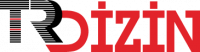
Çekişmeli üretken ağlarla Pap-Smear görüntüsü oluşturmada yeni bir yaklaşım
Yıl: 2022 Cilt: 37 Sayı: 3 Sayfa Aralığı: 1401 - 1410 Metin Dili: Türkçe DOI: 10.17341/gazimmfd.939092 İndeks Tarihi: 29-07-2022
Çekişmeli üretken ağlarla Pap-Smear görüntüsü oluşturmada yeni bir yaklaşım
Öz: Histopatolojik Pap-Smear görsellerinin bilgisayarlı tanı/tespit yazılımlarıyla değerlendirilerek rahim ağzı kanser varlığının otomatik tespit edilmesi konusu aktif bir çalışma alanıdır. Bunun nedeni görsellerde bulunan nesnelerin (hücre çekirdeği, sitoplazma, beyaz kan hücresi, bacil ve benek) üstüste gelerek birbirinin geometrik yapısını ve desenini değiştirmesi, farklı yoğunlukta görüntüye dağılmaları, ve gürültü modellerinin farklı olmalarıdır. Ayrıca etiketlenmiş büyük veri kümesi oluşturmadaki zorluk ve maliyetler bu alanda ortak bir veri kümesinin ortaya çıkmasını engellemiştir. Bahsedilen zorluklar mevcut sınıflandırma çalışmalarındaki başarımları olumsuz etkilemekte ve yeni yaklaşımlara olan ihtiyacı tetiklemektedir. Bu çalışmada, Çekişmeli Üretken Ağlar (ÇÜA) kullanılarak büyük Pap-Smear veri kümeleri inşa etmeye dayalı üç aşamalı bir yaklaşım önerilmektedir. Buna göre ilk aşamada, Pap-Smear görsellerindeki her bir nesne yapısının geometrik şekil ve desen modelleri oluşturulmaktadır. İkinci aşamada, üretilen parametrik modeller kullanılarak istenilen sayıda ve dağılımda nesneye sahip sentetik Pap-Smear görselleri (Ground True) üretilmektedir. Üçüncü aşamada, mevcut ÇÜA’ların (Pix2Pix, CycleGAN, DiscoGAN ve AttentionGAN) GT’yi üretme performansları değerlendirilmekte ve çözüm odaklı yeni bir güncel ÇÜA mimarisi (Pix2PixSSIM) önerilmektedir. Yapılan deneysel çalışmalar, önerilen ÇÜA mimarisiyle oldukça kısa bir sürede büyük Pap-Smear veri kümesinin üretilebildiğini ortaya koymaktadır. Bu sayede yüksek sınıflandırma başarısına sahip derin ağların eğitilebileceği görülmektedir.
Anahtar Kelime: A new approach for Pap-Smear image generation with generative adversarial networks
Öz: The subject of automatic detection of the presence of cervical cancer by evaluating histopathological PapSmear images with computerized diagnosis / detection software is an active field of study. The reason for this is that the objects (cell nucleus, cytoplasm, white blood cell, bacilli and speckle) in the visuals overlap and change the geometric structure and pattern of each other, they are dispersed in the image with different density, and the noise patterns are different. In addition, the difficulty and costs of creating a tagged large dataset prevented the emergence of a common dataset in this area. The mentioned difficulties negatively affect the achievements in current classification studies and trigger the need for new approaches. In this paper, a three-step approach based on building large Pap-Smear datasets using Generative Adversarial Networks (GANs) is proposed. Accordingly, in the first step, geometric shape and pattern models of each object structure in Pap-Smear images are created. In the second stage, synthetic Pap-Smear images (Ground True) with the desired number and distribution of objects are produced using the produced parametric models. In the third stage, the performances of existing GANs (Pix2Pix, CycleGAN, DiscoGAN and AttentionGAN) to produce GT are evaluated and a solution-oriented new current GAN architecture (Pix2PixSSIM) is proposed. Experimental studies show that a large Pap-Smear data set can be produced in a very short time with the proposed GAN architecture. In this way, it is seen that deep networks with high classification success can be trained.
Anahtar Kelime: Belge Türü: Makale Makale Türü: Araştırma Makalesi Erişim Türü: Erişime Açık
- 1. Hanbay K., Hyperspectral image classification using convolutional neural network and twodimensional complex Gabor transform, Journal of the Faculty of Engineering and Architecture of Gazi University, 35 (1), 443-456, 2020.
- 2. Goodfellow, I., Pouget-Abadie, J., Mirza, M., Xu, B., Warde-Farley, D., Ozair, S., & Bengio, Y., Generative adversarial nets, Advances in neural information processing systems, 27, 2014.
- 3. Turhan C.G., Bilge H. Ş., Scalable image generation and super resolution using generative adversarial networks, Journal of the Faculty of Engineering and Architecture of Gazi University, 35 (2), 953-966, 2020.
- 4. Isola, P., Zhu, J. Y., Zhou, T., & Efros, A. A., Image-toimage translation with conditional adversarial networks, In Proceedings of the IEEE conference on computer vision and pattern recognition, 1125-1134, 2017.
- 5. Wang, T. C., Liu, M. Y., Zhu, J. Y., Tao, A., Kautz, J., & Catanzaro, B., High-resolution image synthesis and semantic manipulation with conditional gans. In Proceedings of the IEEE conference on computer vision and pattern recognition, 8798-8807, 2018.
- 6. Choi, Y., Choi, M., Kim, M., Ha, J. W., Kim, S., & Choo, J., Stargan: Unified generative adversarial networks for multi-domain image-to-image translation, In Proceedings of the IEEE conference on computer vision and pattern recognition, 8789-8797, 2018.
- 7. Azadi, S., Tschannen, M., Tzeng, E., Gelly, S., Darrell, T., & Lucic, M., Semantic bottleneck scene generation, arXiv preprint arXiv:1911.11357, 2019.
- 8. Zhu, J. Y., Zhang, R., Pathak, D., Darrell, T., Efros, A. A., Wang, O., & Shechtman, E., Toward multimodal image-to-image translation. arXiv preprint arXiv:1711.11586, 2017
- 9. Xiong, F., Wang, Q., & Gao, Q., Consistent embedded GAN for image-to-image translation. IEEE Access, 7, 126651-126661, 2019.
- 10. Zhu, J. Y., Park, T., Isola, P., & Efros, A. A., Unpaired image-to-image translation using cycle-consistent adversarial networks, In Proceedings of the IEEE international conference on computer vision, 2223- 2232, 2017.
- 11. Kim, T., Cha, M., Kim, H., Lee, J. K., & Kim, J. Learning to discover cross-domain relations with generative adversarial networks, In International Conference on Machine Learning, 1857-1865, PMLR, July 2017.
- 12. Mejjati, Y. A., Richardt, C., Tompkin, J., Cosker, D., & Kim, K. I., Unsupervised attention-guided image to image translation. arXiv preprint arXiv:1806.02311, 2018.
- 13. Liu, M. Y., Breuel, T., & Kautz, J., Unsupervised imageto-image translation networks, arXiv preprint arXiv:1703.00848, 2017.
- 14. Zhou, Y. F., Jiang, R. H., Wu, X., He, J. Y., Weng, S., & Peng, Q., Branchgan: Unsupervised mutual image-toimage transfer with a single encoder and dual decoders, IEEE Transactions on Multimedia, 21 (12), 3136-3149, 2019
- 15. Huang, X., Liu, M. Y., Belongie, S., & Kautz, J., Multimodal unsupervised image-to-image translation. In Proceedings of the European conference on computer vision (ECCV), 172-189, 2018.
- 16. Lin, J., Chen, Z., Xia, Y., Liu, S., Qin, T., & Luo, J., Exploring explicit domain supervision for latent space disentanglement in unpaired image-to-image translation, IEEE transactions on pattern analysis and machine intelligence, 2019.
- 17. Yurt, M., Dar, S. U., Erdem, A., Erdem, E., Oguz, K. K., & Çukur, T., Mustgan: Multi-stream generative adversarial networks for MR image synthesis. Medical Image Analysis, 70, 101944., 2021.
- 18. Beers, A., Brown, J., Chang, K., Campbell, J. P., Ostmo, S., Chiang, M. F., & Kalpathy-Cramer, J., Highresolution medical image synthesis using progressively grown generative adversarial networks. arXiv preprint arXiv:1805.03144, 2018.
- 19. Yao, S., Tan, J., Chen, Y., & Gu, Y., A weighted feature transfer gan for medical image synthesis. Machine Vision and Applications, 32 (1), 1-11, 2021.
- 20. Kaya, H., Cavusoglu, A., Çakmak, H. B., Sen, B., & Delen, D., Supporting the diagnosis process and processes after treatment by using image segmentation and image simulation techniques: Keratoconus example, Journal of the Faculty of Engineering and Architecture of Gazi University, 31 (3), 0-0, 2016.
- 21. Kasım, Ö., & Kuzucuoğlu, A., Detection and Classification of Leukocyte Cells From Smear Image, Journal of the Faculty of Engineering and Architecture of Gazi University, 30 (1),95-109, 2015.
- 22. Shakuntala C., Cervical cancer preventable, treatable, but continues to kill women, Medknow Publications, 1, 2016.
- 23. Mustafa, W. A., Halim, A., Jamlos, M. A., & Idrus, S. Z. S., A Review: Pap Smear Analysis Based on Image Processing Approach, In Journal of Physics: Conference Series, 1529 (2), 022080, IOP Publishing, April, 2020.
- 24. Fekri-Ershad, S., Pap smear classification using combination of global significant value, texture statistical features and time series features, Multimedia Tools and Applications, 78 (22), 31121-31136, 2019.
- 25. Gautam, S., Jith, N., Sao, A. K., Bhavsar, A., & Natarajan, A., Considerations for a PAP smear image analysis system with CNN features, arXiv preprint arXiv:1806.09025, 2018.
- 26. Malm, P., Brun, A., & Bengtsson, E., Simulation of bright‐field microscopy images depicting pap‐smear specimen. Cytometry Part A, 87 (3), 212-226, 2015.
- 27. Sato, M., Hotta, K., Imanishi, A., Matsuda, M., & Terai, K., Segmentation of Cell Membrane and Nucleus by Improving Pix2pix., In BIOSIGNALS, 216-220, January, 2018.
APA | ALTUN GÜVEN S, Talu M (2022). Çekişmeli üretken ağlarla Pap-Smear görüntüsü oluşturmada yeni bir yaklaşım. , 1401 - 1410. 10.17341/gazimmfd.939092 |
Chicago | ALTUN GÜVEN SARA,Talu Muhammed Çekişmeli üretken ağlarla Pap-Smear görüntüsü oluşturmada yeni bir yaklaşım. (2022): 1401 - 1410. 10.17341/gazimmfd.939092 |
MLA | ALTUN GÜVEN SARA,Talu Muhammed Çekişmeli üretken ağlarla Pap-Smear görüntüsü oluşturmada yeni bir yaklaşım. , 2022, ss.1401 - 1410. 10.17341/gazimmfd.939092 |
AMA | ALTUN GÜVEN S,Talu M Çekişmeli üretken ağlarla Pap-Smear görüntüsü oluşturmada yeni bir yaklaşım. . 2022; 1401 - 1410. 10.17341/gazimmfd.939092 |
Vancouver | ALTUN GÜVEN S,Talu M Çekişmeli üretken ağlarla Pap-Smear görüntüsü oluşturmada yeni bir yaklaşım. . 2022; 1401 - 1410. 10.17341/gazimmfd.939092 |
IEEE | ALTUN GÜVEN S,Talu M "Çekişmeli üretken ağlarla Pap-Smear görüntüsü oluşturmada yeni bir yaklaşım." , ss.1401 - 1410, 2022. 10.17341/gazimmfd.939092 |
ISNAD | ALTUN GÜVEN, SARA - Talu, Muhammed. "Çekişmeli üretken ağlarla Pap-Smear görüntüsü oluşturmada yeni bir yaklaşım". (2022), 1401-1410. https://doi.org/10.17341/gazimmfd.939092 |
APA | ALTUN GÜVEN S, Talu M (2022). Çekişmeli üretken ağlarla Pap-Smear görüntüsü oluşturmada yeni bir yaklaşım. Gazi Üniversitesi Mühendislik Mimarlık Fakültesi Dergisi, 37(3), 1401 - 1410. 10.17341/gazimmfd.939092 |
Chicago | ALTUN GÜVEN SARA,Talu Muhammed Çekişmeli üretken ağlarla Pap-Smear görüntüsü oluşturmada yeni bir yaklaşım. Gazi Üniversitesi Mühendislik Mimarlık Fakültesi Dergisi 37, no.3 (2022): 1401 - 1410. 10.17341/gazimmfd.939092 |
MLA | ALTUN GÜVEN SARA,Talu Muhammed Çekişmeli üretken ağlarla Pap-Smear görüntüsü oluşturmada yeni bir yaklaşım. Gazi Üniversitesi Mühendislik Mimarlık Fakültesi Dergisi, vol.37, no.3, 2022, ss.1401 - 1410. 10.17341/gazimmfd.939092 |
AMA | ALTUN GÜVEN S,Talu M Çekişmeli üretken ağlarla Pap-Smear görüntüsü oluşturmada yeni bir yaklaşım. Gazi Üniversitesi Mühendislik Mimarlık Fakültesi Dergisi. 2022; 37(3): 1401 - 1410. 10.17341/gazimmfd.939092 |
Vancouver | ALTUN GÜVEN S,Talu M Çekişmeli üretken ağlarla Pap-Smear görüntüsü oluşturmada yeni bir yaklaşım. Gazi Üniversitesi Mühendislik Mimarlık Fakültesi Dergisi. 2022; 37(3): 1401 - 1410. 10.17341/gazimmfd.939092 |
IEEE | ALTUN GÜVEN S,Talu M "Çekişmeli üretken ağlarla Pap-Smear görüntüsü oluşturmada yeni bir yaklaşım." Gazi Üniversitesi Mühendislik Mimarlık Fakültesi Dergisi, 37, ss.1401 - 1410, 2022. 10.17341/gazimmfd.939092 |
ISNAD | ALTUN GÜVEN, SARA - Talu, Muhammed. "Çekişmeli üretken ağlarla Pap-Smear görüntüsü oluşturmada yeni bir yaklaşım". Gazi Üniversitesi Mühendislik Mimarlık Fakültesi Dergisi 37/3 (2022), 1401-1410. https://doi.org/10.17341/gazimmfd.939092 |