, no.4 (2023): 2179 - 2190. 10.17341/gazimmfd.1041091 |
MLA | Şafak Emre,BARIŞÇI Necaattin Derin öğrenme kullanılarak mobil cihazlar için gerçek zamanlı yangın ve duman tespiti. Gazi Üniversitesi Mühendislik Mimarlık Fakültesi Dergisi, vol.38, no.4, 2023, ss.2179 - 2190. 10.17341/gazimmfd.1041091 |
AMA | Şafak E,BARIŞÇI N Derin öğrenme kullanılarak mobil cihazlar için gerçek zamanlı yangın ve duman tespiti. Gazi Üniversitesi Mühendislik Mimarlık Fakültesi Dergisi. 2023; 38(4): 2179 - 2190. 10.17341/gazimmfd.1041091 |
Vancouver | Şafak E,BARIŞÇI N Derin öğrenme kullanılarak mobil cihazlar için gerçek zamanlı yangın ve duman tespiti. Gazi Üniversitesi Mühendislik Mimarlık Fakültesi Dergisi. 2023; 38(4): 2179 - 2190. 10.17341/gazimmfd.1041091 |
IEEE | Şafak E,BARIŞÇI N "Derin öğrenme kullanılarak mobil cihazlar için gerçek zamanlı yangın ve duman tespiti." Gazi Üniversitesi Mühendislik Mimarlık Fakültesi Dergisi, 38, ss.2179 - 2190, 2023. 10.17341/gazimmfd.1041091 |
ISNAD | Şafak, Emre - BARIŞÇI, Necaattin. "Derin öğrenme kullanılarak mobil cihazlar için gerçek zamanlı yangın ve duman tespiti". Gazi Üniversitesi Mühendislik Mimarlık Fakültesi Dergisi 38/4 (2023), 2179-2190. https://doi.org/10.17341/gazimmfd.1041091 |