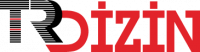
COMPARISON OF CLASSIFICATION PERFORMANCE OF MACHINE LEARNING METHODS IN PREDICTION FINANCIAL FAILURE: EVIDENCE FROM BORSA İSTANBUL
Yıl: 2021 Cilt: 14 Sayı: 1 Sayfa Aralığı: 56 - 86 Metin Dili: İngilizce DOI: 10.17218/hititsbd.880658 İndeks Tarihi: 29-07-2022
COMPARISON OF CLASSIFICATION PERFORMANCE OF MACHINE LEARNING METHODS IN PREDICTION FINANCIAL FAILURE: EVIDENCE FROM BORSA İSTANBUL
Öz: This study aimed to predict the 1 to 2 year future time of the financial failure of 86 manufacturing companies that are operating in Borsa İstanbul. The data comprised of 2010-2012 period, and it depends on 8 quantitative financial variables. Beside 6 variables come from non financial statements. In the study, Artificial Neural Network (NN), Classification and Regression Trees (CART), Support Vector Machine (SVM) and k-Nearest Neighbors (KNN) were used to compare classification performances of related methods. ROC Curve was used to compare the classification performance of the methods. As a result of the analyseis, the overall classification accuracy from the highest to the lowest was SVM (92,31%), CART (88,46%), ANN (84,62%) and KNN (80,77%) 2 years before the financial failure. The overall classification accuracy from the highest to the lowest was CART (96,15%), ANN (92,31%), SVM (80,77%) and KNN (84,62%) 1 year before the financial failure. Return on Equity (ROE) and Return on Assets Ratio (ROA) were found as important variables in the creation of the CART decision tree. The fact that the four models obtained in thise study predicted financial success/failure at a higher rate, and it shows that the models obtained in this study can be included in the models used by relevant people.
Anahtar Kelime: FİNANSAL BAŞARISIZLIK TAHMİNİNDE MAKİNE ÖĞRENMESİ YÖNTEMLERİNİN SINIFLANDIRMA PERFORMANSININ KARŞILAŞTIRILMASI: BORSA İSTANBUL ÖRNEĞİ
Öz: Bu çalışmada Borsa İstanbul İmalat Sanayi Sektörüne kayıtlı 86 firmanın, 2010-2012 dönemine ait verileri kullanılarak 1 ve 2 yıl öncesinden finansal başarısızlık tahmini yapılmıştır. Araştırmada 8 mali tablolara dayalı nicel ve 6 mali tablolara dayalı olmayan değişken kullanılmıştır. Çalışma amacına yönelik analizlerde Yapay Sinir Ağları (ANN), Sınıflandırma ve Regresyon Ağaçları (CART), Destek Vektör Makineleri (SVM) ve K-En Yakın Komşular Algoritması (KNN) yöntemlerinin tahmin performansları yöntemlerin ayırt edici özellikleri altında karşılaştırılmıştır. ROC Eğrisi yöntemlerin sınıflandırma performanslarını karşılaştırmak için kullanılmıştır. Analiz sonucunda, finansal başarısızlıktan iki yıl önce en yüksekten düşüğe genel sınıflandırma doğruluğu SVM (% 92,31), CART (%88,46), ANN (% 84,62), KNN (%80,77) olarak bulunmuştur. Finansal başarısızlıktan bir yıl önce en yüksekten en düşüğe genel sınıflandırma doğruluğu CART (% 96,15), ANN (%92,31), SVM (% 80,77) ve KNN (%84,62) olarak elde edilmiştir. CART karar ağacının oluşturulmasında önemli değişkenler olarak Özsermaye kârlılığı (ROE) ve Aktif Kârlılık Oranı (ROA) bulunmuştur. Çalışmada elde edilen dört modelin finansal başarı/başarısızlığı bir ve iki yıl öncesinden yüksek oranda tahmin etmesi, ilgililerin kullandıkları modeller içerisine bu çalışmada elde edilen modelleri dâhil edebileceklerini göstermektedir.
Anahtar Kelime: Belge Türü: Makale Makale Türü: Araştırma Makalesi Erişim Türü: Erişime Açık
- Akay, E. Ç. (2018). A new horizon in econometrics: big data and machine learning. Social Sciences Research Journal, 7(2), 41-53. Retrieved from: https://dergipark.org.tr/ tr/pub/ssrj/issue/37241/423147
- Akkaya, G. C., Demireli, E., and Yakut, Ü. H. (2009). Using artificial neural networks in financial failure prediction: an application in Borsa Istanbul. Journal of Social Sciences, Eskişehir Osmangazi University, 10(2), 187-216. Retrieved from: https://dergipark.org.tr/tr/pub/ogusbd/issue/10996/131592
- Akpınar, H. (2014). Data mining data analysis. İstanbul: Papatya Publications.
- Alfaro, E., Garcia, M. G. N., and Elizondo, D. (2008). Bankruptcy forecasting: an empirical comparison of adaboost and neural networks. Decision Support Systems, (45), 110-122. doi: 10.1016/j.dss.2007.12.002
- Bilir, H. (2015). Definition and market oriented solution of financial distress: debt structuring, asset sales and new capital injection. Sosyoekonomi, 1, 9-24. Retrieved from: https://dergipark.org.tr/tr/download/article-file/197806
- Chandra, D. K., Ravi, V., and Bose, I. (2009). Failure prediction of DOTCOM companies using hybrid intelligent techniques. Expert Systems with Applications, 36, 4830-4837. doi: 10.1016/j.eswa.2008.05.047
- Chen, Mu-Y. (2011). Predicting corporate financial distress based on integration of decision tree classification and logistic regression. Expert Systems with Applications, 38, 11261-11272. doi: 10.1016/j.eswa.2011.02.173
- Chen, M.-Y. (2011). Bankruptcy prediction in firms with statistical and intelligent techniques and a comparison of evolutionary computation approaches. Computers and Mathematics with Applications, 62(12), 4514-4524. doi: 10.1016/j.camwa.2011.10.030
- Chuang, C.-L. (2013). Application of hybrid case-based reasoning for enhanced performance in bankruptcy prediction. Information Sciences, (236), 174-185. doi: 10.1016/j.ins.2013.02.015
- Çelik U., Akçetin, E., and Gök, M. (2017). Data mining with Rapidminer, İstanbul: Pusula Publications.
- Çöllü, D. A., Akgün, L., and Eyduran, E. (2020). Financial failure prediction with decision tree algorithms: textile, wearing apparel and leather sector application. International Journal of Economics and Innovation, 6(2), 225-246. doi: 10.20979/ueyd.698738
- Dener, M., Dörterler, M., and Orman, A. (2009). Conference: XI. Academic Informatics Conference, Open Source Data Mining Programs: Sample Application in Weka. (pp. 1-11). Şanlıurfa
- Divsalar, M., Firouzabadi, A. K., Sadeghi, M., Behrooz, A. H., and Alavi, A. H. (2011). Towards the prediction of business failure via computational intelligence techniques. Expert Systems, 28(3), 209-226. doi: 10.1111/j.1468-0394.2011.00580.x
- Enke, D., and Thawornwong, S. (2005). The use of data mining and neural networks for forecasting stock market returns. Expert Systems with Applications, (29), 927-940. doi: 10.1016/j.eswa.2005.06.024
- Elmas, Ç. (2018). Artificial intelligence applications. Ankara: Seçkin Publishing.
- Etemadi, H., Rostamy, A., A., A., and Dehkordi, H. F. (2009). A genetic programming model for bankruptcy prediction: Empirical evidence from Iran. Expert Systems with Applications, (36), 3199–3207. doi: 10.1016/j.eswa.2008.01.012
- Gaganis, C. (2009). Classification techniques for the identification of falsified financial statements: A comparative analysis. Intelligent Systems in Accounting, Finance and Management, (16), 207-229. doi: 10.1002/isaf.303
- Geng, R., Bose, I., and Chen, X. (2015). Prediction of financial distress: an empirical study of listed Chinese companies using data mining. European Journal of Operational Research, (241), 236-247. doi: 10.1016/j.ejor.2014.08.016
- Gepp, A., Kumar, K., and Bhattacharya, S. (2010). Business failure prediction using decision trees. Journal of Forecasting, (29), 536-555. doi: 10.1002/for.1153
- Gepp, A., and Kumar, K. (2015). Predicting financial distress: A comparison of survival analysis and decision tree techniques. Procedia Computer Science, (54), 396-404. doi: 10.1016/j.procs.2015.06.046
- Huang, Z., Chen, H., Hsu, C-J., Chen, W.-H., and Wu, S. (2004). Credit rating analysis with support vector machines and neural networks: A market comparative study. Decision Support Systems, (37), 543-558. doi: 10.1016/S0167-9236(03)00086-1
- Jardin, P. D. (2010). Predicting bankruptcy using neural networks and other classification methods: the influence of variable selection techniques on model accuracy. Neurocomputing, (73), 2047-2060. doi: 10.1016/j.neucom.2009.11.034
- Jardin, P. D. (2012). The inflcuence of variable selection methods on the accuracy of bankruptcy prediction models. Bankers, Markets and Investors, (116), 20-39. Retrieved from: https:www.researchgate.net/publication/235643793
- Jardin, P. D., and Séverin, E. (2011). Predicting corporate bankruptcy using a self-organizing map: An empirical study to improve the forecasting horizon of a financial failure model. Decision Support Systems, (51), 701-711. doi: 10.1016/j.dss.2011.04.001
- Jardin, P. D. (2016). A two-stage classification technique for bankruptcy prediction. European Journal of Operational Research, (254), 236-252. doi: 10.1016/j.ejor.2016.03.008
- Kara, Y., Boyacıoğlu, M. A., and Baykan, Ö. K. (2011). Predicting direction of stock price index movement using artificial neural networks and support vector machines: the sample of the İstanbul Stock Exchange. Expert Systems with Applications, (38), 5311-5319. doi: 10.1016/j.eswa.2010.10.027
- Kaygın, C. Y., Tazegül, A., and Yazarkan, H. (2016). Estimation capability of financial failures and successes of enterprises using data mining and logistic regression analysis. Ege Academic Review, 16(1), 147-159. doi: 10.21121/eab.2016116590
- Keasey, K., and Watson, R. (1987). Non-financial symptoms and the prediction of small company failure: a test of Arcenti’s Hypotheses. Journal of Business Finance and Accounting, 14(3), 335-354. doi: 10.1111/J.1468-5957.1987.TB00099.X
- Kılıç, S., (2015). Kappa test. Journal of Mood Disorders, 5(3), 142-144. doi: 10.5455/jmood.20150920115439
- Koç, S., and Ulucan S. (2016). Testing of Altman Z methods which is used for detecting of financial failures with fuzzy logic (Anfis) technique: a case study on technology and textile sector. Journal of Finance Letters, (106), 147-167. doi: 10.33203/mfy.341768
- Le, H. H., and Viviani, J.-L. (2018). Predicting bank failure: An improvement by implementing a machine-learning approach to classical financial ratios. Research in International Business and Finance, (44), 16-25. doi: 10.1016/j.ribaf.2017.07.104
- Li, H., and Sun, J. (2009). Predicting business failure using multiple case-based reasoning combined with support vector machine. Expert Systems with Applications, (36), 10085– 10096. doi: 10.1016/j.eswa.2009.01.013
- Li, H., Sun, J., and Wu, J. (2010). Predicting business failure using classification and regression tree: an empirical comparison with popular classical statistical methods and top classification mining methods. Expert Systems with Applications, (37), 5895-5904. doi: 10.1016/j.eswa.2010.02.016
- Li, H., and Sun, J. (2013). Predicting business failure using an rsf-based case-based reasoning ensemble forecasting method. Journal of Forecasting, (32), 180-192. doi: 10.1002/for.1265
- Li, A., and Wu J. and Zhidong L. (2017). Market manipulation detection based on classification methods. Procedia Computer Science, (122), 788-795. doi: 10.1016/j.procs.2017.11.438
- Liang, D., Tsaı, C.-F., and Wu, H.-T. (2015). The effect of feature selection on financial distress prediction. Knowledge-Based Systems, (73), 289-297. doi: 10.1016/j.knosys.2014. 10.010
- Lin, F., Liang, D., Yeh, C.-C., and Huang, J.-C. (2014). Novel feature selection methods to financial distress prediction. Expert Systems with Applications, (41), 2472–2483. doi: 10.1016/j.eswa.2013.09.047
- Min, J. H., and Lee, Y.-C. (2005). Bankruptcy prediction using support vector machine with optimal choice of kernel function parameters. Expert Systems with Applications, (28), 603–614. doi: 10.1016/j.eswa.2004.12.008
- Muller, G.H, Steyn-Bruwer, B. W., and Hamman, W.D. (2009). Predicting financial distress of companies listed on the JSE- a comparison of techniques. S. Afr.Bus. Manage, 40(1), 21-32. doi: 10.4102/sajbm.v40i1.532
- Özçalıcı, M. (2017). Stock price prediction with extreme learning machines. Hacettepe University Journal of Economics and Administrative Sciences, 35(1), 67-88. doi: 10.17065/huniibf.303305
- Özdağoğlu, G., Özdağoğlu, A., Gümüş, Y., and Gümüş, G. K. (2017). The application of data mining techniques in manipulated financial statement classification: The case of Turkey. Journal of AI and Data Mining, 5(1), 67-77. doi: 10.22044/jadm.2016.664
- Özkan, Y. (2016). Data mining methods. İstanbul: Papatya Publications.
- Öztemel, E. (2012). Artificial neural networks, İstanbul: Papatya Publications.
- Sayılgan, G., and Ece, A. (2016). Bankruptcy postponement and panoramic analysis on bankruptcy postponement litigations in Turkey between 2009-2013. Revenue and Finance Articles, (105), 47-74. doi: 10.33203/mfy.312132
- Selimoğlu, S., and Orhan, A. (2015). Measuring business failure by using ratio analysis and discriminant analysis: a research on textile, clothes and leather firms listed in the İstanbul Stock Exchange. Journal of Accounting and Finance, April, 21-40. doi: 10.25095/mufad.396529
- Shin, K.-S., Lee, T. S., and Kim, H.-j. (2005). An application of support vector machines in bankruptcy prediction model. Expert Systems with Applications, (28), 127-135. doi: 10.1016/j.eswa.2004.08.009
- Silahtaroğlu, G. (2016). Data mining concepts and algorithms. İstanbul: Papatya Publications.
- Sun, J., Li, H., Huang, Q.-H., and He, K.-Y. (2014). Predicting financial distress and corporate failure: a review from the state-of-the-art definitions, modeling, sampling, and featuring approaches. Knowledge-Based Systems, (57), 41-56. doi: 10.1016/j.knosys.2013. 12.006
- Tsai, C. F., Hsu, Y. F., and Yen D. C. (2014). A comparative study of classifier ensembles for bankruptcy prediction. Applied Soft Computing, (24), 977-984. doi: 10.1016/j.asoc.2014.08.047
- Wu, W., Lee, V. C. S., and Tan, T. Y. (2006). Data preprocessing and data parsimony in corporate failure forecast models: Evidence from Australian materials industry. Accounting and Finance, (46), 327-345. doi: 10.1111/j.1467-629X.2006.00170.x
- Yakut, E. (2012). The Comparison of the classification successes of the artifical neural networks through data mining techniques of c5.0 algorithm and supporting vector machines: an application in manufacturing sector. (Unpublished PhD Thesis). Atatürk University Social Sciences Institute, Erzurum.
- Yürük, M. F., and Ekşi, İ. H. (2019). Financial failure prediction of companies using artificial intelligencė methods: An application in Bist manufacturing sector. Mukaddime, 10(1), 393-422. doi: 10.19059/mukaddime.533151
APA | AKSOY B, BOZTOSUN D (2021). COMPARISON OF CLASSIFICATION PERFORMANCE OF MACHINE LEARNING METHODS IN PREDICTION FINANCIAL FAILURE: EVIDENCE FROM BORSA İSTANBUL. , 56 - 86. 10.17218/hititsbd.880658 |
Chicago | AKSOY BARIS,BOZTOSUN Derviş COMPARISON OF CLASSIFICATION PERFORMANCE OF MACHINE LEARNING METHODS IN PREDICTION FINANCIAL FAILURE: EVIDENCE FROM BORSA İSTANBUL. (2021): 56 - 86. 10.17218/hititsbd.880658 |
MLA | AKSOY BARIS,BOZTOSUN Derviş COMPARISON OF CLASSIFICATION PERFORMANCE OF MACHINE LEARNING METHODS IN PREDICTION FINANCIAL FAILURE: EVIDENCE FROM BORSA İSTANBUL. , 2021, ss.56 - 86. 10.17218/hititsbd.880658 |
AMA | AKSOY B,BOZTOSUN D COMPARISON OF CLASSIFICATION PERFORMANCE OF MACHINE LEARNING METHODS IN PREDICTION FINANCIAL FAILURE: EVIDENCE FROM BORSA İSTANBUL. . 2021; 56 - 86. 10.17218/hititsbd.880658 |
Vancouver | AKSOY B,BOZTOSUN D COMPARISON OF CLASSIFICATION PERFORMANCE OF MACHINE LEARNING METHODS IN PREDICTION FINANCIAL FAILURE: EVIDENCE FROM BORSA İSTANBUL. . 2021; 56 - 86. 10.17218/hititsbd.880658 |
IEEE | AKSOY B,BOZTOSUN D "COMPARISON OF CLASSIFICATION PERFORMANCE OF MACHINE LEARNING METHODS IN PREDICTION FINANCIAL FAILURE: EVIDENCE FROM BORSA İSTANBUL." , ss.56 - 86, 2021. 10.17218/hititsbd.880658 |
ISNAD | AKSOY, BARIS - BOZTOSUN, Derviş. "COMPARISON OF CLASSIFICATION PERFORMANCE OF MACHINE LEARNING METHODS IN PREDICTION FINANCIAL FAILURE: EVIDENCE FROM BORSA İSTANBUL". (2021), 56-86. https://doi.org/10.17218/hititsbd.880658 |
APA | AKSOY B, BOZTOSUN D (2021). COMPARISON OF CLASSIFICATION PERFORMANCE OF MACHINE LEARNING METHODS IN PREDICTION FINANCIAL FAILURE: EVIDENCE FROM BORSA İSTANBUL. Hitit Sosyal Bilimler Dergisi (Online), 14(1), 56 - 86. 10.17218/hititsbd.880658 |
Chicago | AKSOY BARIS,BOZTOSUN Derviş COMPARISON OF CLASSIFICATION PERFORMANCE OF MACHINE LEARNING METHODS IN PREDICTION FINANCIAL FAILURE: EVIDENCE FROM BORSA İSTANBUL. Hitit Sosyal Bilimler Dergisi (Online) 14, no.1 (2021): 56 - 86. 10.17218/hititsbd.880658 |
MLA | AKSOY BARIS,BOZTOSUN Derviş COMPARISON OF CLASSIFICATION PERFORMANCE OF MACHINE LEARNING METHODS IN PREDICTION FINANCIAL FAILURE: EVIDENCE FROM BORSA İSTANBUL. Hitit Sosyal Bilimler Dergisi (Online), vol.14, no.1, 2021, ss.56 - 86. 10.17218/hititsbd.880658 |
AMA | AKSOY B,BOZTOSUN D COMPARISON OF CLASSIFICATION PERFORMANCE OF MACHINE LEARNING METHODS IN PREDICTION FINANCIAL FAILURE: EVIDENCE FROM BORSA İSTANBUL. Hitit Sosyal Bilimler Dergisi (Online). 2021; 14(1): 56 - 86. 10.17218/hititsbd.880658 |
Vancouver | AKSOY B,BOZTOSUN D COMPARISON OF CLASSIFICATION PERFORMANCE OF MACHINE LEARNING METHODS IN PREDICTION FINANCIAL FAILURE: EVIDENCE FROM BORSA İSTANBUL. Hitit Sosyal Bilimler Dergisi (Online). 2021; 14(1): 56 - 86. 10.17218/hititsbd.880658 |
IEEE | AKSOY B,BOZTOSUN D "COMPARISON OF CLASSIFICATION PERFORMANCE OF MACHINE LEARNING METHODS IN PREDICTION FINANCIAL FAILURE: EVIDENCE FROM BORSA İSTANBUL." Hitit Sosyal Bilimler Dergisi (Online), 14, ss.56 - 86, 2021. 10.17218/hititsbd.880658 |
ISNAD | AKSOY, BARIS - BOZTOSUN, Derviş. "COMPARISON OF CLASSIFICATION PERFORMANCE OF MACHINE LEARNING METHODS IN PREDICTION FINANCIAL FAILURE: EVIDENCE FROM BORSA İSTANBUL". Hitit Sosyal Bilimler Dergisi (Online) 14/1 (2021), 56-86. https://doi.org/10.17218/hititsbd.880658 |